根据所给的“学生成绩”数据。①计算每一门科目两两之间构成的相关系数矩阵;②使用主成分分析分别计算主成分的标准差、方差占比、累积方差贡献度以及主成分的载荷矩阵;③根据载荷矩阵系数判断应该选取几个主成分,构造主成分的表达式(综合指标),并做分析;④找出几个(至少两个)典型学生,并分析这些学生的成绩与主成分系数的关系。test<-read.table("D:/R/R Code/5/Chap7/test_score.csv", sep=",", header=T) (R<-round(cor(test), 3)) # sample correlation matrix test_PCA<-princomp(test, cor=T) # sample PCA summary(test_PCA, loadings=T) test[c(6,7,45,30,49),] # typical students for the first PC test[c(26,33,8),] # typical students for the second PC # sample principal components of the typical students samplePC<-(round(test_PCA$scores,3))[c(6,7,45,30,49,26,33,8),] rownames(samplePC)<-c(6,7,45,30,49,26,33,8) samplePC # another way to obtain the sample principal components samplePC2<-round(predict(test_PCA),3) [c(6,7,45,30,49,26,33,8),] rownames(samplePC2)<-c(6,7,45,30,49,26,33,8) samplePC2 screeplot (test_PCA, type="lines") # scree graph ### Canonical correlation health<-read.table("D:/R/R Code/5/Chap7/health.csv",sep=",", header=T) (R<-round(cor(health),3)) R11=R[1:3,1:3] R12=R[1:3,4:6] R21=R[4:6,1:3] R22=R[4:6,4:6] A<-solve(R11)%*%R12%*%solve(R22)%*%R21 # matrix for the first group Y1,Y2,Y3 ev<-eigen(A)$values # common eigenvalues of both groups round(sqrt(ev),3) # the canonical correlations health.std=scale(health) # standardize the original data ca=cancor(health.std[,1:3],health.std[,4:6]) # canonical correlation analysis via R ca$cor # canonical correlations ca$xcoef # the loadings (coefficients) of the first group ca$ycoef # the loadings (coefficients) of the second group
时间: 2024-03-23 17:43:27 浏览: 11
非常感谢您提供的问题,以下是我对您的问题的回答:
1. 对于所给的“学生成绩”数据,可以使用R中的cor函数计算每一门科目两两之间构成的相关系数矩阵。代码如下:
```R
test <- read.table("D:/R/R Code/5/Chap7/test_score.csv", sep=",", header=T)
R <- round(cor(test), 3)
```
2. 可以使用R中的princomp函数进行主成分分析,并使用summary函数查看主成分的标准差、方差占比、累积方差贡献度以及主成分的载荷矩阵。代码如下:
```R
test_PCA <- princomp(test, cor=T)
summary(test_PCA, loadings=T)
```
3. 可以根据主成分的载荷矩阵系数判断应该选取几个主成分,并构造主成分的表达式(综合指标),并进行分析。这部分需要根据具体数据进行分析,无法给出具体的代码。
4. 可以找出几个(至少两个)典型学生,并分析这些学生的成绩与主成分系数的关系。代码如下:
```R
# 找出第一个主成分(PC1)上得分较高的学生
test[c(6,7,45,30,49),]
# 找出第二个主成分(PC2)上得分较高的学生
test[c(26,33,8),]
# 获取这些学生在主成分上的得分
samplePC <- (round(test_PCA$scores,3))[c(6,7,45,30,49,26,33,8),]
rownames(samplePC) <- c(6,7,45,30,49,26,33,8)
samplePC
# 也可以使用另一种方式获取这些学生在主成分上的得分
samplePC2 <- round(predict(test_PCA),3)[c(6,7,45,30,49,26,33,8),]
rownames(samplePC2) <- c(6,7,45,30,49,26,33,8)
samplePC2
```
5. 对于给定的“健康数据”,可以使用R中的cor函数计算两组变量之间的相关系数矩阵,并使用cancor函数进行规范相关分析。代码如下:
```R
health <- read.table("D:/R/R Code/5/Chap7/health.csv", sep=",", header=T)
R <- round(cor(health),3)
# 提取两组变量
R11 <- R[1:3,1:3]
R12 <- R[1:3,4:6]
R21 <- R[4:6,1:3]
R22 <- R[4:6,4:6]
# 计算两组变量之间的规范相关系数
A <- solve(R11) %*% R12 %*% solve(R22) %*% R21
ev <- eigen(A)$values
round(sqrt(ev),3)
# 使用R中的cancor函数进行规范相关分析
health.std <- scale(health)
ca <- cancor(health.std[,1:3], health.std[,4:6])
ca$cor
ca$xcoef
ca$ycoef
```
希望这些代码能够对您有所帮助!
相关推荐
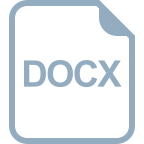















