StratifiedKFold
时间: 2023-11-27 14:04:25 浏览: 46
StratifiedKFold是一种交叉验证的方法,在KFold的基础上加入了分层抽样的思想。它可以保证训练集和测试集的数据分布相同,因此在算法上更加可靠。使用StratifiedKFold时,需要同时输入数据和标签,以便统一训练集和测试集的分布。StratifiedKFold会将数据集分成k等分,然后依次选取其中一份作为测试集,剩下的k-1份作为训练集。
下面是一个示例代码,演示了如何使用StratifiedKFold:
```python
from sklearn.model_selection import StratifiedKFold
import numpy as np
X = np.array([[1,2,3,4], [11,12,13,14], [21,22,23,24], [31,32,33,34], [41,42,43,44], [51,52,53,54], [61,62,63,64], [71,72,73,74]])
y = np.array([1,1,0,0,1,1,0,0])
sfolder = StratifiedKFold(n_splits=4, random_state=0)
for train, test in sfolder.split(X, y):
print('Train: %s | Test: %s' % (train, test))
```
相关问题
python StratifiedKFold
StratifiedKFold是一个用于数据集交叉验证的方法,它可以确保每个折叠(fold)中各个类别的样本比例与整个数据集中的比例相同。这对于不平衡的数据集特别有用。
在Python中,可以使用sklearn.model_selection模块中的StratifiedKFold类来实现。
以下是一个示例代码:
```python
from sklearn.model_selection import StratifiedKFold
# 创建数据集和标签
X = ...
y = ...
# 创建StratifiedKFold对象
kf = StratifiedKFold(n_splits=5, shuffle=True, random_state=42)
# 使用StratifiedKFold进行交叉验证
for train_index, test_index in kf.split(X, y):
X_train, X_test = X[train_index], X[test_index]
y_train, y_test = y[train_index], y[test_index]
# 在训练集上训练模型
model.fit(X_train, y_train)
# 在测试集上评估模型
score = model.score(X_test, y_test)
print("Accuracy:", score)
```
在上述示例中,我们将数据集X和对应的标签y传递给StratifiedKFold的split方法。然后,我们遍历生成的折叠(train_index和test_index),并根据这些索引将数据集划分为训练集和测试集。最后,我们使用训练集来训练模型,并使用测试集评估模型的性能。
stratifiedkfold参数
在 Scikit-learn 中,StratifiedKFold 是一种交叉验证方法,用于将数据集划分为 k 个折叠,其中每个折叠都保留了原始数据集中不同类别的比例。这种方法适用于分类问题,因为它可以确保每个折叠中都包含足够数量的每个类别样本。
StratifiedKFold 的主要参数如下:
- n_splits:表示将数据分成几个折叠,默认值为 5。
- shuffle:表示是否在分割前将数据集打乱,默认为 False。
- random_state:用于控制随机数生成器的种子,以确保每次运行结果一致。默认情况下,它是 None,表示使用默认的随机数生成器。
以下是一个示例:
```python
from sklearn.model_selection import StratifiedKFold
# 创建 StratifiedKFold 对象
skf = StratifiedKFold(n_splits=5, shuffle=True, random_state=42)
# 对数据集进行分割
for train_index, test_index in skf.split(X, y):
X_train, X_test = X[train_index], X[test_index]
y_train, y_test = y[train_index], y[test_index]
# 使用训练集进行模型训练,并在测试集上评估模型性能
```
相关推荐
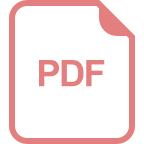
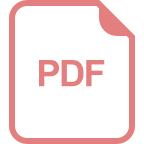
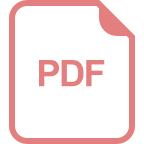













