ghostnet替换unet主干
时间: 2024-06-23 08:02:20 浏览: 8
GhostNet是一种轻量级的卷积神经网络(CNN),由腾讯AI Lab于2019年提出,它的设计目标是提高模型效率的同时保持较高的性能。与经典的U-Net(全卷积网络,特别适用于图像分割任务)相比,GhostNet在主干网络结构上采用了Ghost操作,这是一种通过子特征图插值和并行扩展通道数的方法,减少了参数数量和计算开销。
在U-Net中,通常使用像VGG、ResNet或DenseNet等深度网络作为主干,这些网络结构具有很多层和参数,适合处理复杂的图像特征。而GhostNet的主干则更注重效率,使用较少的计算资源就能达到相似甚至更好的性能,因此在需要高效计算和内存限制的应用场景下,如嵌入式设备上的医疗图像分析,GhostNet可能是U-Net的一个可行替代。
如果你正在考虑在图像分割项目中使用GhostNet替换U-Net的主干,有几个关键点需要考虑:
1. 性能对比:评估 GhostNet 在相同数据集和任务上的表现,看看是否能提供类似或更好的分割准确度。
2. 训练速度:由于 GhostNet 的轻量化设计,训练时间可能会有所缩短。
3. 模型大小:GhostNet 的模型尺寸通常较小,这有利于在资源有限的设备上部署。
4. 调参:可能需要针对GhostNet调整超参数,例如学习率、优化器等,以优化其在特定任务中的性能。
相关问题
UNET++替换主干
引用中提到,UNET可以很容易地作为Mask-RCNN中的主干架构来替换。只需用建议的嵌套密集跳过路径替换普通跳过连接即可。因此,可以将UNET替换为Mask-RCNN的主干架构。<span class="em">1</span><span class="em">2</span><span class="em">3</span>
#### 引用[.reference_title]
- *1* *2* [精读论文UNet++: A Nested U-Net Architecture for Medical Image Segmentation(附翻译)](https://blog.csdn.net/m0_38088084/article/details/107499067)[target="_blank" data-report-click={"spm":"1018.2226.3001.9630","extra":{"utm_source":"vip_chatgpt_common_search_pc_result","utm_medium":"distribute.pc_search_result.none-task-cask-2~all~insert_cask~default-1-null.142^v93^chatsearchT3_2"}}] [.reference_item style="max-width: 50%"]
- *3* [UNet++: A Nested U-Net Architecture for Medical Image Segmentation](https://blog.csdn.net/Acmer_future_victor/article/details/114251630)[target="_blank" data-report-click={"spm":"1018.2226.3001.9630","extra":{"utm_source":"vip_chatgpt_common_search_pc_result","utm_medium":"distribute.pc_search_result.none-task-cask-2~all~insert_cask~default-1-null.142^v93^chatsearchT3_2"}}] [.reference_item style="max-width: 50%"]
[ .reference_list ]
将Unet的主干网络替换成densenet
将UNet的主干网络替换为DenseNet是可行的。UNet是一种常用的语义分割网络,而DenseNet是一种密集连接的卷积网络,具有更好的特征重用能力。下面是一种将UNet的主干网络替换为DenseNet的方法:
1. 导入所需的库和模块:
```python
import torch
import torch.nn as nn
from torchvision.models import densenet121
```
2. 定义一个自定义的UNet模型,并将UNet的主干网络替换为DenseNet:
```python
class UNet(nn.Module):
def __init__(self, in_channels, out_channels):
super(UNet, self).__init__()
# 替换UNet的主干网络为DenseNet
self.densenet = densenet121(pretrained=True)
# 调整DenseNet的输入通道数
self.densenet.features.conv0 = nn.Conv2d(in_channels, 64, kernel_size=7, stride=2, padding=3, bias=False)
# 定义UNet的其他层
self.encoder1 = self.densenet.features.denseblock1
self.encoder2 = self.densenet.features.denseblock2
self.encoder3 = self.densenet.features.denseblock3
self.encoder4 = self.densenet.features.denseblock4
# 定义解码器
self.decoder4 = nn.ConvTranspose2d(1024, 512, kernel_size=3, stride=2, padding=1, output_padding=1, bias=False)
self.decoder3 = nn.ConvTranspose2d(512, 256, kernel_size=3, stride=2, padding=1, output_padding=1, bias=False)
self.decoder2 = nn.ConvTranspose2d(256, 128, kernel_size=3, stride=2, padding=1, output_padding=1, bias=False)
self.decoder1 = nn.ConvTranspose2d(128, 64, kernel_size=3, stride=2, padding=1, output_padding=1, bias=False)
self.upsample = nn.Upsample(scale_factor=2, mode='bilinear', align_corners=True)
self.final_conv = nn.Conv2d(64, out_channels, kernel_size=1)
def forward(self, x):
# 编码器部分
encoder1 = self.encoder1(x)
encoder2 = self.encoder2(encoder1)
encoder3 = self.encoder3(encoder2)
encoder4 = self.encoder4(encoder3)
# 解码器部分
decoder4 = self.decoder4(encoder4)
decoder3 = self.decoder3(decoder4 + encoder3)
decoder2 = self.decoder2(decoder3 + encoder2)
decoder1 = self.decoder1(decoder2 + encoder1)
# 上采样
upsampled = self.upsample(decoder1)
# 输出层
output = self.final_conv(upsampled)
return output
```
在上面的代码中,我们首先导入了所需的库和模块。然后,我们定义了一个自定义的UNet模型,并将UNet的主干网络替换为DenseNet。我们通过加载预训练的DenseNet模型并调整其输入通道数来实现这一点。接下来,我们定义了UNet的其他层,如编码器和解码器。最后,我们定义了模型的前向传播函数。
请注意,上面的代码中使用的是DenseNet121作为示例,你可以根据需要选择不同的DenseNet模型。
希望这可以回答你的问题!如果你还有其他问题,请继续提问。
相关推荐
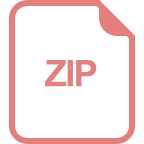
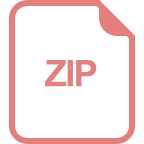












