graph = nx.DiGraph() graph.add_nodes_from(example.dominoModules[i].nodes()) graph.add_edges_from(example.dominoModules[i].edges()) pos = nx.spring_layout(graph) plt.title(f'Bayesian Network Based On {example.dominoModules[i].get_roots()[0]}') nx.draw(graph, pos, with_labels=True, node_color='lightblue', node_size=2000, edge_color='gray', arrows=True) plt.show()这样绘制的贝叶斯网络图形很乱怎么调整
时间: 2024-04-17 14:27:40 浏览: 22
如果使用`nx.spring_layout()`布局绘制的贝叶斯网络图形很乱,可以尝试使用其他布局算法或进行一些调整来改善图形的可读性。下面是一些可能的解决方法:
1. 更换布局算法:`networkx`库提供了多种布局算法可供选择。除了`spring_layout`,还可以尝试使用其他布局算法,如`circular_layout`、`shell_layout`、`kamada_kawai_layout`等。不同的布局算法适用于不同类型的图形,您可以尝试多种算法以找到适合您的贝叶斯网络的最佳布局。
2. 调整节点位置:使用`pos`参数手动调整节点的位置,可以将节点移动到更合适的位置。您可以通过修改`pos`字典中节点的坐标来实现。尝试手动调整根节点和一级节点的位置,以使它们更接近您想要的布局效果。
3. 缩小节点尺寸:节点的尺寸可能会影响整体图形的可读性。尝试减小节点的大小,以便更多节点能够在有限的绘图空间内展示。
4. 调整边的样式:通过调整边的样式,如颜色、线型、箭头等,可以使图形更易于理解。您可以使用`edge_color`、`linewidths`、`arrows`等参数来调整边的样式。
5. 分层绘制:如果贝叶斯网络具有明显的层次结构,您可以尝试按层次绘制节点,以使图形更有结构感。可以使用`networkx`库中的`bipartite_layout`或`multipartite_layout`函数来实现分层绘制。
通过尝试上述方法,您应该能够改善贝叶斯网络图形的可读性。根据贝叶斯网络的特点和数据集的复杂性,可能需要多次调整布局和样式才能达到最佳效果。
相关问题
graph = nx.DiGraph() graph.add_nodes_from(example.dominoModules[i].nodes()) graph.add_edges_from(example.dominoModules[i].edges()) pos = nx.spring_layout(graph) plt.title(f'Bayesian Network Based On {example.dominoModules[i].get_roots()[0]}') nx.draw(graph, pos, with_labels=True, node_color='lightblue', node_size=2000, edge_color='gray', arrows=True) plt.show()改成使用multipartite_layout绘制
要使用`nx.multipartite_layout()`绘制贝叶斯网络图,您需要为每个节点指定层次。由于您没有提供具体的节点层次信息,我将提供一个示例代码,其中假设根节点在最上面,一级节点在根节点的下面,以此类推。请注意,您需要根据实际情况调整节点的层次和样式。
```python
import matplotlib.pyplot as plt
import networkx as nx
from pgmpy.readwrite import XMLBIFReader
# 从XML文件中读取贝叶斯网络
reader = XMLBIFReader("network.xml")
model = reader.get_model()
# 创建一个空的有向图
G = nx.DiGraph()
# 添加节点和层次信息
nodes = model.nodes()
levels = {}
for node in nodes:
level = model.get_independencies(node).level()
levels[node] = level
G.add_node(node, level=level)
# 添加边
G.add_edges_from(model.edges())
# 设置布局
pos = nx.multipartite_layout(G, subset_key="level", align="vertical")
# 绘制图形
nx.draw(G, pos, with_labels=True, node_size=1000, node_color="lightblue", font_size=10)
# 显示图形
plt.axis("off")
plt.show()
```
在这个示例中,我们首先从XML文件中读取贝叶斯网络模型,并创建一个空的有向图。然后,我们为每个节点添加层次信息,并将其作为节点属性存储在图中。接下来,我们添加边,然后使用`nx.multipartite_layout()`函数根据节点的层次信息设置节点的布局,使得根节点在最上面,一级节点在根节点的下面,以此类推。最后,使用`nx.draw()`函数绘制图形,并使用`plt.axis("off")`和`plt.show()`函数显示图形。
请注意,您需要将上述代码中的`"network.xml"`替换为您自己的贝叶斯网络模型的文件名或路径。此外,您可能需要根据实际情况调整节点大小、颜色、字体大小等参数以满足您的需求。
class Graph: graph = nx.DiGraph() def __init__(self): self.graph = nx.DiGraph() def createGraph(self, filename): file = open(filename, 'r') for line in file.readlines(): nodes = line.split() edge = (int(nodes[0]), int(nodes[1])) self.graph.add_edge(*edge) return self.graph 什么意思
这段代码定义了一个Graph类,用于创建和操作DiGraph对象(有向图)。类的初始化方法__init__中创建了一个空的DiGraph对象。类还有一个createGraph方法,用于从文件中读取图的边信息并创建DiGraph对象。方法接收一个文件名作为输入,并返回创建的DiGraph对象。具体实现中,方法打开文件并逐行读取,将每行的两个节点转换为整数,并将它们作为有向边添加到DiGraph对象中。最后,方法返回创建的DiGraph对象。
相关推荐
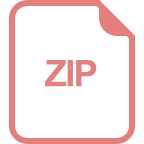












