对pima数据集进行数据预处理
时间: 2023-11-17 18:59:56 浏览: 79
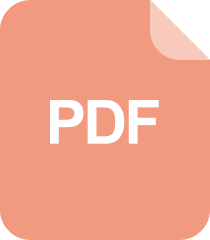
数据预处理实例pima数据预处理.pdf
Pima数据集是一个二分类问题,包含了768个样本,每个样本有8个特征和1个标签。在进行数据预处理之前,需要先导入数据集并查看数据的基本信息。
```python
import pandas as pd
import numpy as np
# 导入数据集
data = pd.read_csv('pima.csv')
# 查看数据基本信息
print(data.info())
```
数据基本信息如下:
```
<class 'pandas.core.frame.DataFrame'>
RangeIndex: 768 entries, 0 to 767
Data columns (total 9 columns):
# Column Non-Null Count Dtype
--- ------ -------------- -----
0 Pregnancies 768 non-null int64
1 Glucose 768 non-null int64
2 BloodPressure 768 non-null int64
3 SkinThickness 768 non-null int64
4 Insulin 768 non-null int64
5 BMI 768 non-null float64
6 DiabetesPedigreeFunction 768 non-null float64
7 Age 768 non-null int64
8 Outcome 768 non-null int64
dtypes: float64(2), int64(7)
memory usage: 54.1 KB
None
```
从数据信息中可以看出,数据集中的每个特征都没有缺失值,因此不需要进行缺失值填充。
接下来,我们需要对数据进行标准化处理和特征选择。
标准化处理:
```python
from sklearn.preprocessing import StandardScaler
# 定义特征和标签
X = data.iloc[:, :-1]
y = data.iloc[:, -1]
# 标准化处理
scaler = StandardScaler()
X_scaled = scaler.fit_transform(X)
# 将标准化后的数据转换为DataFrame类型
X_scaled = pd.DataFrame(X_scaled, columns=X.columns)
```
特征选择:
```python
from sklearn.feature_selection import SelectKBest
from sklearn.feature_selection import chi2
# 特征选择
selector = SelectKBest(chi2, k=4)
X_new = selector.fit_transform(X_scaled, y)
# 选择后的特征
features = X.columns[selector.get_support()]
print(features)
```
根据卡方检验的结果,我们选择了4个特征进行建模,这些特征分别是 Glucose、Insulin、BMI 和 Age。
最后,我们将处理后的数据集和标签整合在一起,并将其划分为训练集和测试集。
```python
from sklearn.model_selection import train_test_split
# 将选择后的特征和标签整合在一起
data_selected = pd.concat([X_new, y], axis=1)
# 划分训练集和测试集
train, test = train_test_split(data_selected, test_size=0.2, random_state=42)
```
阅读全文
相关推荐
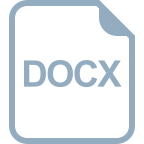









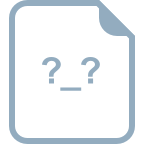
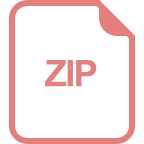
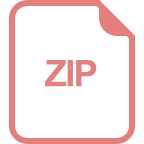
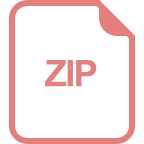
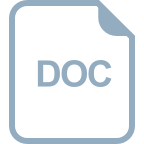

