tf.nn.conv2d
时间: 2023-09-07 11:17:16 浏览: 44
tf.nn.conv2d is a function in TensorFlow that performs a 2D convolution operation on a given input tensor and a set of filters. It is typically used in deep learning models for image processing and computer vision tasks.
The function takes several arguments, including the input tensor, the filter tensor, the strides for the convolution operation, and the padding scheme. The output of the convolution operation is a new tensor that represents the result of applying the filters to the input tensor.
Here is an example usage of tf.nn.conv2d:
```
import tensorflow as tf
# Define input and filter tensors
input_tensor = tf.placeholder(tf.float32, shape=[None, 28, 28, 3])
filter_tensor = tf.Variable(tf.random_normal([5, 5, 3, 32]))
# Perform a 2D convolution operation with strides of 1 and padding of 'SAME'
conv = tf.nn.conv2d(input_tensor, filter_tensor, strides=[1, 1, 1, 1], padding='SAME')
# Run the convolution operation within a TensorFlow session
with tf.Session() as sess:
sess.run(tf.global_variables_initializer())
# Define a sample input tensor
input_data = np.random.rand(1, 28, 28, 3)
# Run the convolution operation on the input tensor
conv_result = sess.run(conv, feed_dict={input_tensor: input_data})
```
In this example, we define an input tensor with a shape of (None, 28, 28, 3), which represents a batch of 28x28 RGB images. We also define a filter tensor with a shape of (5, 5, 3, 32), which represents 32 5x5 filters that will be applied to the input tensor.
We then call tf.nn.conv2d with the input and filter tensors, specifying a stride of 1 and a padding scheme of 'SAME'. This means that the output tensor will have the same spatial dimensions as the input tensor, and that the edges of the input tensor will be zero-padded to ensure that the filters can be applied to all pixels.
Finally, we run the convolution operation within a TensorFlow session, providing a sample input tensor to test the operation. The resulting conv_result tensor will have a shape of (1, 28, 28, 32), representing a batch of 28x28 feature maps for each of the 32 filters applied to the input tensor.
相关推荐
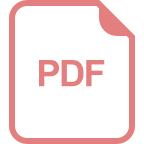
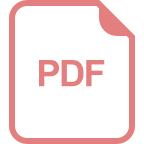
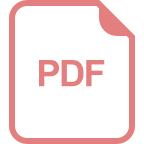














