smooth = .001
时间: 2023-10-07 16:09:20 浏览: 30
这是一个超参数,它通常在优化神经网络的时候使用,用于控制梯度下降时的学习率。具体来说,它是一个小的正数,用于平衡损失函数的权重更新和保持先前的权重值。当学习率太高时,可能会导致权重值来回摆动,影响模型的收敛速度和效果。而当学习率太低时,模型的收敛速度会变慢,导致训练时间变长。因此,设置一个适当的平滑参数可以帮助模型更快地收敛,并且更好地泛化到测试集数据上。通常情况下,平滑参数的值需要在实验中进行调试和优化,以获得最佳的模型性能。
相关问题
Parametric coefficients: Estimate Std. Error t value Pr(>|t|) (Intercept) 0.53529 0.03186 16.8 <2e-16 *** --- Signif. codes: 0 ‘***’ 0.001 ‘**’ 0.01 ‘*’ 0.05 ‘.’ 0.1 ‘ ’ 1 Approximate significance of smooth terms: edf Ref.df F p-value s(X1) 1.818 2.243 0.687 0.5401 s(X2) 1.729 2.080 2.880 0.0675 . s(X3) 1.740 2.110 0.942 0.4003 s(X4) 1.516 1.828 0.973 0.2865 s(X5) 1.727 2.098 0.011 0.9968 s(X6) 2.063 2.478 0.390 0.7171 s(X7) 1.419 1.682 2.056 0.2184 --- Signif. codes: 0 ‘***’ 0.001 ‘**’ 0.01 ‘*’ 0.05 ‘.’ 0.1 ‘ ’ 1 R-sq.(adj) = 0.213 Deviance explained = 42.3% GCV = 0.065102 Scale est. = 0.046688 n = 46这个拟合结果,怎么解释
这个拟合结果是一个 GAM(Generalized Additive Model,广义可加模型)的结果。该模型包含了一个截距项和七个平滑项(s(X1)至s(X7)),其中每个平滑项都是一个自变量对因变量的非线性影响,可以理解为对应自变量的局部回归线。每个平滑项的 edf(estimated degrees of freedom,估计自由度)表示了该平滑项的平滑程度,即该平滑项所对应的局部回归线的灵活性。
拟合结果中还包含了每个参数的估计值(Estimate)、标准误差(Std. Error)、t值(t value)和p值(Pr(>|t|))等信息。其中,t值和p值用于检验每个参数的显著性,p值小于0.05(或0.01、0.001)表示该参数的效应在统计上是显著的。
拟合结果中还包含了模型的拟合优度指标,比如调整后的 R 方值(R-sq.(adj))和解释差异程度(Deviance explained),以及模型的预测误差估计值,比如广义交叉验证误差(GCV)和标准差估计值(Scale est.)等。
需要注意的是,该拟合结果中有些平滑项的p值较大,表明这些平滑项在统计上并不显著。但是,这并不意味着这些自变量对因变量的影响是线性的,而是可能需要更加灵活的非线性模型来描述。
Parametric coefficients: Estimate Std. Error t value Pr(>|t|) (Intercept) 0.52314 0.01395 37.5 <2e-16 *** --- Signif. codes: 0 ‘’ 0.001 ‘’ 0.01 ‘’ 0.05 ‘.’ 0.1 ‘ ’ 1 Approximate significance of smooth terms: edf Ref.df F p-value s(X1) 1.976 1.999 11.034 0.000196 *** s(X2) 1.000 1.000 22.669 3.73e-05 *** s(X3) 1.434 1.670 2.187 0.097375 . s(X4) 1.000 1.000 17.832 0.000178 *** s(X5) 1.875 1.974 6.487 0.007730 ** --- Signif. codes: 0 ‘’ 0.001 ‘’ 0.01 ‘’ 0.05 ‘.’ 0.1 ‘ ’ 1 R-sq.(adj) = 0.943 Deviance explained = 95.4% GCV = 0.01 Scale est. = 0.0079798 n = 41解释下
This output is from a generalized linear model (GLM) with smooth terms included. The "Parametric coefficients" section shows the estimated coefficients for the intercept and any predictor variables that were included as parametric terms. The "Approximate significance of smooth terms" section shows the estimated degrees of freedom (edf), reference degrees of freedom (Ref.df), F-statistic, and p-value for each smooth term included in the model. The "Signif. codes" indicate the level of significance for each term. The "R-sq.(adj)" is the adjusted R-squared value, which indicates the proportion of variance explained by the model after adjusting for the number of predictors included. The "Deviance explained" is the percentage of deviance in the response variable that is explained by the model. The "GCV" is the generalized cross-validation score, which is a measure of predictive accuracy. The "Scale est." is the estimated scale parameter for the model, which represents the variability of the response variable that is not explained by the predictors. The "n" is the sample size.
相关推荐
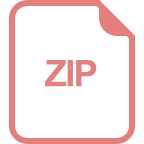
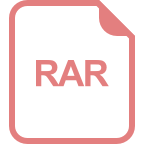












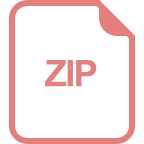