Y = df_dummies['睡眠障碍'] Xtrain,Xtest,Ytrain,Ytest = train_test_split(X,Y,test_size = 0.3) rfc = RandomForestClassifier().fit(Xtrain,Ytrain) print(rfc.score(Xtest,Ytest)) test_scores = [] n_estimators = range(150,200,1) Xtrain,Xtest,Ytrain,Ytest = train_test_split(X,Y,test_size = 0.3) for n in n_estimators: rfc = RandomForestClassifier( n_estimators=n ).fit(Xtrain,Ytrain) test_scores.append(cross_val_score(rfc,Xtest,Ytest,cv =10).mean()) px.line( x = n_estimators, y = test_scores )
时间: 2023-12-24 15:41:25 浏览: 65
这是一个基于随机森林分类器的机器学习模型,用于对睡眠障碍进行预测。其中,X是不包含睡眠障碍的特征矩阵,df_dummies是经过独热编码后的数据集,Y是睡眠障碍的标签。train_test_split函数将数据集分为训练集和测试集,用于模型训练和评估。随机森林分类器是一种集成学习方法,可以用于特征选择和分类预测。通过调整n_estimators参数来寻找最佳模型,cross_val_score函数用于交叉验证模型的准确率。px.line函数将不同n_estimators下的测试准确率绘制成折线图,用于模型选择和调参。
相关问题
代码讲解 from sklearn.preprocessing import OneHotEncoder from sklearn.compose import make_column_transformer column_trans = make_column_transformer( (OneHotEncoder(),['Team1', 'Team2']),remainder='passthrough') pipe_X = pipe_DF.drop('Team1_Result',axis=1) pipe_y = pipe_DF['Team1_Result'] from sklearn.pipeline import make_pipeline pipe_League = make_pipeline(column_trans,StandardScaler(with_mean=False),XGBClassifier(use_label_encoder=False, gamma= 0.01, learning_rate= 0.01, n_estimators= 300, max_depth= 4)) pipe_League.fit(pipe_X,pipe_y) knock_df = pipe_DF[pipe_DF['Team1_Result'] != 2] pipe_knock_df = knock_df knock_df = pd.get_dummies(knock_df) X = knock_df.drop('Team1_Result',axis=1) y = knock_df['Team1_Result'] X_train, X_val, y_train, y_val = train_test_split(X, y, test_size=0.2, random_state=42) X_hold_test, X_test, y_hold_test, y_test = train_test_split(X_val, y_val, test_size=0.5, random_state=42)
这段代码的目的是进行数据预处理和建模,其中包括以下步骤:
1. 导入必要的库:`from sklearn.preprocessing import OneHotEncoder` 和 `from sklearn.compose import make_column_transformer`。
2. 定义列变换器 `column_trans`,使用 `OneHotEncoder()` 对 `['Team1', 'Team2']` 这两列进行独热编码,同时保留其他列(`remainder='passthrough'`)。
3. 将数据分为特征和目标变量,分别存储在 `pipe_X` 和 `pipe_y` 中。
4. 导入管道模型构建库 `from sklearn.pipeline import make_pipeline`。
5. 定义管道模型 `pipe_League`,它包括列变换器 `column_trans`、标准化处理 `StandardScaler(with_mean=False)` 和 XGBoost分类器 `XGBClassifier(use_label_encoder=False, gamma= 0.01, learning_rate= 0.01, n_estimators= 300, max_depth= 4)`。
6. 使用 `fit()` 方法拟合管道模型,将 `pipe_X` 和 `pipe_y` 作为输入数据。
7. 选择需要处理的数据,在这里是将 `pipe_DF` 中 `Team1_Result` 列中的值为 2 的行剔除。
8. 使用 `pd.get_dummies()` 将分类变量进行独热编码。
9. 将特征和目标变量分别存储在 `X` 和 `y` 中。
10. 使用 `train_test_split()` 将数据划分为训练集和验证集(`X_train, X_val, y_train, y_val`)以及保留测试集(`X_hold_test, X_test, y_hold_test, y_test`)。其中,测试集占据验证集的一半,`random_state` 参数用于保证每次运行划分结果相同。
def machine_study_forecast(df): df1=clean_date(df) middle_time = pd.to_datetime('2014-12-14') df1['date'] = pd.to_datetime(df1['date']) df2 = df1[df1['date'] > middle_time] df3 = df1[df1['date'] <= middle_time] df_train=pd.get_dummies(df3['behavior_type'],prefix='behavior_type') print(df_train) y=df_train.behavior_type_4.values y = y.reshape(-1, 1) x=df_train.drop(columns=['behavior_type_4']) x=pd.concat([df3,x],axis=1) x=x.drop(columns=['behavior_type']) print(x) print(y) x_train, x_test, y_train, y_test = train_test_split(x, y, test_size=0.2, random_state=0) ann = Sequential() # 创建一个序贯ANN(Artifical Neural Network)模型 ann.add(Dense(units=8, input_dim=8, activation='relu')) # 添加输入层 ann.add(Dense(units=16, activation='relu')) # 添加隐层 ann.add(Dense(units=1, activation='sigmoid')) # 添加输出层 ann.summary() # 显示网络模型(这个语句不是必须的) # SVG(model_to_dot(ann, show_shapes=True).create(prog='dot', format='svg')) ann.compile(optimizer='adam', # 优化器 loss='binary_crossentropy', # 损失函数 metrics=['acc']) # 评估指标 history = ann.fit(x_train, y_train, # 指定训练集 epochs=30, # 指定训练的轮次 batch_size=64, # 指定数据批量 validation_data=(x_test, y_test)) # 指定验证集,这里为了简化模型,直接用测试集数据进行验证
这是一段Python代码,用于进行机器学习预测。其中的步骤包括数据清理、数据处理(如对日期进行转换)、对数据进行编码、制定输入和输出变量,并对数据进行训练和测试。最后用到了Keras深度学习框架中的Sequential类。
阅读全文
相关推荐
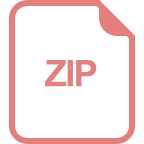
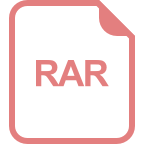
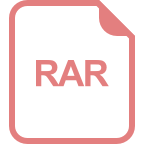














