帮忙优化一下这段代码i=0:0.1:45 ; i0=i*pi/180; r1=70*pi/180; m1=195; K=1500; L=3025; qwz=acot(cot(i0)-K/L); %期望值 P1=sin(r1+i0); Q1=(K/m1)*(2*cos(r1)-cos(r1+i0))-cos(2*r1); R1=sqrt((K/m1)^2+1-2*(K/m1)*cos(r1+i0)); yhq=real(r1-asin(P1./R1)-acos(Q1./R1)); %实际值 plot(i,qwz,'b-'); grid on; hold on; %画在同一张图上 plot(i,yhq,'r+'); hold on; xlabel('外轮转角'); ylabel('内轮转角'); legend('理论转角','实际转角'); title('理论转角VS实际转角');
时间: 2024-02-21 17:00:10 浏览: 189
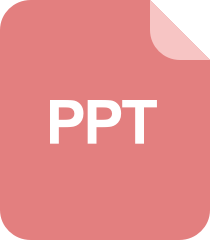
三:Matlab绘图.ppt
这段代码中有一些重复计算的部分,可以将它们提前计算出来,以提高代码的效率。此外,可以考虑使用矩阵运算来避免使用循环。
以下是优化后的代码:
```matlab
i = 0:0.1:45;
i0 = i * pi / 180;
r1 = 70 * pi / 180;
m1 = 195;
K = 1500;
L = 3025;
cot_i0 = cot(i0); % 提前计算 cot(i0)
P1 = sin(r1 + i0);
cos_r1 = cos(r1);
cos_r1_i0 = cos(r1 + i0);
Q1 = (K/m1)*(2*cos_r1-cos_r1_i0)-cos(2*r1);
K_div_m1 = K/m1;
R1 = sqrt(K_div_m1^2+1-2*K_div_m1*cos_r1_i0);
ywq = r1 - asin(P1./R1) - acos(Q1./R1);
plot(i, acot(cot_i0-K/L), 'b-', i, real(ywq), 'r+');
grid on;
xlabel('外轮转角');
ylabel('内轮转角');
legend('理论转角', '实际转角');
title('理论转角 VS 实际转角');
```
这样,代码会更加简洁和高效。
阅读全文
相关推荐
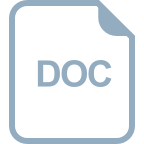
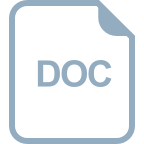
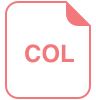
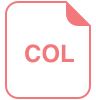
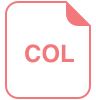
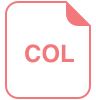
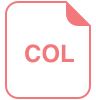









