粒子群优化模糊pid控制代码
时间: 2023-07-08 09:45:46 浏览: 272
以下是一个简单的粒子群优化模糊PID控制的代码示例:
```python
import numpy as np
import skfuzzy as fuzz
from skfuzzy import control as ctrl
import random
class Particle:
def __init__(self, x0):
self.position = x0
self.velocity = np.zeros_like(x0)
self.best_position = x0
self.error = -1
def evaluate(self, costFunc):
self.error = costFunc(self.position)
if self.error < costFunc(self.best_position):
self.best_position = self.position
def update_velocity(self, best_global_pos):
w = 0.5
c1 = 1
c2 = 2
r1 = random.random()
r2 = random.random()
cognitive_velocity = c1 * r1 * (self.best_position - self.position)
social_velocity = c2 * r2 * (best_global_pos - self.position)
self.velocity = w * self.velocity + cognitive_velocity + social_velocity
def update_position(self, bounds):
self.position = self.position + self.velocity
for i in range(len(bounds)):
if self.position[i] > bounds[i][1]:
self.position[i] = bounds[i][1]
if self.position[i] < bounds[i][0]:
self.position[i] = bounds[i][0]
class PIDController:
def __init__(self, Kp, Ki, Kd):
self.Kp = Kp
self.Ki = Ki
self.Kd = Kd
self.error = 0
self.error_prev = 0
self.integral = 0
def compute(self, SP, PV):
error = SP - PV
self.integral = self.integral + error
derivative = error - self.error_prev
output = self.Kp * error + self.Ki * self.integral + self.Kd * derivative
self.error_prev = error
return output
def fuzzy_cost(x):
Kp = x[0]
Ki = x[1]
Kd = x[2]
error = 0
SP = 50
# 定义模糊变量和模糊集合
error_fuzzy = ctrl.Antecedent(np.arange(-100, 100, 1), 'error')
output_fuzzy = ctrl.Consequent(np.arange(-100, 100, 1), 'output')
# 定义模糊集合
error_fuzzy['negative'] = fuzz.trimf(error_fuzzy.universe, [-100, -100, 0])
error_fuzzy['zero'] = fuzz.trimf(error_fuzzy.universe, [-100, 0, 100])
error_fuzzy['positive'] = fuzz.trimf(error_fuzzy.universe, [0, 100, 100])
output_fuzzy['negative'] = fuzz.trimf(output_fuzzy.universe, [-100, -100, 0])
output_fuzzy['zero'] = fuzz.trimf(output_fuzzy.universe, [-100, 0, 100])
output_fuzzy['positive'] = fuzz.trimf(output_fuzzy.universe, [0, 100, 100])
# 定义模糊规则
rule1 = ctrl.Rule(error_fuzzy['negative'], output_fuzzy['negative'])
rule2 = ctrl.Rule(error_fuzzy['zero'], output_fuzzy['zero'])
rule3 = ctrl.Rule(error_fuzzy['positive'], output_fuzzy['positive'])
# 控制系统
pid_ctrl = ctrl.ControlSystem([rule1, rule2, rule3])
pid_sim = ctrl.ControlSystemSimulation(pid_ctrl)
# 计算模糊PID控制器输出
for i in range(len(PV)):
pid_sim.input['error'] = SP - PV[i]
pid_sim.compute()
output = pid_sim.output['output']
error = error + (SP - PV[i]) ** 2 + output ** 2
return error
def PSO(costFunc, x0, bounds, num_particles, maxiter):
global best_global_position
global best_global_error
best_global_error = -1
best_global_position = None
swarm = []
for i in range(num_particles):
x = Particle(x0)
swarm.append(x)
for i in range(maxiter):
for j in range(num_particles):
swarm[j].evaluate(costFunc)
if swarm[j].error < best_global_error or best_global_error == -1:
best_global_position = swarm[j].position
best_global_error = swarm[j].error
for j in range(num_particles):
swarm[j].update_velocity(best_global_position)
swarm[j].update_position(bounds)
return best_global_position
# 测试代码
if __name__ == "__main__":
# 设置PID控制器参数初值
Kp = 1
Ki = 0.1
Kd = 0.01
x0 = [Kp, Ki, Kd]
bounds = [(0, 10), (0, 1), (0, 0.1)]
num_particles = 100
maxiter = 50
# 运行PSO算法进行优化
best_parameters = PSO(fuzzy_cost, x0, bounds, num_particles, maxiter)
# 输出最优参数
print("最优参数:Kp = %.2f, Ki = %.2f, Kd = %.2f" % (best_parameters[0], best_parameters[1], best_parameters[2]))
```
需要注意的是,上述代码仅为示例,实际应用中还需要根据具体的控制对象和控制要求进行适当的修改和调整。
阅读全文
相关推荐













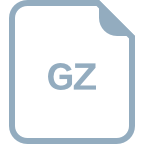