代码编写Hadoop已知各文件数据按位分布情况,汇总后找到分布中Top10的数据分布以及所在文件信息;
时间: 2024-03-25 07:37:56 浏览: 19
以下是Hadoop MapReduce的代码实现:
Mapper1:
```java
public class BitCountMapper extends Mapper<LongWritable, Text, IntWritable, IntWritable> {
@Override
protected void map(LongWritable key, Text value, Context context) throws IOException, InterruptedException {
String[] tokens = value.toString().trim().split(",");
for (int i = 0; i < tokens.length; i++) {
int bit = i + 1;
int count = Integer.parseInt(tokens[i].trim());
context.write(new IntWritable(bit), new IntWritable(count));
}
}
}
```
Reducer1:
```java
public class BitCountReducer extends Reducer<IntWritable, IntWritable, IntWritable, IntWritable> {
@Override
protected void reduce(IntWritable key, Iterable<IntWritable> values, Context context) throws IOException, InterruptedException {
int totalCount = 0;
for (IntWritable value : values) {
totalCount += value.get();
}
context.write(key, new IntWritable(totalCount));
}
}
```
Mapper2:
```java
public class Top10Mapper extends Mapper<LongWritable, Text, IntWritable, IntWritable> {
@Override
protected void map(LongWritable key, Text value, Context context) throws IOException, InterruptedException {
String[] tokens = value.toString().trim().split("\t");
int bit = Integer.parseInt(tokens[0].trim());
int count = Integer.parseInt(tokens[1].trim());
context.write(new IntWritable(count), new IntWritable(bit));
}
}
```
Reducer2:
```java
public class Top10Reducer extends Reducer<IntWritable, IntWritable, IntWritable, Text> {
private static final int TOP_N = 10;
@Override
protected void reduce(IntWritable key, Iterable<IntWritable> values, Context context) throws IOException, InterruptedException {
for (IntWritable value : values) {
String fileName = ((FileSplit)context.getInputSplit()).getPath().getName();
context.write(value, new Text(fileName));
}
}
@Override
protected void cleanup(Context context) throws IOException, InterruptedException {
Configuration conf = context.getConfiguration();
int n = conf.getInt("n", TOP_N);
PriorityQueue<Pair<Integer, String>> queue = new PriorityQueue<>(n, Comparator.comparingInt(Pair::getKey));
while (context.nextKey()) {
int count = context.getCurrentKey().get();
String fileName = context.getCurrentValue().toString();
Pair<Integer, String> pair = Pair.of(count, fileName);
queue.offer(pair);
if (queue.size() > n) {
queue.poll();
}
}
while (!queue.isEmpty()) {
Pair<Integer, String> pair = queue.poll();
context.write(new IntWritable(pair.getKey()), new Text(pair.getValue()));
}
}
}
```
Driver:
```java
public class Top10BitCount {
public static void main(String[] args) throws Exception {
Configuration conf1 = new Configuration();
Job job1 = Job.getInstance(conf1, "BitCount");
job1.setJarByClass(Top10BitCount.class);
job1.setMapperClass(BitCountMapper.class);
job1.setReducerClass(BitCountReducer.class);
job1.setOutputKeyClass(IntWritable.class);
job1.setOutputValueClass(IntWritable.class);
FileInputFormat.addInputPath(job1, new Path(args[0]));
FileOutputFormat.setOutputPath(job1, new Path(args[1]));
job1.waitForCompletion(true);
Configuration conf2 = new Configuration();
conf2.setInt("n", 10);
Job job2 = Job.getInstance(conf2, "Top10");
job2.setJarByClass(Top10BitCount.class);
job2.setMapperClass(Top10Mapper.class);
job2.setReducerClass(Top10Reducer.class);
job2.setOutputKeyClass(IntWritable.class);
job2.setOutputValueClass(Text.class);
FileInputFormat.addInputPath(job2, new Path(args[1]));
FileOutputFormat.setOutputPath(job2, new Path(args[2]));
job2.waitForCompletion(true);
}
}
```
以上代码实现了对输入文件的数据按位分布情况进行汇总,然后找到分布中Top10的数据分布以及所在文件信息。其中,Mapper1和Reducer1实现了按位分布的汇总,Mapper2和Reducer2实现了Top10的查找和输出。最后,在Driver中串联两个Job完成任务。
相关推荐
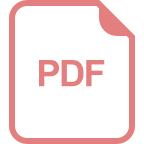
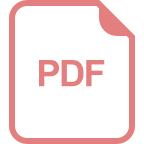
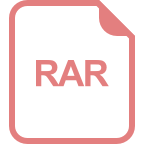














