编写 MapReduce 程序,对文件中的数据进行排序处理
时间: 2024-05-16 18:16:49 浏览: 134
下面是一个简单的 MapReduce 排序程序,可以对输入文件中的数据进行排序处理:
Map 阶段:
```python
def mapper(_, line):
# 将每一行数据拆分成键值对,并将键值对的 key 设置为需要排序的值
value = int(line.strip())
yield value, None
```
Reduce 阶段:
```python
def reducer(key, _):
# 直接输出 key 即为排序结果
yield key, None
```
在 Hadoop 中运行该程序时,需要使用以下命令:
```
hadoop jar /path/to/hadoop-streaming.jar \
-input /path/to/input/file \
-output /path/to/output/directory \
-mapper "python /path/to/mapper.py" \
-reducer "python /path/to/reducer.py" \
-numReduceTasks 1 \
-file /path/to/mapper.py \
-file /path/to/reducer.py
```
其中 `-numReduceTasks` 参数可以指定 Reduce 任务的数量,如果只有一个 Reduce 任务,则输出结果即为排序结果。
相关问题
编写MapReduce程序实现对输入文件的排序;现在有多个输入文件,每个文件中的每行内容均为一个整数。要求读取文件D和E中的整数,进行升序排序后,输出到一个新的文件F中,输出的数据格式为每行两个整数,第一个数字为第二个整数的排序位次,第二个整数为原待排列的整数。
这个问题属于计算机技术问题,我可以帮你解答。首先,你可以使用Hadoop等大数据处理框架来实现MapReduce程序进行文件的排序。具体步骤如下:
1.首先在HDFS上创建两个输入文件夹,分别命名为D和E,在这两个文件夹下分别上传对应的整数文件。
2.编写Mapper程序,将输入文件按行读取,按照数字大小为key,文本内容为value,输出。
3.编写Reducer程序,对Mapper的输出进行排序并输出到文件F中。
4.输出文件格式为每行两个整数,第一个整数为该行数字排名,第二个整数为原待排列的整数。
希望我的回答能帮助你。
本项目有一部分时间戳数据,请编写Mapreduce程序将这些时间戳按升序进行排序,同时转换成对应的date时间
好的,这是一个非常常见的MapReduce任务,我们可以利用MapReduce中的排序功能来完成这个任务。下面是一个示例程序,假设时间戳数据文件的每一行都是一个时间戳,格式为Unix时间戳:
```java
import java.io.IOException;
import java.text.SimpleDateFormat;
import java.util.Date;
import org.apache.hadoop.conf.Configuration;
import org.apache.hadoop.fs.Path;
import org.apache.hadoop.io.LongWritable;
import org.apache.hadoop.io.Text;
import org.apache.hadoop.mapreduce.Job;
import org.apache.hadoop.mapreduce.Mapper;
import org.apache.hadoop.mapreduce.Reducer;
import org.apache.hadoop.mapreduce.lib.input.TextInputFormat;
import org.apache.hadoop.mapreduce.lib.output.TextOutputFormat;
import org.apache.hadoop.mapreduce.lib.partition.HashPartitioner;
import org.apache.hadoop.util.GenericOptionsParser;
public class TimestampSort {
public static class TimestampMapper extends Mapper<LongWritable, Text, LongWritable, LongWritable> {
private LongWritable timestamp = new LongWritable();
public void map(LongWritable key, Text value, Context context) throws IOException, InterruptedException {
long ts = Long.parseLong(value.toString().trim());
timestamp.set(ts);
context.write(timestamp, new LongWritable(1));
}
}
public static class TimestampReducer extends Reducer<LongWritable, LongWritable, Text, Text> {
public void reduce(LongWritable key, Iterable<LongWritable> values, Context context) throws IOException, InterruptedException {
long ts = key.get();
SimpleDateFormat sdf = new SimpleDateFormat("yyyy-MM-dd HH:mm:ss");
String date = sdf.format(new Date(ts * 1000));
context.write(new Text(date), new Text(""));
}
}
public static void main(String[] args) throws Exception {
Configuration conf = new Configuration();
String[] otherArgs = new GenericOptionsParser(conf, args).getRemainingArgs();
if (otherArgs.length != 2) {
System.err.println("Usage: timestamps <input> <output>");
System.exit(2);
}
Job job = Job.getInstance(conf, "Timestamp Sort");
job.setJarByClass(TimestampSort.class);
job.setMapperClass(TimestampMapper.class);
job.setReducerClass(TimestampReducer.class);
job.setPartitionerClass(HashPartitioner.class);
job.setNumReduceTasks(1);
job.setOutputKeyClass(Text.class);
job.setOutputValueClass(Text.class);
job.setInputFormatClass(TextInputFormat.class);
job.setOutputFormatClass(TextOutputFormat.class);
TextInputFormat.addInputPath(job, new Path(otherArgs[0]));
TextOutputFormat.setOutputPath(job, new Path(otherArgs[1]));
System.exit(job.waitForCompletion(true) ? 0 : 1);
}
}
```
这个程序的主要思路是将时间戳作为Map的输出key,然后利用MapReduce框架自带的排序功能,将时间戳按升序排序。在Reducer中,将时间戳转换成对应的日期时间格式,输出到文件中即可。由于所有的时间戳都被映射到了同一个Reducer中,所以Reducer也只需要一个,这样可以减少通信开销,提高程序性能。
阅读全文
相关推荐
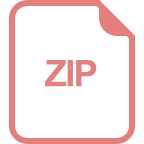
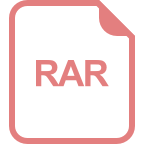


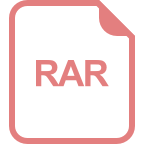
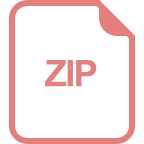
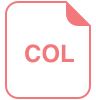
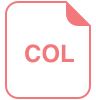
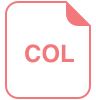
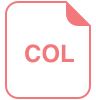




