nn.BatchNorm2d(out_planes)的out_planes是什么
时间: 2024-04-15 13:24:13 浏览: 164
`out_planes` 是指定批标准化层 (`BatchNorm2d`) 的输出通道数。在深度学习中,卷积神经网络 (Convolutional Neural Networks, CNNs) 经常使用批标准化来加速训练和提高模型的性能。批标准化层的输入是一个具有多个通道的特征图(feature map),而输出也是具有相同通道数的特征图。`out_planes` 参数用于指定输出特征图的通道数,即决定了批标准化层的输出形状。
相关问题
class Block(nn.Module): def __init__(self, in_planes, out_planes, expansion, stride): super(Block, self).__init__() self.stride = stride planes = expansion * in_planes self.conv1 = nn.Conv2d(in_planes, planes, kernel_size=1, bias=False) self.bn1 = nn.BatchNorm2d(planes) self.conv2 = nn.Conv2d(planes, planes, kernel_size=3, stride=stride, padding=1, groups=planes, bias=False) self.bn2 = nn.BatchNorm2d(planes) self.conv3 = nn.Conv2d(planes, out_planes, kernel_size=1, bias=False) self.bn3 = nn.BatchNorm2d(out_planes) self.shortcut = nn.Sequential() if stride == 1 and in_planes != out_planes: self.shortcut = nn.Sequential( nn.Conv2d(in_planes, out_planes, kernel_size=1, stride=stride, bias=False), nn.BatchNorm2d(out_planes) )
这是一个基本的残差块(Residual Block)类。在初始化函数中,它接收输入通道数(in_planes)、输出通道数(out_planes)、扩展倍数(expansion)和步长(stride)作为参数。在初始化过程中,它定义了一系列的卷积层和批归一化层。
具体来说,它包含以下几个层:
- self.conv1:一个1x1的卷积层,用于对输入进行通道数的转换,将输入通道数变为扩展倍数乘以输入通道数。
- self.bn1:对conv1的输出进行批归一化操作。
- self.conv2:一个3x3的卷积层,用于在空间上对特征进行卷积操作。
- self.bn2:对conv2的输出进行批归一化操作。
- self.conv3:一个1x1的卷积层,用于将特征映射的通道数变为输出通道数。
- self.bn3:对conv3的输出进行批归一化操作。
此外,如果步长为1并且输入通道数与输出通道数不相等,则会添加一个shortcut(短连接)来使输入与输出形状匹配。shortcut由一个包含1x1卷积层和批归一化层的Sequential组成。
这个残差块类用于构建ResNet等网络结构。
expansion = 1 def __init__(self, in_planes, planes, stride=1): super(BasicBlock, self).__init__() self.conv1 = nn.Conv2d(in_planes, planes, kernel_size=3, stride=stride, padding=1, bias=False) self.bn1 = nn.BatchNorm2d(planes) self.conv2 = nn.Conv2d(planes, planes, kernel_size=3, stride=1, padding=1, bias=False) self.bn2 = nn.BatchNorm2d(planes) self.shortcut = nn.Sequential() if stride != 1 or in_planes != self.expansion * planes: self.shortcut = nn.Sequential( nn.Conv2d(in_planes, self.expansion * planes, kernel_size=1, stride=stride, bias=False), nn.BatchNorm2d(self.expansion * planes) ) def forward(self, x): out = nn.ReLU()(self.bn1(self.conv1(x))) out = self.bn2(self.conv2(out)) out += self.shortcut(x) out = nn.ReLU()(out) return out其中的expansion是什么意思
在给定的代码中,`expansion` 是一个类变量,表示 BasicBlock 中通道数的扩展倍数。它用于确定在残差连接中使用的卷积层的输出通道数。
在 ResNet 等架构中,残差块通常会对输入进行降采样(stride 不等于 1)或者通道数进行扩展。当输入通道数与输出通道数不匹配时,需要使用一个额外的 1x1 卷积层将输入通道数扩展到相应的输出通道数。`expansion` 变量就是用来控制这个扩展倍数的。
在代码中的这段逻辑中,如果 stride 不等于 1 或者输入通道数 `in_planes` 不等于 `self.expansion * planes`,则会创建一个包含一个 1x1 卷积层和一个批归一化层的 `shortcut` 模块,用于将输入通道数进行扩展。
总之,`expansion` 变量用于确定残差块中卷积层通道数的扩展倍数,并控制残差连接中输入通道数与输出通道数的匹配。
阅读全文
相关推荐
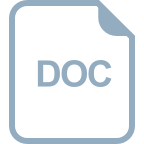
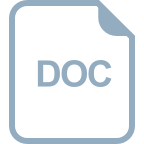
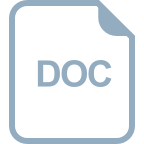





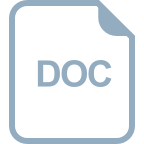
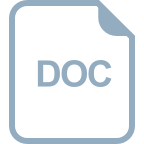
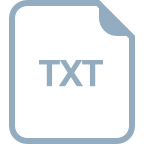
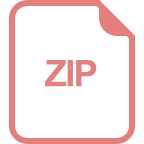
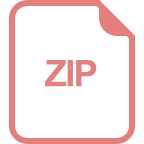
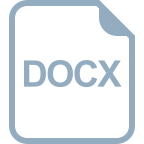
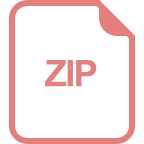
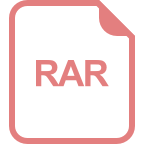