python代码实现混沌
时间: 2023-11-25 10:52:40 浏览: 118
以下是Python实现混沌的代码:
```python
def chaotic_function(x):
for i in range(10):
x = 3.9 * x * (1 - x)
print(x)
chaotic_function(0.5)
```
该代码定义了一个名为chaotic_function的函数,该函数接受一个参数x。在函数内部,使用for循环迭代10次,每次计算x的新值并将其打印出来。计算新值的公式为3.9 * x * (1 - x)。最后,我们调用该函数并传入初始值0.5。
相关问题
python 实现logistics混沌加密png图像
实现这个功能需要使用到Python的Pillow库来读取和写入PNG图像,以及numpy库来进行矩阵计算。
首先,我们需要实现logistics混沌算法,可以使用以下代码:
```python
import numpy as np
def logistics_chaos(x, a=4.0):
return a * x * (1 - x)
def generate_chaos_sequence(x0, n):
result = np.zeros(n)
result[0] = x0
for i in range(1, n):
result[i] = logistics_chaos(result[i-1])
return result
```
其中,`logistics_chaos()`函数实现了logistics混沌算法,`generate_chaos_sequence()`函数生成了一个混沌序列。
接下来,我们需要将PNG图像转换为numpy数组,并将其压缩成一维数组,可以使用以下代码:
```python
from PIL import Image
def compress_image(image):
img = Image.open(image)
img_array = np.array(img)
return img_array.flatten()
```
然后,我们需要使用生成的混沌序列对图像进行加密,可以使用以下代码:
```python
def encrypt_image(image, x0=0.5, a=4.0):
img_data = compress_image(image)
chaos_seq = generate_chaos_sequence(x0, len(img_data))
encrypted_data = np.bitwise_xor(np.array(img_data), np.array(chaos_seq))
return encrypted_data
```
其中,`np.bitwise_xor()`函数对数组进行异或运算,实现了加密操作。
最后,我们需要将加密后的数据重新写入PNG图像文件中,可以使用以下代码:
```python
def save_image(data, filename):
img_array = np.reshape(data, (-1, 3))
img = Image.fromarray(np.uint8(img_array))
img.save(filename)
def decrypt_image(data, x0=0.5, a=4.0):
chaos_seq = generate_chaos_sequence(x0, len(data))
decrypted_data = np.bitwise_xor(np.array(data), np.array(chaos_seq))
return decrypted_data
def main():
# 加密图像
encrypted_data = encrypt_image('test.png')
save_image(encrypted_data, 'encrypted.png')
# 解密图像
decrypted_data = decrypt_image(encrypted_data)
save_image(decrypted_data, 'decrypted.png')
if __name__ == '__main__':
main()
```
其中,`save_image()`函数将数据重新写入PNG图像文件中,`decrypt_image()`函数对数据进行解密操作。
完整代码如下:
```python
import numpy as np
from PIL import Image
def logistics_chaos(x, a=4.0):
return a * x * (1 - x)
def generate_chaos_sequence(x0, n):
result = np.zeros(n)
result[0] = x0
for i in range(1, n):
result[i] = logistics_chaos(result[i-1])
return result
def compress_image(image):
img = Image.open(image)
img_array = np.array(img)
return img_array.flatten()
def encrypt_image(image, x0=0.5, a=4.0):
img_data = compress_image(image)
chaos_seq = generate_chaos_sequence(x0, len(img_data))
encrypted_data = np.bitwise_xor(np.array(img_data), np.array(chaos_seq))
return encrypted_data
def save_image(data, filename):
img_array = np.reshape(data, (-1, 3))
img = Image.fromarray(np.uint8(img_array))
img.save(filename)
def decrypt_image(data, x0=0.5, a=4.0):
chaos_seq = generate_chaos_sequence(x0, len(data))
decrypted_data = np.bitwise_xor(np.array(data), np.array(chaos_seq))
return decrypted_data
def main():
# 加密图像
encrypted_data = encrypt_image('test.png')
save_image(encrypted_data, 'encrypted.png')
# 解密图像
decrypted_data = decrypt_image(encrypted_data)
save_image(decrypted_data, 'decrypted.png')
if __name__ == '__main__':
main()
```
需要注意的是,由于logistics混沌算法对初始值x0非常敏感,因此需要保证每次加密和解密使用的x0相同。此外,由于加密操作是基于像素级别的,因此加密后的图像可能会出现一些噪点。
tent混沌映射python代码
下面是使用 Python 实现 Tent 混沌映射的代码:
```python
import matplotlib.pyplot as plt
def tent_map(x, a):
if x < a:
return x / a
else:
return (1 - x) / (1 - a)
# 设置参数
a = 0.7
x0 = 0.2
n = 1000
# 生成混沌序列
x = [x0]
for i in range(n):
x.append(tent_map(x[-1], a))
# 绘制混沌图像
plt.plot(x)
plt.title("Tent Map with a = {}".format(a))
plt.xlabel("Iteration")
plt.ylabel("Value")
plt.show()
```
在上面的代码中,我们首先定义了 Tent 映射函数 `tent_map(x, a)`,该函数接受两个参数 `x` 和 `a`,并返回映射后的结果。然后我们设置了 Tent 映射的参数 `a`,初始值 `x0`,以及迭代次数 `n`。接下来,我们使用一个 for 循环来计算混沌序列,并将结果保存在列表 `x` 中。最后,我们使用 Matplotlib 库来绘制混沌图像。
请注意,Tent 映射函数中的参数 `a` 应该取值在 [0, 1] 的范围内。而在实际应用中,我们通常将初始值 `x0` 取为一个随机数。
阅读全文
相关推荐
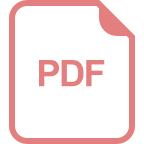
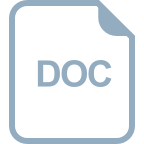
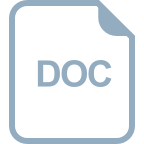
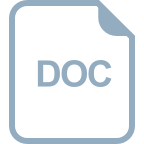
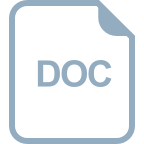
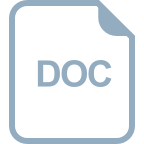
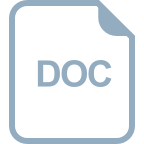
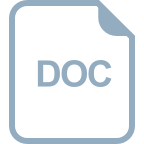
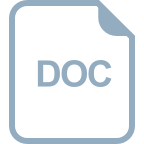
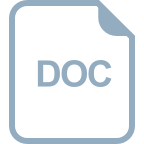




