# Model print(colored('Retrieve model', 'blue')) model = get_model(p) print('Model is {}'.format(model.__class__.__name__)) print('Model parameters: {:.2f}M'.format(sum(p.numel() for p in model.parameters()) / 1e6)) print(model) model = model.cuda(),这句话是什么意思
时间: 2024-04-28 11:20:32 浏览: 19
这段代码主要是用于构建模型,并将模型移动到 GPU 上进行加速运算。其中,`get_model` 是一个函数,它根据配置文件 `p` 中的参数返回一个模型对象 `model`。`print('Model is {}'.format(model.__class__.__name__))` 输出模型的类名。`print('Model parameters: {:.2f}M'.format(sum(p.numel() for p in model.parameters()) / 1e6))` 输出模型参数的数量,单位为百万(M)。最后,`model = model.cuda()` 是将模型移动到 GPU 上,以便进行加速计算。
相关问题
p = create_config(args.config_env, args.config_exp) print(colored(p, 'red')) # Model print(colored('Retrieve model', 'blue')) model = get_model(p) print('Model is {}'.format(model.__class__.__name__)) print('Model parameters: {:.2f}M'.format(sum(p.numel() for p in model.parameters()) / 1e6)) print(model) model = model.cuda()
这段代码使用了前面定义的`create_config`函数和`get_model`函数,根据命令行参数解析出的配置文件路径,获取配置参数并获取模型。
首先,调用`create_config`函数读取环境配置文件和实验配置文件,并将读取到的配置参数保存在变量`p`中。然后,使用`print(colored(p, 'red'))`语句将配置参数以红色字体输出到控制台,方便用户查看。
接着,使用`get_model`函数获取模型,传入参数`p`表示实验配置参数。然后,使用`print`语句输出模型的类名、参数数量以及模型结构信息。最后,将模型移动到GPU上,以便在GPU上进行训练。
通过这段代码,可以方便地读取和管理配置参数,并获取模型对象。这样可以大大简化代码的编写,提高代码的可读性和可维护性。同时,将模型移动到GPU上,也提高了模型训练的效率。
tst_print_colored
tst_print_colored是一个C语言库函数,它用于在终端上以不同的颜色输出文本。该函数可以让终端输出的文本更加醒目,方便用户区分不同的信息。
使用tst_print_colored函数需要包含头文件tst_utils.h,并按照以下方式调用函数:
```
void tst_print_colored(enum tst_color color, const char* format, ...);
```
其中,color参数指定输出文本的颜色;format和...参数指定输出的文本内容和格式。
tst_print_colored函数支持以下颜色枚举类型:
-***
- TST_COLOR_BLUE:蓝色
- TST_COLOR_MAGENTA:洋红色
- TST_COLOR_CYAN:青色
- TST_COLOR_LIGHT_GRAY:浅灰色
- TST_COLOR_DARK_GRAY:深灰色
相关推荐
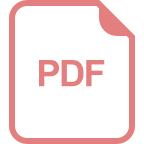
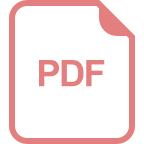
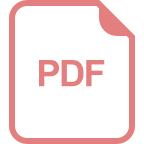













