sns.set_palette('husl')
时间: 2024-01-15 13:02:47 浏览: 127
这是什么意思呢?
这是 Python 的 Seaborn 库中的一个函数调用,用于设置绘图时使用的颜色板。具体来说,它会将颜色按照一定的规则映射到一组颜色值中,并将这些颜色值应用到绘图中。其中,'husl' 是一种颜色映射方案,它可以产生一组比较均匀分布的颜色。这个函数的作用是让绘图变得更加美观和易于区分。
相关问题
怎么使用ns.color_palette()函数并传递我喜欢的预设名称
在seaborn (sns) 中,`color_palette()` 函数是一个非常有用的工具,它允许你创建一系列颜色,通常用于数据可视化中的多种图形元素。如果你想自定义配色方案,只需向该函数传递一个你喜欢的颜色名称、调色板名称或者具体的颜色列表。
例如,如果你想使用matplotlib的预设颜色名称,可以这样做:
```python
import seaborn as sns
from matplotlib import cm
# 使用matplotlib的内置调色板
my_colors = sns.color_palette('Set2') # 这里用了Set2调色板
print(my_colors)
# 或者指定特定的颜色名称
my_colors = sns.color_palette(['red', 'green', 'blue'])
```
如果你想使用更复杂或者自定义的颜色命名,可以查阅matplotlib的颜色字典,如`cm.get_cmap(name)`,这里name是你喜欢的颜色名字。
另外,如果想使用 seaborn 自带的一些预设调色板,可以直接传入相应的字符串,比如 `sns.diverging_palette` 用于创建对比鲜明的渐变配色,`sns.husl_palette` 则适用于生成美观的有对比度的色彩组合等。
一旦获得了颜色列表,你可以直接在你的图表上设置`palette`参数,如之前提到的`sns.pointplot`函数:
```python
pointplot_colors = my_colors
sns.pointplot(x='波形', y='损耗', hue='温度', data=data, palette=pointplot_colors)
```
修改下列代码,使得最后得到的图中每个类别的颜色都不一样:import pandas as pd import matplotlib.pyplot as plt # 将 Release Date 列转化为年份格式 df['year'] = pd.to_datetime(df['Release Date']).dt.year df['Genre'] = df['Genre'].apply(lambda x: eval(x)) genre_counts = df.groupby('year')['Genre'].apply(lambda x: pd.Series(x).value_counts(normalize=True)).unstack(fill_value=0) genre_counts.plot(kind='bar', stacked=True, figsize=(10, 6)) plt.title('Genre Distribution Over the Years') plt.xlabel('Year') plt.ylabel('Frequency') ax.set_xlim(df['year'].min(), 2021) plt.legend(loc='upper left', bbox_to_anchor=(1.05, 1)) plt.show()
import pandas as pd
import matplotlib.pyplot as plt
import seaborn as sns
# 将 Release Date 列转化为年份格式
df['year'] = pd.to_datetime(df['Release Date']).dt.year
df['Genre'] = df['Genre'].apply(lambda x: eval(x))
# 利用 seaborn 调色板来设置颜色
sns.set_palette("husl")
genre_counts = df.groupby('year')['Genre'].apply(lambda x: pd.Series(x).value_counts(normalize=True)).unstack(fill_value=0)
genre_counts.plot(kind='bar', stacked=True, figsize=(10, 6))
plt.title('Genre Distribution Over the Years')
plt.xlabel('Year')
plt.ylabel('Frequency')
ax.set_xlim(df['year'].min(), 2021)
plt.legend(loc='upper left', bbox_to_anchor=(1.05, 1))
plt.show()
阅读全文
相关推荐
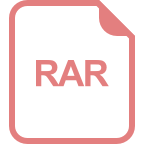
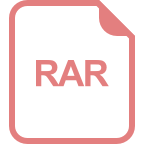
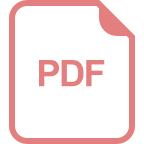
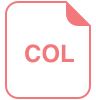


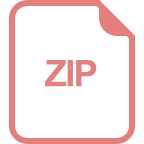
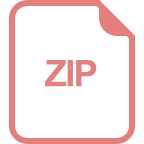