pytorch图像质量评价代码
时间: 2023-07-13 09:33:23 浏览: 40
以下是基于PyTorch实现的图像质量评价的代码示例:
```python
import torch
import torch.nn as nn
import torch.nn.functional as F
class SSIM(nn.Module):
def __init__(self, window_size=11, size_average=True):
super(SSIM, self).__init__()
self.window_size = window_size
self.size_average = size_average
self.channel = 1
self.window = self.create_window(window_size, self.channel)
def gaussian(self, window_size, sigma):
gauss = torch.Tensor([math.exp(-(x - window_size//2)**2/float(2*sigma**2)) for x in range(window_size)])
return gauss/gauss.sum()
def create_window(self, window_size, channel):
_1D_window = self.gaussian(window_size, 1.5).unsqueeze(1)
_2D_window = _1D_window.mm(_1D_window.t()).float().unsqueeze(0).unsqueeze(0)
window = _2D_window.expand(channel, 1, window_size, window_size).contiguous()
return window
def forward(self, img1, img2):
(_, channel, _, _) = img1.size()
if channel == self.channel and self.window.dtype == img1.dtype:
window = self.window
else:
window = self.create_window(self.window_size, channel).to(img1.device).type(img1.dtype)
self.window = window
self.channel = channel
mu1 = F.conv2d(img1, window, padding=self.window_size//2, groups=channel)
mu2 = F.conv2d(img2, window, padding=self.window_size//2, groups=channel)
mu1_sq = mu1.pow(2)
mu2_sq = mu2.pow(2)
mu1_mu2 = mu1*mu2
sigma1_sq = F.conv2d(img1*img1, window, padding=self.window_size//2, groups=channel) - mu1_sq
sigma2_sq = F.conv2d(img2*img2, window, padding=self.window_size//2, groups=channel) - mu2_sq
sigma12 = F.conv2d(img1*img2, window, padding=self.window_size//2, groups=channel) - mu1_mu2
C1 = (0.01*255)**2
C2 = (0.03*255)**2
ssim_map = ((2*mu1_mu2 + C1)*(2*sigma12 + C2)) / ((mu1_sq + mu2_sq + C1)*(sigma1_sq + sigma2_sq + C2))
if self.size_average:
return ssim_map.mean()
else:
return ssim_map.mean(1).mean(1).mean(1)
class PSNR(nn.Module):
def __init__(self, max_value=255.0):
super(PSNR, self).__init__()
self.max_value = max_value
def forward(self, img1, img2):
mse = torch.mean((img1 - img2) ** 2)
if mse == 0:
return 100
return 10 * torch.log10(self.max_value ** 2 / mse)
```
其中,SSIM和PSNR分别是评价图像结构相似性和峰值信噪比的指标。可以在训练过程中使用这些指标来评价生成的图像质量。
相关推荐
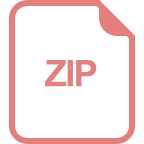
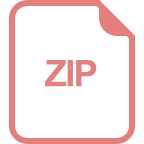
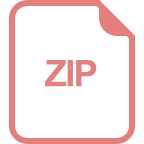
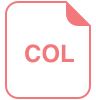

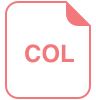

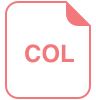









