df.drop(df[(df.score < 50) & (df.score > 20)].index, inplace=True)
时间: 2024-04-16 08:25:16 浏览: 89
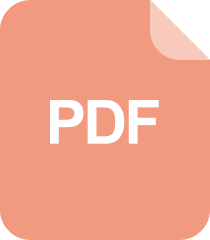
df.rank & pd.pivot_table & pd.read_excel & df添加行 &调整df行列顺序(reindex再理解)
这是一行代码,它使用了 Pandas 库中的 `drop` 函数来删除 DataFrame 中满足特定条件的行。在这个例子中,它删除了 `score` 列中取值在 20 到 50 之间的行。
具体来说,`df.score < 50` 和 `df.score > 20` 是两个条件,它们通过逻辑与运算符 `&` 结合起来,表示要筛选出 `score` 列中取值小于 50 且大于 20 的行。`.index` 表示返回满足条件的行的索引。最后,`inplace=True` 表示在原始 DataFrame 上进行修改。
总结起来,这行代码的作用是删除 DataFrame 中 `score` 列取值在 20 到 50 之间的行,并在原始 DataFrame 上进行修改。
阅读全文
相关推荐
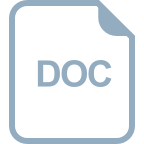
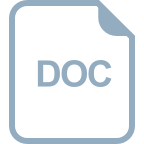





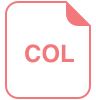









