for train_index, test_index in kf.split(X, y): print('\n{} of kfold {}'.format(i,kf.n_splits)) X_train, X_test = X[train_index], X[test_index] y_train, y_test = y[train_index], y[test_index]
时间: 2023-11-29 09:44:53 浏览: 41
这段代码使用了k-fold交叉验证来划分数据集为训练集和测试集。kf是一个KFold对象,split方法用于生成训练集和测试集的索引。循环中的train_index和test_index表示当前折的训练集和测试集的索引。
在循环体内,首先打印出当前是第几折的信息。然后根据索引将原始数据集X和标签y划分为训练集和测试集,分别赋值给X_train、X_test、y_train和y_test。这样就完成了一次交叉验证的划分。
相关问题
import pandas as pd import numpy as np from sklearn.model_selection import StratifiedKFold, KFold from sklearn.svm import SVR train = pd.read_csv('train.csv') test = pd.read_csv('test.csv') # 分离数据集 X_train_c = train.drop(['ID', 'CLASS'], axis=1).values y_train_c = train['CLASS'].values X_test_c = test.drop(['ID'], axis=1).values nfold = 5 kf = KFold(n_splits=nfold, shuffle=True, random_state=2020) prediction1 = np.zeros((len(X_test_c),)) i = 0 for train_index, valid_index in kf.split(X_train_c, y_train_c): print("\nFold {}".format(i + 1)) X_train, label_train = X_train_c[train_index], y_train_c[train_index] X_valid, label_valid = X_train_c[valid_index], y_train_c[valid_index] clf = SVR(kernel='rbf', C=1, gamma='scale') clf.fit(X_train, label_train) x1 = clf.predict(X_valid) y1 = clf.predict(X_test_c) prediction1 += y1 / nfold i += 1 result1 = np.round(prediction1) id_ = range(210, 314) df = pd.DataFrame({'ID': id_, 'CLASS': result1}) df.to_csv("baseline.csv", index=False)
这段代码是一个基线模型,使用了支持向量回归(SVR)算法,通过 K 折交叉验证来训练模型,并对测试集进行预测。其中,训练数据集和测试数据集均为 CSV 格式的文件,需要使用 pandas 库读取。具体的实现过程如下:
1. 读取训练集和测试集的数据,并进行预处理。
```python
train = pd.read_csv('train.csv')
test = pd.read_csv('test.csv')
X_train_c = train.drop(['ID', 'CLASS'], axis=1).values
y_train_c = train['CLASS'].values
X_test_c = test.drop(['ID'], axis=1).values
```
2. 定义 K 折交叉验证器,并进行模型训练和预测。
```python
nfold = 5
kf = KFold(n_splits=nfold, shuffle=True, random_state=2020)
prediction1 = np.zeros((len(X_test_c),))
i = 0
for train_index, valid_index in kf.split(X_train_c, y_train_c):
print("\nFold {}".format(i + 1))
X_train, label_train = X_train_c[train_index], y_train_c[train_index]
X_valid, label_valid = X_train_c[valid_index], y_train_c[valid_index]
clf = SVR(kernel='rbf', C=1, gamma='scale')
clf.fit(X_train, label_train)
x1 = clf.predict(X_valid)
y1 = clf.predict(X_test_c)
prediction1 += y1 / nfold
i += 1
```
3. 对测试集的预测结果进行处理,并将结果保存到 CSV 文件中。
```python
result1 = np.round(prediction1)
id_ = range(210, 314)
df = pd.DataFrame({'ID': id_, 'CLASS': result1})
df.to_csv("baseline.csv", index=False)
```
其中,`prediction1` 是对测试集的预测结果进行累加的数组,`result1` 是将累加结果四舍五入后得到的最终预测结果。最后将结果保存到 CSV 文件中,方便后续的提交。
def cv_model(clf, train_x, train_y, test_x, clf_name='lgb'): folds = 5 seed = 2021 kf = KFold(n_splits=folds, shuffle=True, random_state=seed) train = np.zeros(train_x.shape[0]) test = np.zeros(test_x.shape[0]) cv_scores = [] for i, (train_index, valid_index) in enumerate(kf.split(train_x, train_y)): print('************ {} *************'.format(str(i+1))) trn_x, trn_y, val_x, val_y = train_x.iloc[train_index], train_y[train_index], train_x.iloc[valid_index], train_y[valid_index] train_matrix = clf.Dataset(trn_x, label=trn_y) valid_matrix = clf.Dataset(val_x, label=val_y) params = { 'boosting_type': 'gbdt', 'objective': 'binary', 'metric': 'auc', 'min_child_weight': 5, 'num_leaves': 2**6, 'lambda_l2': 10, 'feature_fraction': 0.9, 'bagging_fraction': 0.9, 'bagging_freq': 4, 'learning_rate': 0.01, 'seed': 2021, 'nthread': 28, 'n_jobs':-1, 'silent': True, 'verbose': -1, } model = clf.train(params, train_matrix, 50000, valid_sets=[train_matrix, valid_matrix], #categorical_feature = categorical_feature, verbose_eval=500,early_stopping_rounds=200) val_pred = model.predict(val_x, num_iteration=model.best_iteration) test_pred = model.predict(test_x, num_iteration=model.best_iteration) train[valid_index] = val_pred test += test_pred / kf.n_splits cv_scores.append(roc_auc_score(val_y, val_pred)) print(cv_scores) print("%s_scotrainre_list:" % clf_name, cv_scores) print("%s_score_mean:" % clf_name, np.mean(cv_scores)) print("%s_score_std:" % clf_name, np.std(cv_scores)) return train, test lgb_train, lgb_test = cv_model(lgb, x_train, y_train, x_test)这段代码什么意思,分类标签为0和1,属于二分类,预测结果点击率的数值是怎么来的
这段代码实现了一个基于 LightGBM 模型的交叉验证方法,其中:
- `clf` 表示 LightGBM 的分类器。
- `train_x` 和 `train_y` 分别表示训练集的特征和标签。
- `test_x` 表示测试集的特征。
- `clf_name` 表示分类器的名称,用于输出结果时的标识。
具体流程如下:
1. 将训练集分成五份,每份作为一次验证集,其余作为训练集,进行五次交叉验证。
2. 定义 LightGBM 模型的参数。
3. 对每一份验证集进行训练,得到模型。
4. 对验证集和测试集进行预测,得到预测结果。
5. 将五次交叉验证的预测结果进行平均,作为最终的预测结果。
6. 输出交叉验证的 AUC 分数,作为模型的评价指标。
在这个代码中,分类标签为 0 和 1,属于二分类问题。预测结果点击率的数值是通过模型预测得到的,其大小表示样本被预测为正例的概率,也就是点击率的估计值。
相关推荐














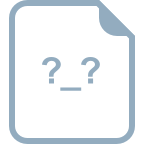
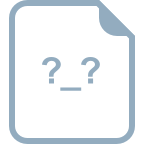