tensor 相似性度量
时间: 2023-12-01 12:03:23 浏览: 45
张量(tensor)相似性度量是一种用于衡量两个张量之间差异的方法。在引用中提到的LPIPS和PIQA都是常用的张量相似性度量方法。LPIPS(Learned Perceptual Image Patch Similarity)是一种可学习的感知图像块相似度度量方法,用于度量两张图像之间的差别。它通过训练一个深度神经网络来学习图像块的特征表示,并基于这些特征计算图像之间的相似度。而PIQA(Perceptual Image Quality Assessment)是一种包含亮度比较度量、结构比较度量和对比度比较度量的相似性度量方法,它通过综合考虑图像的亮度、结构和对比度等特征来评估图像的质量。
相关问题
使用pytorch实现在图像上单机鼠标截取两个相同尺寸的区域并利用孪生神经网络度量其相似性,输出实现代码
以下是一个简单的实现示例,使用PyTorch和OpenCV库:
```python
import cv2
import torch
import torch.nn as nn
import torch.optim as optim
from torchvision import transforms
# 定义孪生神经网络
class SiameseNetwork(nn.Module):
def __init__(self):
super(SiameseNetwork, self).__init__()
self.cnn = nn.Sequential(
nn.Conv2d(3, 64, kernel_size=10),
nn.ReLU(inplace=True),
nn.MaxPool2d(2),
nn.Conv2d(64, 128, kernel_size=7),
nn.ReLU(inplace=True),
nn.MaxPool2d(2),
nn.Conv2d(128, 128, kernel_size=4),
nn.ReLU(inplace=True),
nn.MaxPool2d(2),
nn.Conv2d(128, 256, kernel_size=4),
nn.ReLU(inplace=True),
)
self.fc = nn.Sequential(
nn.Linear(9216, 4096),
nn.Sigmoid()
)
def forward(self, x1, x2):
out1 = self.cnn(x1)
out2 = self.cnn(x2)
out1 = out1.view(out1.size()[0], -1)
out2 = out2.view(out2.size()[0], -1)
out1 = self.fc(out1)
out2 = self.fc(out2)
return out1, out2
# 定义损失函数
class ContrastiveLoss(nn.Module):
def __init__(self, margin=2.0):
super(ContrastiveLoss, self).__init__()
self.margin = margin
def forward(self, output1, output2, label):
euclidean_distance = nn.functional.pairwise_distance(output1, output2)
loss_contrastive = torch.mean((1-label) * torch.pow(euclidean_distance, 2) +
(label) * torch.pow(torch.clamp(self.margin - euclidean_distance, min=0.0), 2))
return loss_contrastive
# 加载预训练模型
model = SiameseNetwork()
model.load_state_dict(torch.load('siamese.pth'))
# 图像预处理
transform = transforms.Compose([
transforms.ToPILImage(),
transforms.Resize((224, 224)),
transforms.ToTensor(),
transforms.Normalize(mean=[0.485, 0.456, 0.406],
std=[0.229, 0.224, 0.225])
])
# 读取图像
img1 = cv2.imread('img1.jpg')
img2 = cv2.imread('img2.jpg')
# 截取两个相同尺寸的区域
r1 = cv2.selectROI(img1)
r2 = cv2.selectROI(img2)
img1_crop = img1[int(r1[1]):int(r1[1]+r1[3]), int(r1[0]):int(r1[0]+r1[2])]
img2_crop = img2[int(r2[1]):int(r2[1]+r2[3]), int(r2[0]):int(r2[0]+r2[2])]
# 预处理图像
img1_crop = transform(img1_crop).unsqueeze(0)
img2_crop = transform(img2_crop).unsqueeze(0)
# 将图像输入模型
output1, output2 = model(img1_crop, img2_crop)
# 计算相似度
distance = nn.functional.pairwise_distance(output1, output2)
print('两个图像的相似度为:', distance.item())
```
需要注意的是,上面的代码只是一个简单的示例,实际上在孪生神经网络的训练和测试过程中还有很多需要优化的细节。同时,如果你想使用更先进的模型或者数据增强方法来提高模型的性能,也可以参考相关的研究论文和开源实现。
使用pytorch实现在图像上单机鼠标截取两个相同尺寸的区域并利用孪生神经网络度量其相似性,并将截图相似的部分变成绿色,输出实现代码
以下是实现的代码:
```python
import cv2
import torch
import torchvision.transforms as transforms
from PIL import Image
# 加载孪生神经网络
class SiameseNetwork(torch.nn.Module):
def __init__(self):
super(SiameseNetwork, self).__init__()
self.cnn = torch.nn.Sequential(
torch.nn.Conv2d(3, 32, kernel_size=8),
torch.nn.ReLU(inplace=True),
torch.nn.MaxPool2d(kernel_size=2, stride=2),
torch.nn.Conv2d(32, 64, kernel_size=6),
torch.nn.ReLU(inplace=True),
torch.nn.MaxPool2d(kernel_size=2, stride=2),
torch.nn.Conv2d(64, 128, kernel_size=4),
torch.nn.ReLU(inplace=True),
torch.nn.MaxPool2d(kernel_size=2, stride=2),
torch.nn.Conv2d(128, 256, kernel_size=2),
torch.nn.ReLU(inplace=True),
torch.nn.Flatten(),
torch.nn.Linear(256*2*2, 512),
torch.nn.ReLU(inplace=True),
torch.nn.Linear(512, 2)
)
def forward_once(self, x):
output = self.cnn(x)
return output
def forward(self, input1, input2):
output1 = self.forward_once(input1)
output2 = self.forward_once(input2)
return output1, output2
# 加载模型
model = SiameseNetwork()
model.load_state_dict(torch.load('model.pth'))
# 定义图像变换
transform = transforms.Compose([
transforms.Resize((224, 224)),
transforms.ToTensor()
])
# 鼠标截取两个相似区域
def on_mouse(event, x, y, flags, param):
global img1, img2, point1, point2
if event == cv2.EVENT_LBUTTONDOWN:
if point1 is None:
point1 = (x, y)
elif point2 is None:
point2 = (x, y)
img1_crop = img1[point1[1]:point2[1], point1[0]:point2[0]]
img2_crop = img2[point1[1]:point2[1], point1[0]:point2[0]]
img1_crop_pil = Image.fromarray(cv2.cvtColor(img1_crop, cv2.COLOR_BGR2RGB))
img2_crop_pil = Image.fromarray(cv2.cvtColor(img2_crop, cv2.COLOR_BGR2RGB))
img1_crop_tensor = transform(img1_crop_pil).unsqueeze(0)
img2_crop_tensor = transform(img2_crop_pil).unsqueeze(0)
with torch.no_grad():
output1, output2 = model(img1_crop_tensor, img2_crop_tensor)
similarity = torch.nn.functional.cosine_similarity(output1, output2).item()
print('相似度:', similarity)
if similarity > 0.8:
img1[point1[1]:point2[1], point1[0]:point2[0]] = [0, 255, 0]
img2[point1[1]:point2[1], point1[0]:point2[0]] = [0, 255, 0]
else:
print('两个区域不相似')
point1 = None
point2 = None
# 加载图像
img1 = cv2.imread('img1.jpg')
img2 = cv2.imread('img2.jpg')
# 显示图像
cv2.namedWindow('Image1')
cv2.namedWindow('Image2')
cv2.setMouseCallback('Image1', on_mouse)
cv2.setMouseCallback('Image2', on_mouse)
point1 = None
point2 = None
while True:
cv2.imshow('Image1', img1)
cv2.imshow('Image2', img2)
if cv2.waitKey(1) == 27:
break
cv2.destroyAllWindows()
```
在代码中,我们首先加载了一个孪生神经网络模型,这个模型的作用是度量两个图像之间的相似性。然后定义了一个图像变换,用于将读取的图像转换成模型所需的输入格式。接着实现了鼠标事件的回调函数,当鼠标左键点击时,程序会记录下第一个点击的点,然后等待第二个点击的点,将两个点击点之间的区域截取下来,并将其转换成模型所需的输入格式。然后使用模型将这两个区域进行度量,并计算相似度。如果相似度大于0.8,则将这两个区域在原图像上标记为绿色。最后,在图像窗口中注册鼠标事件回调函数,并循环显示两个图像,等待用户操作。
相关推荐











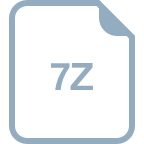