pytorch 胶囊网络
时间: 2023-11-10 07:03:07 浏览: 52
PyTorch胶囊网络是一种深度学习模型,用于解决图像识别和计算机视觉任务。它是由Hinton等人于2017年提出的,旨在克服传统卷积神经网络(CNN)的一些限制。
胶囊网络引入了胶囊层这一新概念,以替代CNN中的池化层和全连接层。胶囊层由许多小的胶囊组成,每个胶囊代表一个特定的实例,如一个特征或一个对象的部分。胶囊使用向量编码来表示实例的特征,而不仅仅是标量值。因此,胶囊网络具有丰富的表达能力和良好的泛化性能。
胶囊网络的工作原理是通过动态路由算法来学习特征之间的空间关系。它通过在胶囊层之间传递向量以进行信息交换,从而计算预测对象的姿态和存在概率。这种动态路由机制使得胶囊网络可以检测更复杂的模式和对象,比传统CNN更具准确性和鲁棒性。
PyTorch胶囊网络的特点包括:
1. 自然地处理空间关系:胶囊网络通过向量编码处理图像中的部分和对象,可以自然地捕捉它们之间的空间关系。
2. 减少信息丢失:相较于传统CNN,胶囊层使用向量编码,可以减少信息丢失,提高模型的鲁棒性。
3. 强大的泛化性能:胶囊网络的动态路由机制使得模型可以学习对象的不同姿态和变化,具有更强大的泛化性能。
4. 可解释性:胶囊网络对于学习到的特征和姿态具有可解释性,可以帮助我们更好地理解模型的决策过程。
总之,PyTorch胶囊网络是一种创新的深度学习模型,通过引入胶囊层和动态路由机制,可以提高图像识别和计算机视觉任务的准确性和鲁棒性。
相关问题
胶囊网络 pytorch
胶囊网络是一种基于神经网络的模型,其目标是通过学习代表物体姿态和特征的向量(胶囊)来改进传统的卷积神经网络(CNN)在识别物体时的性能。胶囊网络能够捕捉物体的空间关系和姿态信息,并且对变形和遮挡等情况具有更好的鲁棒性。
在PyTorch中,可以使用胶囊网络进行实现。通过引用的资料和,我们可以了解到如何在PyTorch中实现胶囊网络。首先,需要导入PyTorch相关的包,如`torch`和`torch.nn`等。然后,可以定义一个继承自`nn.Module`的胶囊网络模型类,并将相关的胶囊层、激活函数等组件添加到模型中。
在使用胶囊网络时,可以使用相应的数据集进行训练和测试。可以使用PyTorch提供的`torchvision`模块加载数据集,并使用`torch.nn`模块中的损失函数和优化器进行训练。可以根据需要进行数据预处理和数据增强等操作,以提高模型的性能。
同时,胶囊网络的论文中提到了一些关于胶囊的概念和理解,可以参考引用中的内容来进一步了解胶囊的概念。
最后,可以使用`matplotlib`等工具来可视化模型的训练过程和结果,并根据需要保存和加载训练好的模型参数。可以参考引用中的代码来实现这些功能。
总之,胶囊网络是一种改进传统卷积神经网络的模型,并且在PyTorch中可以使用相应的包和代码来实现。<span class="em">1</span><span class="em">2</span><span class="em">3</span><span class="em">4</span>
胶囊网络 pytorch实现
Pytorch是一种基于Python的科学计算包,它主要针对深度学习及自然语言处理任务。胶囊网络是一种新型的神经网络,它试图优化传统的卷积神经网络中存在的缺陷,能够更好地进行图像分类、目标检测、姿态估计等任务。
Pytorch实现的胶囊网络主要基于python语言进行编程,使用PyTorch框架搭建起来比较容易。具体来说,PyTorch提供了一些重要的工具来实现胶囊网络,其中包括自动微分、优化器和各种计算功能。
建立胶囊网络主要分为三个步骤。首先,需要定义网络的结构,包括胶囊层和路由算法等。其次,利用PyTorch的自动微分,对整个网络进行反向求导。最后,通过优化器对网络进行训练,使得其能够最优地完成某些任务。
Pytorch实现的胶囊网络具有许多优点,比如高效的计算、灵活的网络结构、强大的数据处理和可视化等。这些优点使得基于PyTorch的胶囊网络具有很广泛的应用前景,并且也为研究胶囊网络的性能和效果提供了良好的平台。
相关推荐
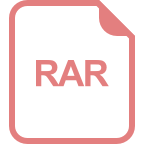
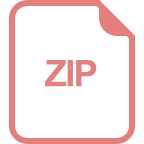
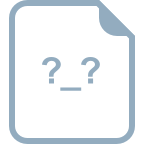












