生成Alexnet的网络图
时间: 2024-01-31 10:08:54 浏览: 24
为了生成AlexNet网络图,我们可以使用Graphviz这个工具。Graphviz是一个开源的可视化工具,可以将文本文件转换为图形。我们可以使用Python的graphviz库来生成AlexNet的网络图。以下是生成AlexNet网络图的步骤:
1. 安装graphviz库和pydotplus库
```shell
pip install graphviz
pip install pydotplus
```
2. 导入必要的库
```python
import graphviz
import torch
import torch.nn as nn
import torchvision.models as models
from torch.autograd import Variable
from graphviz import Digraph
import numpy as np
```
3. 定义AlexNet网络
```python
class AlexNet(nn.Module):
def __init__(self, num_classes=1000):
super(AlexNet, self).__init__()
self.features = nn.Sequential(
nn.Conv2d(3, 64, kernel_size=11, stride=4, padding=2),
nn.ReLU(inplace=True),
nn.MaxPool2d(kernel_size=3, stride=2),
nn.Conv2d(64, 192, kernel_size=5, padding=2),
nn.ReLU(inplace=True),
nn.MaxPool2d(kernel_size=3, stride=2),
nn.Conv2d(192, 384, kernel_size=3, padding=1),
nn.ReLU(inplace=True),
nn.Conv2d(384, 256, kernel_size=3, padding=1),
nn.ReLU(inplace=True),
nn.Conv2d(256, 256, kernel_size=3, padding=1),
nn.ReLU(inplace=True),
nn.MaxPool2d(kernel_size=3, stride=2),
)
self.avgpool = nn.AdaptiveAvgPool2d((6, 6))
self.classifier = nn.Sequential(
nn.Dropout(),
nn.Linear(256 * 6 * 6, 4096),
nn.ReLU(inplace=True),
nn.Dropout(),
nn.Linear(4096, 4096),
nn.ReLU(inplace=True),
nn.Linear(4096, num_classes),
)
def forward(self, x):
x = self.features(x)
x = self.avgpool(x)
x = x.view(x.size(0), 256 * 6 * 6)
x = self.classifier(x)
return x
```
4. 定义一个函数来生成网络图
```python
def make_dot(var, params=None):
""" Produces Graphviz representation of PyTorch autograd graph
Blue nodes are the Variables that require grad, orange are Tensors
saved for backward in torch.autograd.Function
Args:
var: output Variable
params: dict of (name, Variable) to add names to node that
require grad (TODO: make optional)
"""
if params is not None:
# 保存变量名
param_map = {id(v): k for k, v in params.items()}
node_attr = dict(style='filled',
shape='box',
align='left',
fontsize='12',
ranksep='0.1',
height='0.2')
dot = Digraph(node_attr=node_attr, graph_attr=dict(size="12,12"))
seen = set()
def size_to_str(size):
return '('+(', ').join(['%d' % v for v in size])+')'
def add_nodes(var):
if var not in seen:
if torch.is_tensor(var):
dot.node(str(id(var)), size_to_str(var.size()), fillcolor='orange')
elif hasattr(var, 'variable'):
u = var.variable
name = param_map[id(u)] if params is not None else ''
node_name = '%s\n %s' % (name, size_to_str(u.size()))
dot.node(str(id(var)), node_name, fillcolor='lightblue')
else:
dot.node(str(id(var)), str(type(var).__name__))
seen.add(var)
if hasattr(var, 'next_functions'):
for u in var.next_functions:
if u[0] is not None:
dot.edge(str(id(u[0])), str(id(var)))
add_nodes(u[0])
if hasattr(var, 'saved_tensors'):
for t in var.saved_tensors:
dot.edge(str(id(t)), str(id(var)))
add_nodes(t)
add_nodes(var.grad_fn)
return dot
```
5. 生成网络图
```python
# 创建一个随机的输入张量
x = Variable(torch.randn(1, 3, 224, 224))
# 创建一个AlexNet模型
model = AlexNet()
# 生成网络图
y = model(x)
g = make_dot(y, params=dict(model.named_parameters()))
# 保存网络图
g.view()
```
运行以上代码后,将会生成一个AlexNet的网络图。你可以使用Graphviz的可视化工具来查看这个网络图。
相关推荐
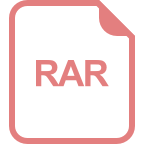
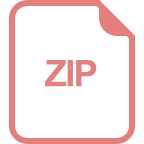
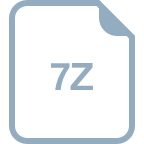
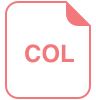


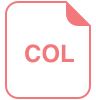
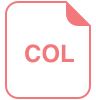









