If you wish to re-run the setup routine, please either move OR delete your previous base_conf file first.怎么解决
时间: 2024-11-10 19:25:25 浏览: 21
这句话的意思是在执行初始化设置时,如果你想要重新运行,首先需要对之前的基础配置文件(base_conf)采取操作,要么移动它到其他位置,要么直接删除。这是因为系统可能检测到了之前的配置文件存在,为了避免冲突或者覆盖已有的设置,你需要先处理掉这个文件。
要解决这个问题,你可以按照以下步骤操作:
1. **删除旧文件**:找到并删除 `base_conf` 文件,通常会在项目根目录、特定配置目录或者环境变量指定的路径下。
```sh
rm /path/to/your/base_conf
```
2. **移动旧文件**:如果希望保留配置文件但不想立即覆盖新的设置,可以将其移到别的地方,比如备份文件夹。
```sh
mv /path/to/your/base_conf /path/to/backup/
```
3. **重新运行setup**:现在旧文件已经移除或删除,你可以安全地重新运行初始化设置脚本了。
```sh
./setup.sh
```
记得在运行setup前确认新的base_conf文件是否已经准备就绪,并按照新脚本的要求进行配置。
相关问题
RET_DIAG DIAGAPP_RoutineControlReq( const stDiag_RoutineCtrlInfo* routinectrl_info, const stDiag_DataBodyArea* data_body ) { APL_MSGHDR msg_hdr = {0}; ST_MSGID_DIAGAPP_ROUTINE_CONTROL_REQ rctrl_req_info = {0}; if( routinectrl_info != NULL ){ msg_hdr.retblockID = 0; msg_hdr.resourceID = 0; msg_hdr.datasize = sizeof(rctrl_req_info); rctrl_req_info.routine_id = routinectrl_info->routine_id; rctrl_req_info.routinectrl_type = routinectrl_info->routinectrl_type; if( (data_body != NULL) && (data_body->data_len <= DIAG_DATA_MAX_SIZE) ){ if(data_body->data_len >= BYTE2) { rctrl_req_info.routine_data.data_len = (data_body->data_len - BYTE2); } else { rctrl_req_info.routine_data.data_len = 0; } ( void )FSIF_memcpy( rctrl_req_info.routine_data.data, &data_body->data_body[BYTE2], data_body->data_len ); /* Routine ID分を考慮して2Byte分マイナス */ } APP_DEBUG_LOG( APP_LOG_DEBUG1, "[DiagApp] <SndMsg> MSGID_SH_ROUTINE_CONTROL_REQ" ) /* ルーチンコントロール要求を送信 */ FRM_apl_sndmsg(MSGID_SH_ROUTINE_CONTROL_REQ, BLKID_APL_DIAGAPP, &msg_hdr, &rctrl_req_info); } return RET_DIAG_NORMAL; }
这段代码是一个名为 `DIAGAPP_RoutineControlReq` 的函数,用于发送诊断应用程序的路由控制请求。
函数接受两个参数,`routinectrl_info` 和 `data_body`,分别是类型为 `stDiag_RoutineCtrlInfo` 和 `stDiag_DataBodyArea` 的结构体指针。
以下是代码的逻辑解释:
1. 首先,代码初始化了 `msg_hdr` 和 `rctrl_req_info` 两个变量。
2. 如果 `routinectrl_info` 不为 NULL,则设置 `msg_hdr.retblockID`、`msg_hdr.resourceID` 和 `msg_hdr.datasize` 的值,并将 `routinectrl_info` 中的字段赋值给 `rctrl_req_info` 中相应的字段。
3. 接下来,代码检查 `data_body` 是否不为 NULL,并且 `data_body->data_len` 是否小于等于 `DIAG_DATA_MAX_SIZE`。如果满足条件,则进入内部的条件语句块。
4. 在条件语句块中,根据 `data_body->data_len` 的大小来设置 `rctrl_req_info.routine_data.data_len` 的值。如果 `data_body->data_len` 大于等于 `BYTE2`(一个常量值),则将其减去 `BYTE2` 并赋值给 `rctrl_req_info.routine_data.data_len`,否则将其设置为 0。
5. 然后,使用 `FSIF_memcpy` 函数将 `data_body->data_body[BYTE2]` 中的数据复制到 `rctrl_req_info.routine_data.data` 中。这里使用了 `data_body->data_len` 作为复制的长度。
6. 最后,函数发送一个日志消息,并通过调用 `FRM_apl_sndmsg` 函数发送 `MSGID_SH_ROUTINE_CONTROL_REQ` 消息,其中包含了 `msg_hdr` 和 `rctrl_req_info`。
7. 函数返回 `RET_DIAG_NORMAL`。
希望这个解释对您有帮助!如果您还有其他问题,请随时提问。
以下代码有什么问题def cal_total_distance(routine): '''The objective function. input routine, return total distance. cal_total_distance(np.arange(num_points)) ''' num_points, = routine.shape return sum([distance_matrix[routine[i % num_points], routine[(i + 1) % num_points]] for i in range(num_points)]) # 蚁群算法 from sko.ACA import ACA_TSP aca = ACA_TSP(func=cal_total_distance, n_dim=num_points, size_pop=50, max_iter=200, distance_matrix=distance_matrix) best_x, best_y = aca.run() fig, ax = plt.subplots(1, 2) best_points_ = np.concatenate([best_x, [best_x[0]]]) best_points_coordinate = points_coordinate[best_points_, :] ax[0].plot(best_points_coordinate[:, 0], best_points_coordinate[:, 1], 'o-r') pd.DataFrame(aca.y_best_history).cummin().plot(ax=ax[1]) plt.show() print(best_points_) print(best_y)
这段代码有两个问题:
1. 在函数 `cal_total_distance` 中,变量 `num_points` 没有定义,应该在调用该函数之前定义该变量。
2. 在代码中缺少必要的库导入,如 `numpy` 和 `matplotlib` 库,需要在代码开头导入这些库。
下面是修改后的代码:
```python
import numpy as np
import matplotlib.pyplot as plt
import pandas as pd
def cal_total_distance(routine, distance_matrix, num_points):
'''The objective function. input routine, return total distance.
cal_total_distance(np.arange(num_points), distance_matrix, num_points)
'''
return sum([distance_matrix[routine[i % num_points], routine[(i + 1) % num_points]] for i in range(num_points)])
# 蚁群算法
from sko.ACA import ACA_TSP
num_points = 10
distance_matrix = np.random.rand(num_points, num_points)
aca = ACA_TSP(func=cal_total_distance, n_dim=num_points, distance_matrix=distance_matrix,
size_pop=50, max_iter=200)
best_x, best_y = aca.run()
fig, ax = plt.subplots(1, 2)
best_points_ = np.concatenate([best_x, [best_x[0]]])
best_points_coordinate = points_coordinate[best_points_, :]
ax[0].plot(best_points_coordinate[:, 0], best_points_coordinate[:, 1], 'o-r')
pd.DataFrame(aca.y_best_history).cummin().plot(ax=ax[1])
plt.show()
print(best_points_)
print(best_y)
```
阅读全文
相关推荐
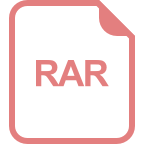
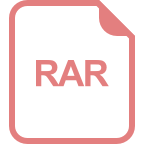
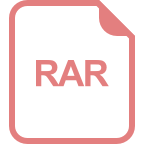
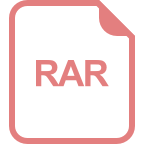
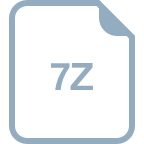
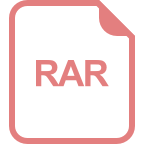
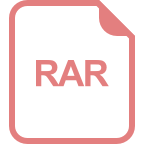
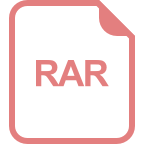
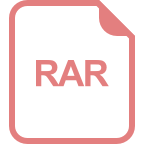
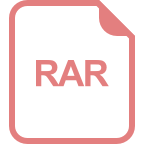
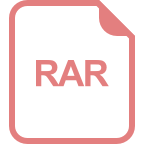
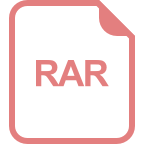
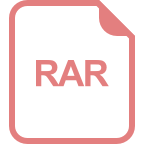
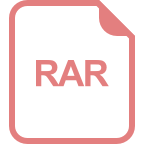


