%% 计算指标 INdex=[]; n=[]; for i=1:k A=NWP_cluster{i}; index=[]; for j=1:size(A,1) for x=1:size(A,2) index(j,x)=sum((A(j,:)-A(x,:)).^2)^0.5; end end INdex(k)=sum(sum(index))/(size(A,1)*size(A,2)-1)/2; n(k)=size(A,1)*size(A,2); end compactness=sum(INdex)/sum(n); disp(['紧致度为:',num2str(compactness)]) %% 找出原始不聚类的训练测试集 Label_test_first=[]; first_label=[]; Label_1=[L{1}' L{2}' L{3}']; for i=1:k Label=find(label==i); A=Label_1(find(label==i)); first_label{i}=Label(1+ceil(length(A)*5/6):end); A(1:ceil(length(A)*5/6))=[]; Label_test_first=[Label_test_first A]; end X=1:size(data,1); X(Label_test_first)=[]; Train_NWP_power_zhijie =[data(X,:) power_date(X,:)]; Test_NWP_power_zhijie =[data(Label_test_first,:) power_date(Label_test_first,:)]; csvwrite('不聚类的训练集.csv',Train_NWP_power_zhijie); csvwrite('不聚类的测试集.csv',Test_NWP_power_zhijie); %% 找出一重聚类结果的训练测试集 first_L1=[]; first_L2=[]; first_L3=[]; for i=1:k B=first_label{i}; L1_label=B(find(B<=length(L{1}))); L2_label=B(find(B<=length([L{1}' L{2}']))); L3_label=B(~ismember(B,L2_label)); L2_label=L2_label(~ismember(L2_label,L1_label)); first_L1=[first_L1;L1_label]; first_L2=[first_L2;L2_label]; first_L3=[first_L3;L3_label]; end first_cluster_test_1=Label_1(first_L1); first_cluster_test_2=Label_1(first_L2); first_cluster_test_3=Label_1(first_L3); first_cluster_train_1=Label_cluster{1}(~ismember(Label_cluster{1},first_cluster_test_1)); first_cluster_train_2=Label_cluster{2}(~ismember(Label_cluster{2},first_cluster_test_2)); first_cluster_train_3=Label_cluster{3}(~ismember(Label_cluster{3},first_cluster_test_3)); %% 划分出训练测试集 NWP_power_test_1=[data(first_cluster_test_1,:) power_date(first_cluster_test_1,:)]; NWP_power_test_2=[data(first_cluster_test_2,:) power_date(first_cluster_test_2,:)]; NWP_power_test_3=[data(first_cluster_test_3,:) power_date(first_cluster_test_3,:)]; NWP_power_train_1=[data(first_cluster_train_1,:) power_date(first_cluster_train_1,:)]; NWP_power_train_2=[data(first_cluster_train_2,:) power_date(first_cluster_train_2,:)]; NWP_power_train_3=[data(first_cluster_train_3,:) power_date(first_cluster_train_3,:)]; NWP_power_test=[{NWP_power_test_1} {NWP_power_test_2} {NWP_power_test_3}]; NWP_power_train=[{NWP_power_train_1} {NWP_power_train_2} {NWP_power_train_3}]; for i=1:k str_test=['NWP_power_test_',num2str(i),'.csv']; csvwrite(str_test,NWP_power_test{i}); str_train=['NWP_power_train_',num2str(i),'.csv']; csvwrite(str_train,NWP_power_train{i}); end
时间: 2024-04-19 13:26:48 浏览: 12
这部分代码是对聚类结果进行指标计算,并根据聚类结果将原始数据集划分为训练集和测试集。
首先,你计算了每个聚类类别的紧致度(compactness),通过计算样本之间的距离来衡量。然后,你将原始数据集中未被聚类的样本标签存储在`Label_test_first`中,并将剩余的样本作为不聚类的训练集和测试集,分别存储在`Train_NWP_power_zhijie`和`Test_NWP_power_zhijie`中。
接下来,你将一重聚类结果中每个类别的样本标签分别存储在`first_L1`、`first_L2`和`first_L3`中,并根据这些标签将一重聚类结果划分为训练集和测试集。训练集中的样本存储在`first_cluster_train_1`、`first_cluster_train_2`和`first_cluster_train_3`中,测试集中的样本存储在`first_cluster_test_1`、`first_cluster_test_2`和`first_cluster_test_3`中。
最后,你根据训练集和测试集的标签将数据集划分为不同的类别,并将每个类别的数据分别存储在`NWP_power_train`和`NWP_power_test`中,并将它们分别写入名为`NWP_power_train_1.csv`、`NWP_power_train_2.csv`、`NWP_power_train_3.csv`、`NWP_power_test_1.csv`、`NWP_power_test_2.csv`和`NWP_power_test_3.csv`的CSV文件中。
相关问题
data00=data m,n = np.shape(data00) a = np.array(data00) Data00 = a[1:m,2:n] Data00 = Data00.astype(np.float64) Power = Data00[:,13] Power_train = Power[0:96] P_min = np.min(Power_train) P_gap = np.max(Power_train)-np.min(Power_train) Power_uni = (Power-P_min)/P_gap # 提取imfs和剩余信号res emd = EMD() emd.emd(Power_uni) imfs, res = emd.get_imfs_and_residue() N = len(imfs) P_H = np.sum(imfs[0:6,:],axis=0) P_M = np.sum(imfs[6:12,:],axis=0) P_L = res P_H =np.expand_dims(P_H,axis=1) P_M =np.expand_dims(P_M,axis=1) P_L =np.expand_dims(P_L,axis=1) Nwp = Data00[:,0:7] Nwp_train = Nwp[0:96] N_min = np.min(Nwp_train,axis=0) N_gap = np.max(Nwp_train,axis=0)-np.min(Nwp_train,axis=0) Nwp_uni = (Nwp-N_min)/N_gap#(N,7) Weather = Data00[:,7:13] Weather_train = Weather[0:96] W_min = np.min(Weather_train,axis=0) W_gap = np.max(Weather_train,axis=0)-np.min(Weather_train,axis=0) Weather_uni = (Weather-W_min)/W_gap#(N,6) 优化代码
以下是部分代码的优化建议:
1. 对于以下代码段:
```
a = np.array(data00)
Data00 = a[1:m,2:n]
```
可以合并为一行:
```
Data00 = np.array(data00)[1:m,2:n]
```
2. 对于以下代码段:
```
P_H = np.sum(imfs[0:6,:],axis=0)
P_M = np.sum(imfs[6:12,:],axis=0)
P_L = res
P_H =np.expand_dims(P_H,axis=1)
P_M =np.expand_dims(P_M,axis=1)
P_L =np.expand_dims(P_L,axis=1)
```
可以使用 `np.newaxis` 替代 `np.expand_dims` 来实现:
```
P_H = np.sum(imfs[0:6,:],axis=0)[:, np.newaxis]
P_M = np.sum(imfs[6:12,:],axis=0)[:, np.newaxis]
P_L = res[:, np.newaxis]
```
3. 对于以下代码段:
```
N_min = np.min(Nwp_train,axis=0)
N_gap = np.max(Nwp_train,axis=0)-np.min(Nwp_train,axis=0)
Nwp_uni = (Nwp-N_min)/N_gap#(N,7)
```
可以使用 `np.ptp` 函数(peak-to-peak)来计算最大值和最小值的差:
```
N_min = np.min(Nwp_train,axis=0)
N_gap = np.ptp(Nwp_train,axis=0)
Nwp_uni = (Nwp-N_min)/N_gap#(N,7)
```
4. 对于以下代码段:
```
Weather = Data00[:,7:13]
Weather_train = Weather[0:96]
W_min = np.min(Weather_train,axis=0)
W_gap = np.max(Weather_train,axis=0)-np.min(Weather_train,axis=0)
Weather_uni = (Weather-W_min)/W_gap#(N,6)
```
可以使用与第三个优化建议类似的方法:
```
Weather = Data00[:,7:13]
Weather_train = Weather[0:96]
W_min = np.min(Weather_train,axis=0)
W_gap = np.ptp(Weather_train,axis=0)
Weather_uni = (Weather-W_min)/W_gap#(N,6)
```
当然,以上只是一些简单的优化建议,具体的优化效果还需要根据实际情况进行评估。
shap.dependence_plot('nwp4', shap_values, interaction_index=np(nwp4), show=False) TypeError: 'module' object is not callable
这个错误通常是因为你将 `np(nwp4)` 作为参数传递给了 `np` 模块,而 `np` 模块实际上是 NumPy 库,它不是可调用的函数。
你需要将 `np(nwp4)` 改为正确的值,例如:`np.array([0, 1, 2, 3])`。
如果你不确定正确的值是什么,可以尝试删除 `interaction_index` 参数,以便绘制非交互依赖图。即使用以下代码:
```python
shap.dependence_plot('nwp4', shap_values, show=False)
```
这将绘制与 `nwp4` 特征单独相关性的依赖图。希望这可以帮助你解决问题。
相关推荐
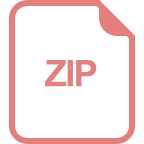









