C++ 中如何打印log至kernel log
时间: 2023-07-12 11:16:59 浏览: 85
在C++中,我们可以使用syslog(3)函数将日志打印到系统日志中,从而实现将日志输出至kernel log的目的。具体的步骤如下:
1. 在代码中包含syslog.h头文件。
2. 调用openlog函数打开日志。
3. 调用syslog函数将日志输出到系统日志中。
4. 调用closelog函数关闭日志。
例如,下面的代码将一条debug级别的日志打印到kernel log中:
```
#include <syslog.h>
int main() {
openlog("my_program", LOG_PID, LOG_USER);
syslog(LOG_DEBUG, "This is a debug log.");
closelog();
return 0;
}
```
注意需要root权限才能查看kernel log。
相关问题
c++怎样打印log
在C语言中,可以使用printf函数来打印log。printf函数是C语言标准库中的函数,可以将指定的字符串或变量的值输出到标准输出设备(例如控制台)。
要打印log,首先需要包含stdio.h头文件,该头文件中包含了printf函数的声明。然后,使用printf函数来输出需要打印的内容。具体的步骤如下:
1. 在代码文件的开头添加#include <stdio.h>语句,以包含stdio.h头文件。
2. 在需要打印log的位置使用printf函数。例如,要输出一个字符串,可以使用printf("log信息");要输出一个变量的值,可以使用printf("变量名:%d", 变量名)。
3. 在以上的printf语句中,%d是一个格式化占位符,用于指定要输出的变量的类型。例如,对于整数变量,可以使用%d;对于字符变量,可以使用%c等。
4. 如果需要在log中添加变量的值,可以使用格式化占位符。例如,printf("变量值为:%d", 变量名)可以将变量的值插入到相应的位置。
5. 可以使用转义字符(\n)来换行,以使打印的log更加清晰易读。
6. 最后,编译并运行代码,可以在控制台输出窗口中看到打印的log信息。
总之,在C语言中,使用printf函数可以将log信息输出到控制台,以便程序员在开发和调试过程中查看相关信息,帮助定位问题。
Android 中如何在C++代码中打印log到logcat中
在Android中,可以使用Android NDK提供的日志库来在C++代码中打印log到logcat中。以下是一个简单的示例代码:
```c++
#include <android/log.h>
#define LOG_TAG "MyNativeLib"
void myFunction() {
__android_log_print(ANDROID_LOG_DEBUG, LOG_TAG, "This is a debug message from C++");
__android_log_print(ANDROID_LOG_INFO, LOG_TAG, "This is an info message from C++");
__android_log_print(ANDROID_LOG_WARN, LOG_TAG, "This is a warning message from C++");
__android_log_print(ANDROID_LOG_ERROR, LOG_TAG, "This is an error message from C++");
}
```
在上面的示例代码中,`__android_log_print`函数的第一个参数是日志级别,第二个参数是日志标签,第三个参数是日志内容。可以使用以下几个日志级别:
- `ANDROID_LOG_DEBUG`:调试信息
- `ANDROID_LOG_INFO`:一般信息
- `ANDROID_LOG_WARN`:警告信息
- `ANDROID_LOG_ERROR`:错误信息
在代码中打印log后,可以在Android Studio中的logcat中查看输出的log。可以使用以下命令过滤出指定标签的log:
```shell
adb logcat -s MyNativeLib
```
这样就可以只显示MyNativeLib标签的log了。
相关推荐
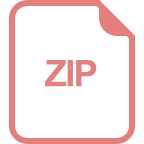
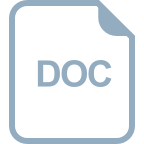












