sp1.set_smoothing_factor(_______) pl.plot(x, sp1(x), 'g', lw = 3)
时间: 2024-06-03 22:07:13 浏览: 34
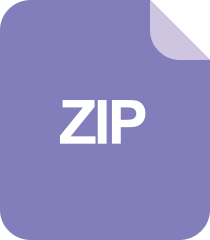
e.zip_smoothing filter_抑制噪声
The blank space in sp1.set_smoothing_factor(_______) should be filled with a numerical value that determines the level of smoothing applied to the data. A smaller value will result in less smoothing and a more jagged curve, while a larger value will result in more smoothing and a smoother curve. The specific value chosen will depend on the nature of the data and the desired level of smoothing.
阅读全文
相关推荐
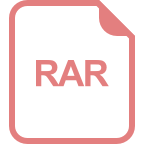
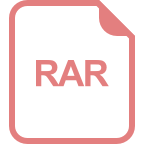



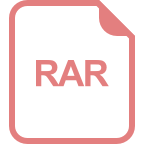
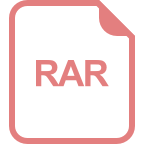
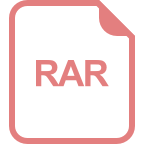



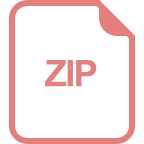