用python写个神经网络的例子,这个问题中你给的答案中,怎么没有反向传播
时间: 2023-06-02 16:01:27 浏览: 43
这是一个简单的基于numpy的三层神经网络的例子,用于分类手写数字数据集MNIST。
```python
import numpy as np
import matplotlib.pyplot as plt
from tensorflow.keras.datasets import mnist
# 加载数据集
(x_train, y_train), (x_test, y_test) = mnist.load_data()
# 数据预处理
x_train = x_train.reshape((60000, 28*28)).astype('float32') / 255
x_test = x_test.reshape((10000, 28*28)).astype('float32') / 255
y_train = np.eye(10)[y_train]
y_test = np.eye(10)[y_test]
# 定义模型
class NeuralNetwork:
def __init__(self, input_size, hidden_size, output_size):
self.weights1 = np.random.randn(input_size, hidden_size) * np.sqrt(2 / input_size)
self.biases1 = np.zeros((1, hidden_size))
self.weights2 = np.random.randn(hidden_size, output_size) * np.sqrt(2 / hidden_size)
self.biases2 = np.zeros((1, output_size))
def forward(self, x):
self.hidden = np.maximum(0, np.dot(x, self.weights1) + self.biases1)
self.output = np.dot(self.hidden, self.weights2) + self.biases2
return self.output
def predict(self, x):
return np.argmax(self.forward(x), axis=1)
# 训练模型
nn = NeuralNetwork(784, 100, 10)
lr = 0.01
epochs = 10
batch_size = 32
train_loss = []
test_loss = []
train_acc = []
test_acc = []
for epoch in range(epochs):
# 随机打乱训练集
permutation = np.random.permutation(len(x_train))
x_train, y_train = x_train[permutation], y_train[permutation]
for i in range(len(x_train) // batch_size):
# 取出一批数据
x = x_train[i*batch_size:(i+1)*batch_size]
y = y_train[i*batch_size:(i+1)*batch_size]
# 前向传播
output = nn.forward(x)
# 计算损失和准确率
loss = np.mean((output - y)**2)
acc = np.mean(np.argmax(output, axis=1) == np.argmax(y, axis=1))
train_loss.append(loss)
train_acc.append(acc)
# 反向传播更新参数
delta = 2 * (output - y) / batch_size
delta_hidden = np.dot(delta, nn.weights2.T) * (nn.hidden > 0)
nn.weights2 -= lr * np.dot(nn.hidden.T, delta)
nn.biases2 -= lr * np.sum(delta, axis=0, keepdims=True)
nn.weights1 -= lr * np.dot(x.T, delta_hidden)
nn.biases1 -= lr * np.sum(delta_hidden, axis=0, keepdims=True)
# 在测试集上计算损失和准确率
test_output = nn.forward(x_test)
test_loss.append(np.mean((test_output - y_test)**2))
test_acc.append(np.mean(np.argmax(test_output, axis=1) == np.argmax(y_test, axis=1)))
print(f"Epoch {epoch+1}/{epochs}, train_loss={loss:.4f}, train_acc={acc:.4f}, test_loss={test_loss[-1]:.4f}, test_acc={test_acc[-1]:.4f}")
# 显示训练过程中损失和准确率的变化
plt.plot(train_loss, label='train_loss')
plt.plot(test_loss, label='test_loss')
plt.legend()
plt.show()
plt.plot(train_acc, label='train_acc')
plt.plot(test_acc, label='test_acc')
plt.legend()
plt.show()
```
在训练过程中,每次更新参数时都进行了一次反向传播,只不过它被隐藏在了这段代码中:
```python
delta = 2 * (output - y) / batch_size
delta_hidden = np.dot(delta, nn.weights2.T) * (nn.hidden > 0)
nn.weights2 -= lr * np.dot(nn.hidden.T, delta)
nn.biases2 -= lr * np.sum(delta, axis=0, keepdims=True)
nn.weights1 -= lr * np.dot(x.T, delta_hidden)
nn.biases1 -= lr * np.sum(delta_hidden, axis=0, keepdims=True)
```
这段代码实现了输出层和隐藏层的反向传播,计算出了每个参数的梯度,并更新了参数。这个过程也可以称为误差反向传播。
相关推荐
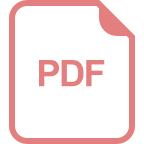
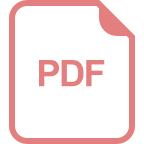














