用Python代码写一个神经网络代码
时间: 2024-05-10 10:15:21 浏览: 126
好的,以下是一个简单的神经网络的 Python 代码:
```python
import numpy as np
# 定义激活函数
def sigmoid(x):
return 1 / (1 + np.exp(-x))
# 初始化权重和偏置
def initialize_parameters(n_x, n_h, n_y):
W1 = np.random.randn(n_h, n_x) * 0.01
b1 = np.zeros((n_h, 1))
W2 = np.random.randn(n_y, n_h) * 0.01
b2 = np.zeros((n_y, 1))
parameters = {"W1": W1, "b1": b1, "W2": W2, "b2": b2}
return parameters
# 前向传播
def forward_propagation(X, parameters):
W1 = parameters["W1"]
b1 = parameters["b1"]
W2 = parameters["W2"]
b2 = parameters["b2"]
Z1 = np.dot(W1, X) + b1
A1 = sigmoid(Z1)
Z2 = np.dot(W2, A1) + b2
A2 = sigmoid(Z2)
cache = {"Z1": Z1, "A1": A1, "Z2": Z2, "A2": A2}
return A2, cache
# 计算损失函数
def compute_cost(A2, Y):
m = Y.shape[1]
cost = -np.sum(Y * np.log(A2) + (1 - Y) * np.log(1 - A2)) / m
cost = np.squeeze(cost)
return cost
# 反向传播
def backward_propagation(parameters, cache, X, Y):
m = X.shape[1]
W1 = parameters["W1"]
W2 = parameters["W2"]
A1 = cache["A1"]
A2 = cache["A2"]
Z1 = cache["Z1"]
dZ2 = A2 - Y
dW2 = np.dot(dZ2, A1.T) / m
db2 = np.sum(dZ2, axis=1, keepdims=True) / m
dZ1 = np.dot(W2.T, dZ2) * (1 - np.power(A1, 2))
dW1 = np.dot(dZ1, X.T) / m
db1 = np.sum(dZ1, axis=1, keepdims=True) / m
gradients = {"dW1": dW1, "db1": db1, "dW2": dW2, "db2": db2}
return gradients
# 更新参数
def update_parameters(parameters, gradients, learning_rate=1.2):
W1 = parameters["W1"]
b1 = parameters["b1"]
W2 = parameters["W2"]
b2 = parameters["b2"]
dW1 = gradients["dW1"]
db1 = gradients["db1"]
dW2 = gradients["dW2"]
db2 = gradients["db2"]
W1 = W1 - learning_rate * dW1
b1 = b1 - learning_rate * db1
W2 = W2 - learning_rate * dW2
b2 = b2 - learning_rate * db2
parameters = {"W1": W1, "b1": b1, "W2": W2, "b2": b2}
return parameters
# 模型训练
def nn_model(X, Y, n_h, num_iterations=10000, print_cost=False):
n_x = X.shape[0]
n_y = Y.shape[0]
parameters = initialize_parameters(n_x, n_h, n_y)
for i in range(num_iterations):
A2, cache = forward_propagation(X, parameters)
cost = compute_cost(A2, Y)
gradients = backward_propagation(parameters, cache, X, Y)
parameters = update_parameters(parameters, gradients)
if print_cost and i % 1000 == 0:
print("Cost after iteration {}: {}".format(i, cost))
return parameters
# 预测
def predict(parameters, X):
A2, cache = forward_propagation(X, parameters)
predictions = (A2 > 0.5)
return predictions
# 测试模型
X = np.array([[0, 0, 1, 1], [0, 1, 0, 1]])
Y = np.array([[0, 1, 1, 0]])
parameters = nn_model(X, Y, n_h=4, num_iterations=10000, print_cost=True)
predictions = predict(parameters, X)
print("Predictions: {}".format(predictions))
```
这个代码实现了一个简单的两层神经网络,并使用 XOR 逻辑门的例子进行了测试。
阅读全文
相关推荐
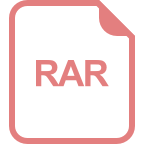
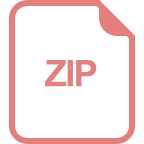
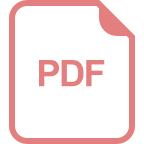















