深度卷积和普通卷积在python程序上的区别
时间: 2024-09-01 19:00:19 浏览: 25
深度卷积(Deep Convolution)和普通卷积(Standard Convolution)的主要区别在于其在网络结构和计算复杂性方面的应用。在Python程序中,它们的区别体现在以下几个方面:
1. **网络深度**:深度卷积通常用于深度学习神经网络(如卷积神经网络CNN),它包含多个卷积层,每一层都能提取图像的不同特征。这使得模型能够处理更复杂的图像识别和分析任务。
2. **参数共享**:深度卷积通过在每个卷积层共享权重参数,减少了模型的参数量,降低了过拟合的风险,并提高了效率。
3. **激活函数**:深度卷积常常与非线性激活函数(如ReLU、Leaky ReLU等)结合,增加了模型的表达能力。
4. **前向传播**:在实际Python代码中,例如用TensorFlow或PyTorch实现,深度卷积操作会在循环中进行多次,每次对输入数据做一次小的变换并累积结果。
5. **反向传播**:梯度下降优化时,深度卷积会计算整个网络路径的梯度,更新所有层级的权重。
相关问题
深度卷积和普通卷积区别
深度卷积(Deep Convolution)与普通的卷积(Standard Convolution)的主要区别在于它们在处理数据以及模型复杂性方面的特性:
1. **计算深度**:深度卷积是指在深度学习网络中包含多层卷积层的设计。每层卷积可以提取图像的不同特征,从低级边缘到高级抽象特征。而普通卷积通常只包含一两层。
2. **参数共享**:无论是深度还是普通卷积,都利用了参数共享的概念,即每个滤波器在整个输入上共享权重。但在深度卷积中,这种共享发生在整个网络的各个层级之间,使得模型能够学习更丰富的特征表示。
3. **非线性和池化**:深度卷积通常会跟随着激活函数(如ReLU)和池化操作,形成卷积神经网络(CNN)的基本模块。这有助于增强模型的学习能力和对局部特征的不变性。
4. **模型表达能力**:深度卷积通过增加网络层数提高了模型的表达能力,能够捕获更复杂的模式。而普通卷积在较浅的网络结构下可能会受限于其表征能力。
深度卷积和普通卷积的区别
深度卷积和普通卷积的区别在于它们的输入和输出。普通卷积只包含一个输入和一个输出,而深度卷积可以接受多个输入和输出。
在普通卷积中,卷积核只对一个通道的输入进行卷积操作,输出也只有一个通道。而在深度卷积中,卷积核可以同时对多个通道进行卷积操作,输出也可以有多个通道。
例如,一个普通卷积层可能接受一张 28x28 的灰度图像作为输入,并使用一个 3x3 的卷积核进行卷积操作,得到一张 26x26 的特征图。而一个深度卷积层可能接受一组大小为 28x28x3 的 RGB 图像作为输入,并使用多个 3x3 的卷积核进行卷积操作,得到一组大小为 26x26x64 的特征图。
深度卷积可以更好地捕捉输入数据中的多个特征,从而提高模型的性能。但是,由于深度卷积需要更多的计算资源和参数调整,因此在实践中需要谨慎使用。
相关推荐
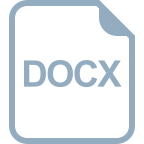
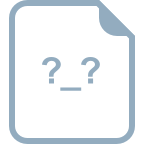
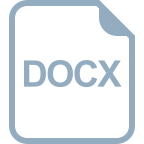












