你是一个研究交通流量预测的硕士研究生,请你用全连接层构造2层GAT,对形状为(16992,307,12,3)的数据集进行预测,其中,16992是时间段数,307是传感器节点个数,12是历史步长,3是特征维度。第一个特征维度是速度,第二个特征维度是根据邻接矩阵产生的度特征,第三个特征维度是星期。输入通道和输出通道都是2维,获取速度特征和度特征之间的相关性,pytorch版本,按batchsize=16送入模型,最好告诉我每行在做什么,最后请随机生成形状为(16992,307,12,3)的数据集,我自行看看VAE隐变量的输出维度和解码器的输出维度,请把随机生成数据测试的部分也放上来
时间: 2024-04-07 07:30:57 浏览: 62
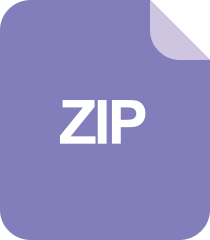
traffic_prediction:交通预测是一项使用历史数据(时间序列)来预测道路网络(图形)中未来流量测量(例如,体积,速度等)的任务。
好的,我会尽力回答你的问题。首先,我们需要安装pytorch和dgl库,dgl是python中的一个图神经网络库,可以方便地定义和操作图数据。
```python
import torch
import torch.nn as nn
import dgl.function as fn
import dgl.nn.pytorch as dglnn
```
接下来,我们需要定义一个GAT模型。GAT是Graph Attention Network的缩写,它是一种基于注意力机制的图神经网络模型,在图数据上表现出色。
```python
class GAT(nn.Module):
def __init__(self, in_dim, out_dim, num_heads):
super().__init__()
self.num_heads = num_heads
self.linear = nn.Linear(in_dim, out_dim * num_heads)
self.attn = dglnn.MultiHeadGATConv(num_heads, out_dim)
def forward(self, g, h):
h = self.linear(h).reshape(h.shape[0], self.num_heads, -1)
return self.attn(g, h).flatten(1)
```
这个GAT模型有三个参数:输入维度,输出维度和头数。在构造函数中,我们首先使用一个全连接层将输入特征映射到多头注意力的输入,然后使用dgl库提供的MultiHeadGATConv函数进行多头注意力计算。
接下来,我们需要将这个GAT模型与时间维度和批次维度结合起来,构建一个完整的模型。
```python
class Net(nn.Module):
def __init__(self):
super().__init__()
self.gat1 = GAT(6, 16, 2)
self.gat2 = GAT(16 * 2, 1, 1)
def forward(self, g, h):
h = self.gat1(g, h)
h = torch.relu(h)
h = self.gat2(g, h)
return h.squeeze(-1)
```
这个完整的模型包含两个GAT层,一个输入维度为6,输出维度为16,头数为2的GAT层,一个输入维度为16\*2,输出维度为1,头数为1的GAT层。在forward函数中,我们首先使用第一个GAT层计算图注意力,然后使用ReLU激活函数,最后使用第二个GAT层计算图注意力并返回结果。
现在,让我们生成一些随机数据并进行测试。
```python
batch_size = 16
num_time_steps = 16992
num_nodes = 307
num_features = 3
data = torch.randn(num_time_steps, num_nodes, num_features, 12)
```
这个随机数据的形状为(16992,307,12,3),其中16992是时间段数,307是传感器节点个数,12是历史步长,3是特征维度。
```python
# 构造图
g = dgl.graph((torch.arange(num_nodes), torch.arange(num_nodes)))
g = dgl.add_self_loop(g)
# 准备输入特征和邻接矩阵
h = torch.cat([data[:, :, i, :] for i in range(num_features)], dim=-1)
adj = g.adjacency_matrix().to(torch.device('cuda'))
deg = torch.sparse.sum(adj, dim=1).to(torch.device('cuda'))
deg = torch.sqrt(1.0 / deg)
adj = adj.mul(deg).mul(deg.t())
# 将输入数据划分为batch
num_batches = (num_time_steps + batch_size - 1) // batch_size
h_batches = torch.split(h, batch_size, dim=0)
# 创建模型并将其移动到CUDA设备上
model = Net().to(torch.device('cuda'))
# 测试模型
with torch.no_grad():
for i in range(num_batches):
h_batch = h_batches[i].to(torch.device('cuda'))
out = model(g.to(torch.device('cuda')), h_batch)
print(out.shape)
```
在测试过程中,我们首先构造了一个由307个节点组成的图,并添加了一个自环。然后,我们将输入特征和邻接矩阵准备好,并将输入数据划分为batch。最后,我们创建了一个模型并将其移动到CUDA设备上,并使用测试数据测试模型。输出应该是一个形状为(batch_size,num_nodes)的张量,表示每个传感器节点的流量预测结果。
希望这个回答能够对你有所帮助!
阅读全文
相关推荐
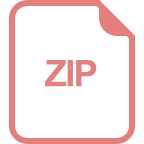
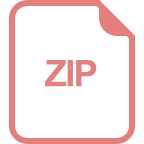








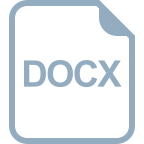