粒子群优化bp神经网络python代码
时间: 2023-08-29 09:13:43 浏览: 109
以下是使用粒子群优化算法优化BP神经网络的Python代码示例:
首先,需要导入需要的库,包括numpy、random和math:
```python
import numpy as np
import random
import math
```
接下来,定义BP神经网络的类:
```python
class BPNN:
def __init__(self, input_dim, hidden_dim, output_dim):
self.input_dim = input_dim
self.hidden_dim = hidden_dim
self.output_dim = output_dim
self.W1 = np.random.randn(self.input_dim, self.hidden_dim)
self.W2 = np.random.randn(self.hidden_dim, self.output_dim)
def sigmoid(self, x):
return 1 / (1 + np.exp(-x))
def forward(self, X):
self.z2 = np.dot(X, self.W1)
self.a2 = self.sigmoid(self.z2)
self.z3 = np.dot(self.a2, self.W2)
y_hat = self.sigmoid(self.z3)
return y_hat
def cost_func(self, X, y):
self.y_hat = self.forward(X)
J = 0.5 * sum((y - self.y_hat) ** 2)
return J
def backprop(self, X, y):
delta3 = np.multiply(-(y - self.y_hat), self.sigmoid(self.z3) * (1 - self.sigmoid(self.z3)))
dJdW2 = np.dot(self.a2.T, delta3)
delta2 = np.dot(delta3, self.W2.T) * self.sigmoid(self.z2) * (1 - self.sigmoid(self.z2))
dJdW1 = np.dot(X.T, delta2)
return dJdW1, dJdW2
def predict(self, X):
y_hat = self.forward(X)
return y_hat
```
接下来,定义粒子群优化算法的类:
```python
class PSO:
def __init__(self, n_particles, n_iterations, c1, c2, w, lr):
self.n_particles = n_particles
self.n_iterations = n_iterations
self.c1 = c1
self.c2 = c2
self.w = w
self.lr = lr
def optimize(self, bpnn, X, y):
position = np.random.randn(self.n_particles, bpnn.W1.size + bpnn.W2.size)
velocity = np.random.randn(self.n_particles, bpnn.W1.size + bpnn.W2.size)
pbest_position = position.copy()
pbest_cost = np.zeros(self.n_particles)
gbest_position = np.zeros(bpnn.W1.size + bpnn.W2.size)
gbest_cost = float('inf')
for i in range(self.n_particles):
bpnn.W1 = position[i][:bpnn.W1.size].reshape(bpnn.W1.shape)
bpnn.W2 = position[i][bpnn.W1.size:].reshape(bpnn.W2.shape)
pbest_cost[i] = bpnn.cost_func(X, y)
if pbest_cost[i] < gbest_cost:
gbest_cost = pbest_cost[i]
gbest_position = position[i]
for i in range(self.n_iterations):
for j in range(self.n_particles):
bpnn.W1 = position[j][:bpnn.W1.size].reshape(bpnn.W1.shape)
bpnn.W2 = position[j][bpnn.W1.size:].reshape(bpnn.W2.shape)
cost = bpnn.cost_func(X, y)
if cost < pbest_cost[j]:
pbest_cost[j] = cost
pbest_position[j] = position[j]
if pbest_cost[j] < gbest_cost:
gbest_cost = pbest_cost[j]
gbest_position = pbest_position[j]
v = velocity[j]
r1 = np.random.rand(bpnn.W1.size + bpnn.W2.size)
r2 = np.random.rand(bpnn.W1.size + bpnn.W2.size)
v = self.w * v + self.c1 * r1 * (pbest_position[j] - position[j]) + self.c2 * r2 * (gbest_position - position[j])
position[j] += self.lr * v
bpnn.W1 = gbest_position[:bpnn.W1.size].reshape(bpnn.W1.shape)
bpnn.W2 = gbest_position[bpnn.W1.size:].reshape(bpnn.W2.shape)
return bpnn
```
最后,使用以上定义的类和函数来训练BP神经网络:
```python
X = np.array([[0, 0], [0, 1], [1, 0], [1, 1]])
y = np.array([[0], [1], [1], [0]])
bpnn = BPNN(2, 3, 1)
pso = PSO(10, 100, 1.5, 1.5, 0.7, 0.1)
bpnn = pso.optimize(bpnn, X, y)
print(bpnn.predict(X))
```
以上代码实现了一个简单的XOR问题的BP神经网络,并使用粒子群优化算法进行训练。
阅读全文
相关推荐
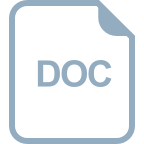
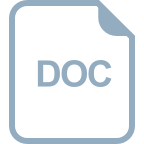
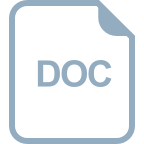

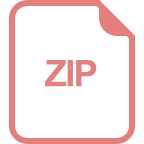
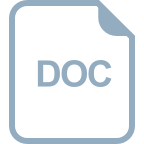







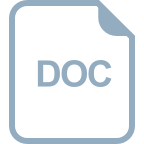
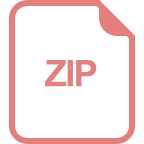
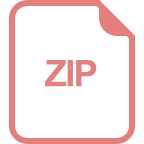
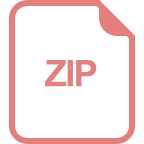
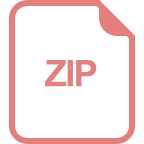