pycharm使用orb算法进行图像特征检测
时间: 2023-07-15 18:15:34 浏览: 127
首先,你需要安装OpenCV库来使用ORB算法。在PyCharm中,你可以通过以下命令安装OpenCV:
```
pip install opencv-python
```
接下来,你可以按照以下步骤使用ORB算法进行图像特征检测:
1. 导入必要的库:
```python
import cv2
import numpy as np
```
2. 读取图像:
```python
img = cv2.imread('image.jpg')
```
3. 创建ORB对象:
```python
orb = cv2.ORB_create()
```
4. 检测图像的关键点和描述符:
```python
keypoints, descriptors = orb.detectAndCompute(img, None)
```
5. 可以在图像上绘制关键点:
```python
img_with_keypoints = cv2.drawKeypoints(img, keypoints, None)
```
6. 展示结果:
```python
cv2.imshow('Image with keypoints', img_with_keypoints)
cv2.waitKey(0)
cv2.destroyAllWindows()
```
这些步骤将使用ORB算法检测图像中的特征点,并在图像上绘制出这些点。你可以对这些关键点进行进一步操作,例如用于图像匹配或目标跟踪。
相关问题
pycharm使用CANNY进行图像配准和拼接
在PyCharm中,您可以使用OpenCV库中的Canny算法来进行图像配准和拼接。以下是一些示例代码,可以帮助您开始:
```python
import cv2
# Load images
img1 = cv2.imread('image1.png')
img2 = cv2.imread('image2.png')
# Convert images to grayscale
gray1 = cv2.cvtColor(img1, cv2.COLOR_BGR2GRAY)
gray2 = cv2.cvtColor(img2, cv2.COLOR_BGR2GRAY)
# Detect edges using Canny algorithm
edges1 = cv2.Canny(gray1, 100, 200)
edges2 = cv2.Canny(gray2, 100, 200)
# Find keypoints and descriptors
orb = cv2.ORB_create()
kp1, des1 = orb.detectAndCompute(edges1, None)
kp2, des2 = orb.detectAndCompute(edges2, None)
# Match descriptors using Brute-Force matcher
bf = cv2.BFMatcher(cv2.NORM_HAMMING, crossCheck=True)
matches = bf.match(des1, des2)
# Sort matches by distance
matches = sorted(matches, key=lambda x: x.distance)
# Draw top matches on a new image
result = cv2.drawMatches(img1, kp1, img2, kp2, matches[:10], None, flags=2)
# Display result
cv2.imshow('Result', result)
cv2.waitKey(0)
```
这段代码会将两张图片转换为灰度图像,并使用Canny算法检测边缘。然后,它使用ORB算法检测关键点和描述符,并使用Brute-Force匹配器将它们匹配。最后,它会将匹配结果绘制在一张新的图片上,并显示出来。您可以根据需要调整参数,以获得更好的结果。
pycharm图像配准
在PyCharm中进行图像配准,需要使用相关的图像处理库,例如OpenCV和scikit-image。以下是一个简单的示例代码,演示了如何使用OpenCV实现基本的图像配准:
```python
import cv2
# 读取待配准的两幅图像
img1 = cv2.imread('image1.jpg')
img2 = cv2.imread('image2.jpg')
# 将图像转换为灰度图像
gray1 = cv2.cvtColor(img1, cv2.COLOR_BGR2GRAY)
gray2 = cv2.cvtColor(img2, cv2.COLOR_BGR2GRAY)
# 使用ORB算法提取关键点和描述符
orb = cv2.ORB_create()
keypoints1, descriptors1 = orb.detectAndCompute(gray1, None)
keypoints2, descriptors2 = orb.detectAndCompute(gray2, None)
# 使用BFMatcher算法匹配关键点
bf = cv2.BFMatcher(cv2.NORM_HAMMING, crossCheck=True)
matches = bf.match(descriptors1, descriptors2)
# 对匹配的关键点进行排序
matches = sorted(matches, key=lambda x: x.distance)
# 取前10个匹配点
src_pts = np.float32([keypoints1[m.queryIdx].pt for m in matches[:10]]).reshape(-1, 1, 2)
dst_pts = np.float32([keypoints2[m.trainIdx].pt for m in matches[:10]]).reshape(-1, 1, 2)
# 使用findHomography函数计算单应性矩阵
M, mask = cv2.findHomography(src_pts, dst_pts, cv2.RANSAC, 5.0)
# 使用warpPerspective函数将img1配准到img2
h, w = img1.shape[:2]
aligned = cv2.warpPerspective(img1, M, (w, h))
# 显示配准后的图像
cv2.imshow('Aligned Image', aligned)
cv2.waitKey(0)
cv2.destroyAllWindows()
```
在上面的示例中,我们首先读取待配准的两幅图像,然后将它们转换为灰度图像,并使用ORB算法提取它们的关键点和描述符。接着,我们使用BFMatcher算法匹配关键点,并根据匹配点计算单应性矩阵。最后,我们使用warpPerspective函数将img1配准到img2,并显示配准后的图像。
需要注意的是,图像配准是一个相对复杂的任务,需要根据具体情况选择合适的算法和参数。以上示例只是一个简单的演示,实际应用中需要根据具体情况进行调整和优化。
阅读全文
相关推荐
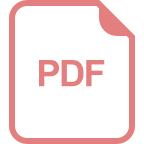
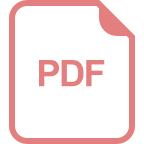
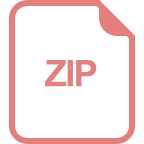
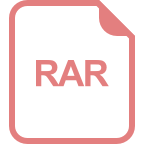
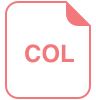
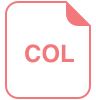
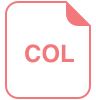
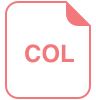
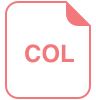
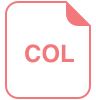
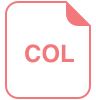
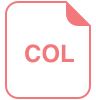
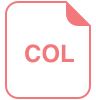
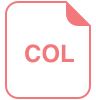
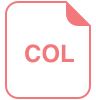
