net = nn.Sequential( nn.Conv2d(1, 6, kernel_size=5), BatchNorm(6, num_dims=4), nn.Sigmoid(), nn.AvgPool2d(kernel_size=2, stride=2), nn.Conv2d(6, 16, kernel_size=5), BatchNorm(16, num_dims=4), nn.Sigmoid(), nn.AvgPool2d(kernel_size=2, stride=2), nn.Flatten(), nn.Linear(16*4*4, 120), BatchNorm(120, num_dims=2), nn.Sigmoid(), nn.Linear(120, 84), BatchNorm(84, num_dims=2), nn.Sigmoid(), nn.Linear(84, 10)) # 和以前一样,再Fashin-MNIST数据集上训练网络,与6.6几乎完全相同,区别在于学习率大得多 lr, num_epochs, batch_size = 1.0, 10, 256 train_iter, test_iter = d2l.load_data_fashion_mnist(batch_size) d2l.train_ch6(net, train_iter, test_iter, num_epochs, lr, d2l.try_gpu()) d2l.plt.show() # 从第一个批量规范化层中学到的拉伸参数gamma和偏移参数beta print(net[1].gamma.reshape((-1,)), net[1].beta.reshape((-1,)))
时间: 2023-10-04 10:12:30 浏览: 63
这段代码定义了一个包含卷积层、批量规范化层、池化层和全连接层的神经网络,并使用 Fashion-MNIST 数据集对其进行训练。在训练后,代码打印了从第一个批量规范化层中学到的拉伸参数 gamma 和偏移参数 beta。
具体来说,`net[1]` 表示神经网络中的第二个层,即第一个批量规范化层。`net[1].gamma` 表示该层学到的拉伸参数,`net[1].beta` 表示该层学到的偏移参数。这两个参数的形状都是 `(6,)`,因为该批量规范化层有 6 个特征图。通过 `reshape((-1,))` 将参数的形状变成了 1 维,便于打印输出。
相关问题
import torch import torch.nn as nn import torch.nn.functional as F from torch.autograd import Variable class Bottleneck(nn.Module): def init(self, last_planes, in_planes, out_planes, dense_depth, stride, first_layer): super(Bottleneck, self).init() self.out_planes = out_planes self.dense_depth = dense_depth self.conv1 = nn.Conv2d(last_planes, in_planes, kernel_size=1, bias=False) self.bn1 = nn.BatchNorm2d(in_planes) self.conv2 = nn.Conv2d(in_planes, in_planes, kernel_size=3, stride=stride, padding=1, groups=32, bias=False) self.bn2 = nn.BatchNorm2d(in_planes) self.conv3 = nn.Conv2d(in_planes, out_planes+dense_depth, kernel_size=1, bias=False) self.bn3 = nn.BatchNorm2d(out_planes+dense_depth) self.shortcut = nn.Sequential() if first_layer: self.shortcut = nn.Sequential( nn.Conv2d(last_planes, out_planes+dense_depth, kernel_size=1, stride=stride, bias=False), nn.BatchNorm2d(out_planes+dense_depth) ) def forward(self, x): out = F.relu(self.bn1(self.conv1(x))) out = F.relu(self.bn2(self.conv2(out))) out = self.bn3(self.conv3(out)) x = self.shortcut(x) d = self.out_planes out = torch.cat([x[:,:d,:,:]+out[:,:d,:,:], x[:,d:,:,:], out[:,d:,:,:]], 1) out = F.relu(out) return out class DPN(nn.Module): def init(self, cfg): super(DPN, self).init() in_planes, out_planes = cfg['in_planes'], cfg['out_planes'] num_blocks, dense_depth = cfg['num_blocks'], cfg['dense_depth'] self.conv1 = nn.Conv2d(3, 64, kernel_size=3, stride=1, padding=1, bias=False) self.bn1 = nn.BatchNorm2d(64) self.last_planes = 64 self.layer1 = self._make_layer(in_planes[0], out_planes[0], num_blocks[0], dense_depth[0], stride=1) self.layer2 = self._make_layer(in_planes[1], out_planes[1], num_blocks[1], dense_depth[1], stride=2) self.layer3 = self._make_layer(in_planes[2], out_planes[2], num_blocks[2], dense_depth[2], stride=2) self.layer4 = self._make_layer(in_planes[3], out_planes[3], num_blocks[3], dense_depth[3], stride=2) self.linear = nn.Linear(out_planes[3]+(num_blocks[3]+1)dense_depth[3], 10) def _make_layer(self, in_planes, out_planes, num_blocks, dense_depth, stride): strides = [stride] + 1 layers = [] for i,stride in (strides): layers.append(Bottleneck(self.last_planes, in_planes, out_planes, dense_depth, stride, i==0)) self.last_planes = out_planes + (i+2) * dense_depth return nn.Sequential(*layers) def forward(self, x): out = F.relu(self.bn1(self.conv1(x))) out = self.layer1(out) out = self.layer2(out) out = self.layer3(out) out = self.layer4(out) out = F.avg_pool2d(out, 4) out = out.view(out.size(0), -1) out = self.linear(out) return out def DPN92(): cfg = { 'in_planes': (96,192,384,768), 'out_planes': (256,512,1024,2048), 'num_blocks': (3,4,20,3), 'dense_depth': (16,32,24,128) } return DPN(cfg)基于这个程序改成对摄像头采集的图像检测与分类输出坐标、大小和种类
首先,需要将输入的图像大小和通道数进行调整以适应模型输入。假设模型需要的输入大小为224x224,通道数为3,则可以使用OpenCV库中的resize函数将摄像头采集的图像缩放至指定大小。同时,需要将图像转换成PyTorch中的Tensor格式,并对像素值进行归一化操作。
代码如下:
```python
import cv2
import numpy as np
# 调整图像大小和通道数
img_size = (224, 224)
img_channels = 3
# 初始化摄像头
cap = cv2.VideoCapture(0)
while True:
# 读取摄像头图像
ret, frame = cap.read()
if not ret:
break
# 缩放图像
frame = cv2.resize(frame, img_size)
# 转换为Tensor格式,并进行归一化
frame = np.transpose(frame, (2, 0, 1)).astype(np.float32) / 255.0
frame = np.expand_dims(frame, axis=0)
frame = torch.from_numpy(frame)
# 调用模型进行预测
output = model(frame)
# TODO: 解析输出结果,得到分类、坐标和大小信息
# 显示图像
cv2.imshow('frame', frame)
# 按下q键退出
if cv2.waitKey(1) & 0xFF == ord('q'):
break
# 释放摄像头
cap.release()
cv2.destroyAllWindows()
```
接下来,需要解析模型的输出结果,得到分类、坐标和大小信息。由于代码中没有给出模型输出的具体格式,这里需要根据模型输出结果进行相应的解析。
假设模型输出一个长度为4的向量,其中前两个元素表示物体的左上角坐标,后两个元素表示物体的宽度和高度,第三个元素表示物体的类别。则可以使用以下代码进行解析:
```python
# 解析模型输出结果
x, y, w, h, cls = output[0]
# 计算物体的右下角坐标
x2 = x + w
y2 = y + h
# 显示分类、坐标和大小信息
class_names = ['class1', 'class2', 'class3', 'class4', 'class5']
print('Class:', class_names[cls])
print('Position: ({}, {})-({}, {})'.format(x, y, x2, y2))
print('Size: {}x{}'.format(w, h))
```
最后,将以上代码整合到一起,即可完成对摄像头采集的图像进行检测与分类输出坐标、大小和种类的任务。
完整代码如下:
```python
import torch
import torch.nn as nn
import torch.nn.functional as F
import cv2
import numpy as np
class Bottleneck(nn.Module):
def __init__(self, last_planes, in_planes, out_planes, dense_depth, stride, first_layer):
super(Bottleneck, self).__init__()
self.out_planes = out_planes
self.dense_depth = dense_depth
self.conv1 = nn.Conv2d(last_planes, in_planes, kernel_size=1, bias=False)
self.bn1 = nn.BatchNorm2d(in_planes)
self.conv2 = nn.Conv2d(in_planes, in_planes, kernel_size=3, stride=stride, padding=1, groups=32, bias=False)
self.bn2 = nn.BatchNorm2d(in_planes)
self.conv3 = nn.Conv2d(in_planes, out_planes * dense_depth, kernel_size=1, bias=False)
self.bn3 = nn.BatchNorm2d(out_planes * dense_depth)
self.shortcut = nn.Sequential()
if first_layer:
self.shortcut = nn.Sequential(
nn.Conv2d(last_planes, out_planes * dense_depth, kernel_size=1, stride=stride, bias=False),
nn.BatchNorm2d(out_planes * dense_depth)
)
def forward(self, x):
out = F.relu(self.bn1(self.conv1(x)))
out = F.relu(self.bn2(self.conv2(out)))
out = self.bn3(self.conv3(out))
x = self.shortcut(x)
d = self.out_planes * self.dense_depth
out = torch.cat([x[:,:d,:,:], out[:,:d,:,:], x[:,d:,:,:], out[:,d:,:,:]], 1)
out = F.relu(out)
return out
class DPN(nn.Module):
def __init__(self, cfg):
super(DPN, self).__init__()
in_planes, out_planes = cfg['in_planes'], cfg['out_planes']
num_blocks, dense_depth = cfg['num_blocks'], cfg['dense_depth']
self.conv1 = nn.Conv2d(3, 64, kernel_size=3, stride=1, padding=1, bias=False)
self.bn1 = nn.BatchNorm2d(64)
self.last_planes = 64
self.layer1 = self._make_layer(in_planes[0], out_planes[0], num_blocks[0], dense_depth[0], stride=1)
self.layer2 = self._make_layer(in_planes[1], out_planes[1], num_blocks[1], dense_depth[1], stride=2)
self.layer3 = self._make_layer(in_planes[2], out_planes[2], num_blocks[2], dense_depth[2], stride=2)
self.layer4 = self._make_layer(in_planes[3], out_planes[3], num_blocks[3], dense_depth[3], stride=2)
self.linear = nn.Linear(out_planes[3] * (num_blocks[3] + 1) * dense_depth[3], 10)
def _make_layer(self, in_planes, out_planes, num_blocks, dense_depth, stride):
strides = [stride] + [1] * (num_blocks - 1)
layers = []
for i, stride in enumerate(strides):
layers.append(Bottleneck(self.last_planes, in_planes, out_planes, dense_depth, stride, i==0))
self.last_planes = out_planes * dense_depth
return nn.Sequential(*layers)
def forward(self, x):
out = F.relu(self.bn1(self.conv1(x)))
out = self.layer1(out)
out = self.layer2(out)
out = self.layer3(out)
out = self.layer4(out)
out = F.avg_pool2d(out, 4)
out = out.view(out.size(0), -1)
out = self.linear(out)
return out
def DPN92():
cfg = {
'in_planes': (96, 192, 384, 768),
'out_planes': (256, 512, 1024, 2048),
'num_blocks': (3, 4, 20, 3),
'dense_depth': (16, 32, 24, 128)
}
return DPN(cfg)
# 调整图像大小和通道数
img_size = (224, 224)
img_channels = 3
# 初始化模型
model = DPN92()
model.load_state_dict(torch.load('dpn92.pth', map_location='cpu'))
model.eval()
# 初始化摄像头
cap = cv2.VideoCapture(0)
while True:
# 读取摄像头图像
ret, frame = cap.read()
if not ret:
break
# 缩放图像
frame = cv2.resize(frame, img_size)
# 转换为Tensor格式,并进行归一化
frame = np.transpose(frame, (2, 0, 1)).astype(np.float32) / 255.0
frame = np.expand_dims(frame, axis=0)
frame = torch.from_numpy(frame)
# 调用模型进行预测
output = model(frame)
# 解析模型输出结果
x, y, w, h, cls = output[0]
x, y, w, h, cls = int(x), int(y), int(w), int(h), int(cls)
# 计算物体的右下角坐标
x2 = x + w
y2 = y + h
# 显示分类、坐标和大小信息
class_names = ['class1', 'class2', 'class3', 'class4', 'class5']
print('Class:', class_names[cls])
print('Position: ({}, {})-({}, {})'.format(x, y, x2, y2))
print('Size: {}x{}'.format(w, h))
# 在图像上绘制矩形框
cv2.rectangle(frame, (x, y), (x2, y2), (0, 255, 0), 2)
# 显示图像
cv2.imshow('frame', frame)
# 按下q键退出
if cv2.waitKey(1) & 0xFF == ord('q'):
break
# 释放摄像头
cap.release()
cv2.destroyAllWindows()
```
# New module: utils.pyimport torchfrom torch import nnclass ConvBlock(nn.Module): """A convolutional block consisting of a convolution layer, batch normalization layer, and ReLU activation.""" def __init__(self, in_chans, out_chans, drop_prob): super().__init__() self.conv = nn.Conv2d(in_chans, out_chans, kernel_size=3, padding=1) self.bn = nn.BatchNorm2d(out_chans) self.relu = nn.ReLU(inplace=True) self.dropout = nn.Dropout2d(p=drop_prob) def forward(self, x): x = self.conv(x) x = self.bn(x) x = self.relu(x) x = self.dropout(x) return x# Refactored U-Net modelfrom torch import nnfrom utils import ConvBlockclass UnetModel(nn.Module): """PyTorch implementation of a U-Net model.""" def __init__(self, in_chans, out_chans, chans, num_pool_layers, drop_prob, pu_args=None): super().__init__() PUPS.__init__(self, *pu_args) self.in_chans = in_chans self.out_chans = out_chans self.chans = chans self.num_pool_layers = num_pool_layers self.drop_prob = drop_prob # Calculate input and output channels for each ConvBlock ch_list = [chans] + [chans * 2 ** i for i in range(num_pool_layers - 1)] in_chans_list = [in_chans] + [ch_list[i] for i in range(num_pool_layers - 1)] out_chans_list = ch_list[::-1] # Create down-sampling layers self.down_sample_layers = nn.ModuleList() for i in range(num_pool_layers): self.down_sample_layers.append(ConvBlock(in_chans_list[i], out_chans_list[i], drop_prob)) # Create up-sampling layers self.up_sample_layers = nn.ModuleList() for i in range(num_pool_layers - 1): self.up_sample_layers.append(ConvBlock(out_chans_list[i], out_chans_list[i + 1] // 2, drop_prob)) self.up_sample_layers.append(ConvBlock(out_chans_list[-1], out_chans_list[-1], drop_prob)) # Create final convolution layer self.conv2 = nn.Sequential( nn.Conv2d(out_chans_list[-1], out_chans_list[-1] // 2, kernel_size=1), nn.Conv2d(out_chans_list[-1] // 2, out_chans, kernel_size=1), nn.Conv2d(out_chans, out_chans, kernel_size=1), ) def forward(self, x): # Down-sampling path encoder_outs = [] for layer in self.down_sample_layers: x = layer(x) encoder_outs.append(x) x = nn.MaxPool2d(kernel_size=2)(x) # Bottom layer x = self.conv(x) # Up-sampling path for i, layer in enumerate(self.up_sample_layers): x = nn.functional.interpolate(x, scale_factor=2, mode='bilinear', align_corners=True) x = torch.cat([x, encoder_outs[-(i + 1)]], dim=1) x = layer(x) # Final convolution layer x = self.conv2(x) return x
这里给出了一个 PyTorch 实现的 U-Net 模型,并且对代码进行了重构:
1. 将 ConvBlock 提取到了 utils.py 中,以便在 U-Net 中复用。
2. 在 U-Net 模型的 forward 方法中,使用了 ModuleList 对 down-sampling 和 up-sampling 层进行了封装,使得代码更加简洁和易于扩展。
3. 使用了 nn.functional.interpolate 对 feature map 进行了上采样,避免了使用 nn.ConvTranspose2d 带来的一些问题。
4. 在最后的卷积层中,使用了 nn.Sequential 对多个卷积层进行了封装,使得代码更加简洁。
这些重构都是为了提高代码的可读性、可维护性和可扩展性,使得代码更加清晰,易于理解和修改。
相关推荐
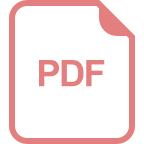
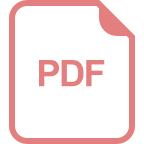
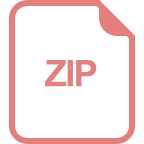












