Pso-Gabor算法
时间: 2023-05-22 15:07:30 浏览: 61
Pso-Gabor算法是一种基于粒子群优化和Gabor滤波器的图像分割算法。它将图像分割问题转化为一个优化问题,通过不断迭代优化粒子群的位置和速度,最终得到一组最优的Gabor滤波器参数,从而实现对图像的分割。该算法在图像处理领域有着广泛的应用,尤其在医学影像分割等领域表现突出。
相关问题
pso-gp算法csdn
PSO-GP算法是一种结合了粒子群优化(Particle Swarm Optimization,PSO)和高斯过程(Gaussian Process,GP)的优化算法。PSO-GP算法通过模拟粒子在搜索空间中的行为和能力,以及利用高斯过程对目标函数进行建模和预测,从而寻找最优解。
PSO-GP算法的基本思想是通过多个粒子在搜索空间中进行搜索,并根据自身经验和邻居粒子的信息进行位置和速度的更新。在每一代迭代中,粒子根据自身的历史最优位置和邻居粒子的历史最优位置进行位置和速度的调整,从而逐渐向全局最优解靠近。
同时,PSO-GP算法利用高斯过程对目标函数进行建模和预测。高斯过程是一种用于建立随机过程和概率分布模型的方法,能够通过有限个训练样本对目标函数进行建模,并根据建模结果对未知样本进行预测。在PSO-GP算法中,通过将高斯过程应用到目标函数建模中,可以更准确地预测目标函数的取值,并将预测结果用于粒子的位置更新和适应度评估。
综上所述,PSO-GP算法将粒子群优化和高斯过程相结合,通过模拟粒子在搜索空间中的行为和能力,并利用高斯过程对目标函数进行建模和预测,实现了对复杂优化问题的求解。它不仅具有全局搜索能力强、优化速度快的特点,还能够对目标函数进行准确的建模和预测,从而提高了优化的效果和效率。
PSO-kmeans算法
PSO-kmeans算法是一种基于粒子群优化算法的聚类算法,它结合了传统的kmeans算法和粒子群优化算法的优点,能够更快、更准确地找到数据集的聚类中心。
具体来说,PSO-kmeans算法的流程如下:
1. 初始化粒子群的位置和速度,其中每个粒子代表一个kmeans聚类的结果。
2. 计算每个粒子的适应度,即该聚类结果的误差平方和。
3. 找出粒子群中适应度最好的粒子,将其作为全局最优解。
4. 更新每个粒子的速度和位置,使其向全局最优解和局部最优解(即该粒子周围的最优解)移动。
5. 再次计算每个粒子的适应度。
6. 如果满足终止条件(如达到最大迭代次数或误差小于某个阈值),则结束算法,否则返回步骤4。
与传统的kmeans算法相比,PSO-kmeans算法能够避免陷入局部最优解,同时也不需要手动指定聚类中心的个数。但是,PSO-kmeans算法的计算量比较大,需要选择合适的参数和调节算法的收敛速度。
相关推荐
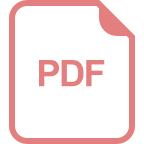
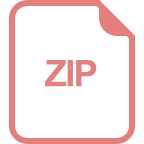












