mobilenetv1代码pytorch
时间: 2023-05-31 17:01:53 浏览: 88
### 回答1:
Mobilenetv1是一种轻量级的卷积神经网络,适用于移动设备和嵌入式设备。在PyTorch中,可以使用torchvision.models.mobilenet_v1来加载预训练的模型,也可以使用该模型的代码进行自定义训练和推理。以下是一个简单的Mobilenetv1代码示例:
```
import torch
import torch.nn as nn
import torch.nn.functional as F
class MobileNetV1(nn.Module):
def __init__(self, num_classes=100):
super(MobileNetV1, self).__init__()
self.conv1 = nn.Conv2d(3, 32, kernel_size=3, stride=2, padding=1, bias=False)
self.bn1 = nn.BatchNorm2d(32)
self.relu = nn.ReLU(inplace=True)
self.conv2 = nn.Conv2d(32, 64, kernel_size=3, stride=1, padding=1, bias=False)
self.bn2 = nn.BatchNorm2d(64)
self.conv3 = nn.Conv2d(64, 128, kernel_size=3, stride=2, padding=1, bias=False)
self.bn3 = nn.BatchNorm2d(128)
self.conv4 = nn.Conv2d(128, 128, kernel_size=3, stride=1, padding=1, bias=False)
self.bn4 = nn.BatchNorm2d(128)
self.conv5 = nn.Conv2d(128, 256, kernel_size=3, stride=2, padding=1, bias=False)
self.bn5 = nn.BatchNorm2d(256)
self.conv6 = nn.Conv2d(256, 256, kernel_size=3, stride=1, padding=1, bias=False)
self.bn6 = nn.BatchNorm2d(256)
self.conv7 = nn.Conv2d(256, 512, kernel_size=3, stride=2, padding=1, bias=False)
self.bn7 = nn.BatchNorm2d(512)
self.conv8 = nn.Conv2d(512, 512, kernel_size=3, stride=1, padding=1, bias=False)
self.bn8 = nn.BatchNorm2d(512)
self.conv9 = nn.Conv2d(512, 512, kernel_size=3, stride=1, padding=1, bias=False)
self.bn9 = nn.BatchNorm2d(512)
self.conv10 = nn.Conv2d(512, 512, kernel_size=3, stride=1, padding=1, bias=False)
self.bn10 = nn.BatchNorm2d(512)
self.conv11 = nn.Conv2d(512, 512, kernel_size=3, stride=1, padding=1, bias=False)
self.bn11 = nn.BatchNorm2d(512)
self.conv12 = nn.Conv2d(512, 1024, kernel_size=3, stride=2, padding=1, bias=False)
self.bn12 = nn.BatchNorm2d(1024)
self.conv13 = nn.Conv2d(1024, 1024, kernel_size=3, stride=1, padding=1, bias=False)
self.bn13 = nn.BatchNorm2d(1024)
self.avgpool = nn.AdaptiveAvgPool2d((1, 1))
self.fc = nn.Linear(1024, num_classes)
def forward(self, x):
x = self.conv1(x)
x = self.bn1(x)
x = self.relu(x)
x = self.conv2(x)
x = self.bn2(x)
x = self.relu(x)
x = self.conv3(x)
x = self.bn3(x)
x = self.relu(x)
x = self.conv4(x)
x = self.bn4(x)
x = self.relu(x)
x = self.conv5(x)
x = self.bn5(x)
x = self.relu(x)
x = self.conv6(x)
x = self.bn6(x)
x = self.relu(x)
x = self.conv7(x)
x = self.bn7(x)
x = self.relu(x)
x = self.conv8(x)
x = self.bn8(x)
x = self.relu(x)
x = self.conv9(x)
x = self.bn9(x)
x = self.relu(x)
x = self.conv10(x)
x = self.bn10(x)
x = self.relu(x)
x = self.conv11(x)
x = self.bn11(x)
x = self.relu(x)
x = self.conv12(x)
x = self.bn12(x)
x = self.relu(x)
x = self.conv13(x)
x = self.bn13(x)
x = self.relu(x)
x = self.avgpool(x)
x = x.view(x.size(), -1)
x = self.fc(x)
return x
```
在这个代码中,我们定义了一个名为MobileNetV1的类,它继承自nn.Module。在__init__函数中,我们定义了网络的各个层,包括卷积层、批归一化层和ReLU激活函数。在forward函数中,我们按照顺序将输入x传递到各个层中,并最终输出分类结果。这个代码可以用于自定义训练和推理,也可以作为torchvision.models.mobilenet_v1的基础代码进行修改和扩展。
### 回答2:
MobileNet V1是一种轻量级深度学习模型,被广泛应用于移动设备和嵌入式设备上进行图像分类任务。在PyTorch中,我们可以使用现成的MobileNet V1代码,快速搭建模型并进行训练和预测。
MobileNet V1的PyTorch代码实现,可以在PyTorch的官方Github仓库中找到。该代码库提供了两种不同版本的MobileNet V1模型,包括预先训练好的模型和用于自定义训练的模型。这些代码使用了PyTorch的函数和类,以及提供了许多用于优化和调整模型的工具。
MobileNet V1代码使用了深度可分离卷积(Depthwise Separable Convolution)来减少模型的计算和内存需求。这种卷积以一种新颖的方式处理特征图,将必要的计算分散到每个通道上。此外,代码还使用了全局平均池化层,将每个特征图替换为其平均值,从而减少了特征图的大小和维度。
使用PyTorch的MobileNet V1代码非常简单。您只需要调用相应的函数来定义和构建模型,并在训练和预测时向其提供相应的输入和输出张量即可。该代码也提供了各种用于数据增强、优化和调整模型的工具,方便用户进行优化和调整。
综上所述,MobileNet V1的PyTorch代码是一种功能强大、易于使用的深度学习工具,它能够在移动设备和嵌入式设备上快速地实现图像分类任务。无论您是初学者还是有经验的深度学习专业人员,该代码库都是一个必不可少的工具。
### 回答3:
MobileNetV1是一款具有高效网络架构的深度学习模型,它可以用于图像分类、目标检测等应用场景。该模型特别适用于具有限制计算资源的移动设备。
在PyTorch中,MobileNetV1代码可以通过下面的方式进行实现:
1. 安装PyTorch库,并导入需要使用的模块:
```
import torch
import torch.nn as nn
import torch.nn.functional as F
```
2. 定义MobileNetV1中的基本模块:
```
class ConvBNReLU(nn.Module):
def __init__(self, in_planes, out_planes, kernel_size=3, stride=1, groups=1):
super(ConvBNReLU, self).__init__()
padding = (kernel_size - 1) // 2
self.conv = nn.Conv2d(in_planes, out_planes, kernel_size, stride, padding, groups=groups, bias=False)
self.bn = nn.BatchNorm2d(out_planes)
self.relu = nn.ReLU(inplace=True)
def forward(self, x):
x = self.conv(x)
x = self.bn(x)
x = self.relu(x)
return x
```
3. 定义MobileNetV1的主体结构:
```
class MobileNetV1(nn.Module):
def __init__(self, num_classes=1000):
super(MobileNetV1, self).__init__()
self.conv1 = ConvBNReLU(3, 32, stride=2)
self.dw1 = nn.Sequential(
ConvBNReLU(32, 32, groups=32),
nn.Conv2d(32, 64, 1, 1, 0, bias=False),
nn.BatchNorm2d(64),
nn.ReLU(inplace=True),
)
self.dw2 = nn.Sequential(
ConvBNReLU(64, 64, stride=2, groups=64),
nn.Conv2d(64, 128, 1, 1, 0, bias=False),
nn.BatchNorm2d(128),
nn.ReLU(inplace=True),
)
self.dw3 = nn.Sequential(
ConvBNReLU(128, 128, stride=2, groups=128),
nn.Conv2d(128, 256, 1, 1, 0, bias=False),
nn.BatchNorm2d(256),
nn.ReLU(inplace=True),
)
self.dw4 = nn.Sequential(
ConvBNReLU(256, 256, stride=2, groups=256),
nn.Conv2d(256, 512, 1, 1, 0, bias=False),
nn.BatchNorm2d(512),
nn.ReLU(inplace=True),
nn.AdaptiveAvgPool2d(1)
)
self.linear = nn.Linear(512, num_classes)
def forward(self, x):
x = self.conv1(x)
x = self.dw1(x)
x = self.dw2(x)
x = self.dw3(x)
x = self.dw4(x)
x = x.view(x.size(0), -1)
x = self.linear(x)
return x
```
在上述代码中,MobileNetV1的主体结构由5个“深度可分离卷积”组成。这些卷积层的参数量很小,并且可以提高计算效率。每个“深度可分离卷积”由一个深度卷积和一个1×1卷积层组成。
最后,使用MobileNetV1可以进行图像分类任务训练,示例如下:
```
# create a MobileNetV1 model
model = MobileNetV1(num_classes=10)
# define a loss function and optimizer
criterion = nn.CrossEntropyLoss()
optimizer = torch.optim.Adam(model.parameters(), lr=0.001)
# train the model
for epoch in range(10):
for i, (images, labels) in enumerate(train_loader):
optimizer.zero_grad()
outputs = model(images)
loss = criterion(outputs, labels)
loss.backward()
optimizer.step()
if (i+1) % 100 == 0:
print ('Epoch [{}/{}], Batch [{}/{}], Loss: {:.4f}'
.format(epoch+1, 10, i+1, len(train_loader), loss.item()))
# test the model
with torch.no_grad():
correct = 0
total = 0
for images, labels in test_loader:
outputs = model(images)
_, predicted = torch.max(outputs.data, 1)
total += labels.size(0)
correct += (predicted == labels).sum().item()
print('Accuracy of the model on the test images: {} %'.format(100 * correct / total))
```
在这个例子中,我们定义了一个包含10类的图像分类任务。通过使用PyTorch实现的MobileNetV1模型,我们可以训练并测试该模型在这个任务上的性能。
相关推荐
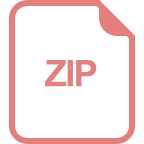
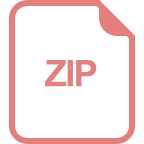
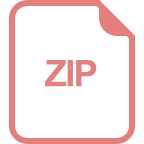














