Mobilenetv3代码
时间: 2023-07-02 10:05:34 浏览: 95
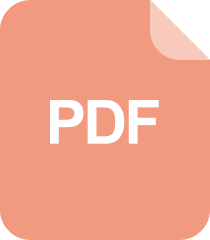
mobilenetv3
以下是使用PyTorch实现MobileNetV3的代码:
```python
import torch
import torch.nn as nn
import torch.nn.functional as F
class Hswish(nn.Module):
def __init__(self, inplace=True):
super(Hswish, self).__init__()
self.inplace = inplace
def forward(self, x):
if self.inplace:
return x.mul_(F.relu6(x + 3., inplace=True)) / 6.
else:
return F.relu6(x + 3.) * x / 6.
class Hsigmoid(nn.Module):
def __init__(self, inplace=True):
super(Hsigmoid, self).__init__()
self.inplace = inplace
def forward(self, x):
if self.inplace:
return F.relu6(x + 3., inplace=True) / 6.
else:
return F.relu6(x + 3.) / 6.
class SEModule(nn.Module):
def __init__(self, in_channels, reduction_ratio=4):
super(SEModule, self).__init__()
self.avg_pool = nn.AdaptiveAvgPool2d(1)
self.fc1 = nn.Conv2d(in_channels, in_channels // reduction_ratio, kernel_size=1, bias=False)
self.relu = nn.ReLU(inplace=True)
self.fc2 = nn.Conv2d(in_channels // reduction_ratio, in_channels, kernel_size=1, bias=False)
self.hsigmoid = Hsigmoid()
def forward(self, x):
module_input = x
x = self.avg_pool(x)
x = self.fc1(x)
x = self.relu(x)
x = self.fc2(x)
x = self.hsigmoid(x)
return module_input * x
class MobileNetV3Block(nn.Module):
def __init__(self, in_channels, out_channels, kernel_size, stride, use_se, activation):
super(MobileNetV3Block, self).__init__()
self.use_se = use_se
self.activation = activation
padding = (kernel_size - 1) // 2
self.conv1 = nn.Conv2d(in_channels, in_channels, kernel_size=1, stride=1, padding=0, bias=False)
self.bn1 = nn.BatchNorm2d(in_channels)
self.conv2 = nn.Conv2d(in_channels, in_channels, kernel_size=kernel_size, stride=stride, padding=padding, groups=in_channels, bias=False)
self.bn2 = nn.BatchNorm2d(in_channels)
self.conv3 = nn.Conv2d(in_channels, out_channels, kernel_size=1, stride=1, padding=0, bias=False)
self.bn3 = nn.BatchNorm2d(out_channels)
if use_se:
self.se = SEModule(out_channels)
if activation == 'relu':
self.activation_fn = nn.ReLU(inplace=True)
elif activation == 'hswish':
self.activation_fn = Hswish(inplace=True)
def forward(self, x):
module_input = x
x = self.conv1(x)
x = self.bn1(x)
x = self.activation_fn(x)
x = self.conv2(x)
x = self.bn2(x)
x = self.activation_fn(x)
x = self.conv3(x)
x = self.bn3(x)
if self.use_se:
x = self.se(x)
x += module_input
return x
class MobileNetV3Large(nn.Module):
def __init__(self, num_classes=1000):
super(MobileNetV3Large, self).__init__()
# Settings for feature extraction part
self.conv1 = nn.Conv2d(3, 16, kernel_size=3, stride=2, padding=1, bias=False)
self.bn1 = nn.BatchNorm2d(16)
self.hs1 = Hswish()
self.block1 = MobileNetV3Block(16, 16, kernel_size=3, stride=1, use_se=False, activation='relu')
self.block2 = MobileNetV3Block(16, 24, kernel_size=3, stride=2, use_se=False, activation='relu')
self.block3 = MobileNetV3Block(24, 24, kernel_size=3, stride=1, use_se=False, activation='relu')
self.block4 = MobileNetV3Block(24, 40, kernel_size=5, stride=2, use_se=True, activation='relu')
self.block5 = MobileNetV3Block(40, 40, kernel_size=5, stride=1, use_se=True, activation='relu')
self.block6 = MobileNetV3Block(40, 40, kernel_size=5, stride=1, use_se=True, activation='relu')
self.block7 = MobileNetV3Block(40, 80, kernel_size=3, stride=2, use_se=False, activation='hswish')
self.block8 = MobileNetV3Block(80, 80, kernel_size=3, stride=1, use_se=False, activation='hswish')
self.block9 = MobileNetV3Block(80, 80, kernel_size=3, stride=1, use_se=False, activation='hswish')
self.block10 = MobileNetV3Block(80, 112, kernel_size=3, stride=1, use_se=True, activation='hswish')
self.block11 = MobileNetV3Block(112, 112, kernel_size=3, stride=1, use_se=True, activation='hswish')
self.block12 = MobileNetV3Block(112, 160, kernel_size=5, stride=2, use_se=True, activation='hswish')
self.block13 = MobileNetV3Block(160, 160, kernel_size=5, stride=1, use_se=True, activation='hswish')
self.block14 = MobileNetV3Block(160, 160, kernel_size=5, stride=1, use_se=True, activation='hswish')
# Settings for classification part
self.conv2 = nn.Conv2d(160, 960, kernel_size=1, stride=1, padding=0, bias=False)
self.bn2 = nn.BatchNorm2d(960)
self.hs2 = Hswish()
self.avgpool = nn.AdaptiveAvgPool2d(1)
self.dropout = nn.Dropout(p=0.2, inplace=True)
self.fc = nn.Linear(960, num_classes)
# Weight initialization
self._initialize_weights()
def forward(self, x):
x = self.conv1(x)
x = self.bn1(x)
x = self.hs1(x)
x = self.block1(x)
x = self.block2(x)
x = self.block3(x)
x = self.block4(x)
x = self.block5(x)
x = self.block6(x)
x = self.block7(x)
x = self.block8(x)
x = self.block9(x)
x = self.block10(x)
x = self.block11(x)
x = self.block12(x)
x = self.block13(x)
x = self.block14(x)
x = self.conv2(x)
x = self.bn2(x)
x = self.hs2(x)
x = self.avgpool(x)
x = x.view(x.size(0), -1)
x = self.dropout(x)
x = self.fc(x)
return x
def _initialize_weights(self):
for m in self.modules():
if isinstance(m, nn.Conv2d):
nn.init.kaiming_normal_(m.weight, mode='fan_out', nonlinearity='relu')
if m.bias is not None:
nn.init.zeros_(m.bias)
elif isinstance(m, nn.BatchNorm2d):
nn.init.ones_(m.weight)
nn.init.zeros_(m.bias)
elif isinstance(m, nn.Linear):
nn.init.normal_(m.weight, 0, 0.01)
nn.init.zeros_(m.bias)
```
这是MobileNetV3的大型版本,你也可以根据需要修改输入和输出通道数等参数来创建其他版本的MobileNetV3。
阅读全文
相关推荐
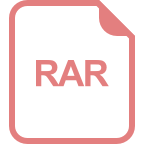



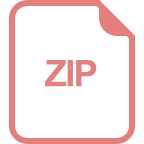










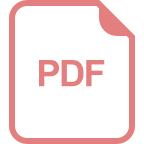
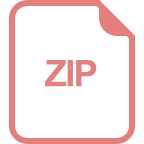