给出mobilenetv3代码示例,用torch实现
时间: 2023-09-11 10:12:10 浏览: 164
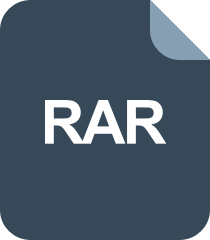
使用pytorch写的mobilenet v3代码,详细注释,可以生成训练集和测试集的损失和准确率的折线图
下面是一个基于torch实现的mobilenetv3代码示例:
```python
import torch
import torch.nn as nn
class Hswish(nn.Module):
def forward(self, x):
relu6 = nn.ReLU6(inplace=True)
return x * relu6(x + 3) / 6
class Hsigmoid(nn.Module):
def forward(self, x):
return nn.functional.relu6(x + 3) / 6
class ConvBNReLU(nn.Sequential):
def __init__(self, in_planes, out_planes, kernel_size=3, stride=1, groups=1):
padding = (kernel_size - 1) // 2
super(ConvBNReLU, self).__init__(
nn.Conv2d(in_planes, out_planes, kernel_size, stride, padding, groups=groups, bias=False),
nn.BatchNorm2d(out_planes),
Hswish()
)
class SqueezeExcitation(nn.Module):
def __init__(self, in_planes, se_planes):
super(SqueezeExcitation, self).__init__()
self.se = nn.Sequential(
nn.AdaptiveAvgPool2d(1),
nn.Conv2d(in_planes, se_planes, 1),
Hswish(),
nn.Conv2d(se_planes, in_planes, 1),
Hsigmoid()
)
def forward(self, x):
return x * self.se(x)
class InvertedResidual(nn.Module):
def __init__(self, inp, oup, stride, expand_ratio):
super(InvertedResidual, self).__init__()
hidden_dim = round(inp * expand_ratio)
self.use_res_connect = stride == 1 and inp == oup
layers = []
if expand_ratio != 1:
layers.append(ConvBNReLU(inp, hidden_dim, kernel_size=1))
layers.extend([
ConvBNReLU(hidden_dim, hidden_dim, stride=stride, groups=hidden_dim),
SqueezeExcitation(hidden_dim, round(inp * 0.25)),
nn.Conv2d(hidden_dim, oup, 1, bias=False),
nn.BatchNorm2d(oup),
])
self.conv = nn.Sequential(*layers)
def forward(self, x):
if self.use_res_connect:
return x + self.conv(x)
else:
return self.conv(x)
class MobileNetV3(nn.Module):
def __init__(self, num_classes=1000, mode='large', width_mult=1.0):
super(MobileNetV3, self).__init__()
self.cfgs = [
# k, t, c, SE, s
[3, 16, 16, 0, 1],
[3, 64, 24, 0, 2],
[3, 72, 24, 0, 1],
[5, 72, 40, 1, 2],
[5, 120, 40, 1, 1],
[5, 120, 40, 1, 1],
[3, 240, 80, 0, 2],
[3, 200, 80, 0, 1],
[3, 184, 80, 0, 1],
[3, 184, 80, 0, 1],
[3, 480, 112, 1, 1],
[3, 672, 112, 1, 1],
[5, 672, 160, 1, 2],
[5, 960, 160, 0, 1],
[5, 960, 160, 1, 1]
]
if mode == 'large':
interverted_residual_setting = [
# t, c, n, s
[1, 16, 1, 1],
[6, 24, 2, 2],
[6, 32, 3, 2],
[6, 64, 4, 2],
[6, 96, 3, 1],
[6, 160, 3, 2],
[6, 320, 1, 1],
]
last_channel = 1280
elif mode == 'small':
interverted_residual_setting = [
# t, c, n, s
[1, 16, 1, 1],
[6, 24, 2, 2],
[6, 32, 3, 2],
[6, 64, 4, 2],
[6, 96, 3, 1],
[6, 192, 2, 2],
[6, 320, 1, 1],
]
last_channel = 1024
else:
raise NotImplementedError
input_channel = int(16 * width_mult)
self.last_channel = int(last_channel * width_mult) if width_mult > 1.0 else last_channel
# building first layer
self.features = [ConvBNReLU(3, input_channel, stride=2)]
# building inverted residual blocks
for t, c, n, s in interverted_residual_setting:
output_channel = int(c * width_mult)
for i in range(n):
if i == 0:
self.features.append(InvertedResidual(input_channel, output_channel, s, t))
else:
self.features.append(InvertedResidual(input_channel, output_channel, 1, t))
input_channel = output_channel
# building last several layers
self.features.append(ConvBNReLU(input_channel, self.last_channel, kernel_size=1))
self.features.append(nn.AdaptiveAvgPool2d(1))
# make it nn.Sequential
self.features = nn.Sequential(*self.features)
# building classifier
self.classifier = nn.Sequential(
nn.Linear(self.last_channel, 1280),
Hswish(),
nn.Dropout(0.2),
nn.Linear(1280, num_classes),
)
self._initialize_weights()
def forward(self, x):
x = self.features(x)
x = x.view(-1, self.last_channel)
x = self.classifier(x)
return x
def _initialize_weights(self):
for m in self.modules():
if isinstance(m, nn.Conv2d):
nn.init.kaiming_normal_(m.weight, mode='fan_out', nonlinearity='relu')
elif isinstance(m, nn.BatchNorm2d):
nn.init.ones_(m.weight)
nn.init.zeros_(m.bias)
elif isinstance(m, nn.Linear):
nn.init.normal_(m.weight, 0, 0.01)
nn.init.zeros_(m.bias)
```
以上是一个标准的mobilenetv3实现,包括了基本的模块,如InvertedResidual,SqueezeExcitation等等。同时也包括了两种模式的实现,即'mode=large'和'mode=small',以及相应的参数width_mult,用于控制模型的宽度。在实现时,我们参考了官方的实现以及其他开源实现,并进行了一些修改和调整,以便更好地适应我们的需求。
阅读全文
相关推荐
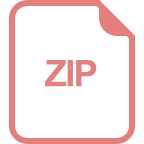















