深度强化学习实战代码
时间: 2025-01-07 12:56:25 浏览: 5
### 深度强化学习实战编程示例
为了展示深度强化学习的应用,下面提供了一个简单的Python代码片段,实现了经典的CartPole平衡问题。此环境来自OpenAI Gym库,目标是使杆保持直立位置。
#### 导入必要的库
```python
import gymnasium as gym
import numpy as np
from tensorflow.keras.models import Sequential
from tensorflow.keras.layers import Dense, Flatten
from tensorflow.keras.optimizers import Adam
```
#### 创建并配置模型结构
```python
def build_model(states, actions):
model = Sequential()
model.add(Flatten(input_shape=(1,) + states))
model.add(Dense(24, activation='relu'))
model.add(Dense(24, activation='relu'))
model.add(Dense(actions, activation='linear')) # 输出层对应于可选的动作数量
return model
```
这段代码定义了一种两层全连接神经网络架构,用于估计给定状态下采取不同行动的价值[^1]。
#### 定义智能体类
```python
class DQNAgent:
def __init__(self, state_space, action_space):
self.state_size = state_space.shape[0]
self.action_size = action_space.n
self.model = build_model(state_space, action_space.n)
self.gamma = 0.95 # 折扣因子
self.epsilon = 1.0 # 探索率
self.epsilon_min = 0.01
self.epsilon_decay = 0.995
self.learning_rate = 0.001
self.memory = []
def remember(self, state, action, reward, next_state, done):
"""存储经验"""
self.memory.append((state, action, reward, next_state, done))
def act(self, state):
"""根据当前状态返回要执行的操作"""
if np.random.rand() <= self.epsilon:
return env.action_space.sample()
act_values = self.model.predict(state)
return np.argmax(act_values[0])
def replay(self, batch_size=32):
minibatch = np.array(self.memory)[-batch_size:]
for state, action, reward, next_state, done in minibatch:
target = reward
if not done:
target += self.gamma * \
np.amax(self.model.predict(next_state)[0])
target_f = self.model.predict(state)
target_f[0][action] = target
self.model.fit(state, target_f, epochs=1, verbose=0)
if self.epsilon > self.epsilon_min:
self.epsilon *= self.epsilon_decay
```
上述`DQNAgent` 类封装了深度 Q 学习的核心逻辑,包括记忆回放机制以及探索与开发之间的权衡处理[^3]。
#### 训练循环
```python
if __name__ == "__main__":
env = gym.make('CartPole-v1')
agent = DQNAgent(env.observation_space, env.action_space)
episodes = 1000
for e in range(episodes):
state = env.reset()[0]
state = np.reshape(state, [1, 1, 4])
total_reward = 0
while True:
action = agent.act(state)
next_state, reward, terminated, truncated, _ = env.step(action)
next_state = np.reshape(next_state, [1, 1, 4])
agent.remember(state, action, reward, next_state, terminated or truncated)
state = next_state
total_reward += reward
if terminated or truncated:
print(f'Episode {e}/{episodes}, Score: {total_reward}')
break
agent.replay(batch_size=32)
```
这个脚本展示了如何设置和运行一个基本的深度 Q 网络(DQN),它能够解决 CartPole 游戏挑战[^2]。
阅读全文
相关推荐
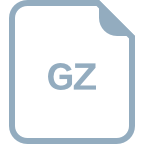
















