"C:\Users\WXF\Application Data\Scripts\python.exe" C:\20\frames\n.py No Python at 'D:\BaiduNetdiskDownload\pyhon3.9.0\python.exe' Process finished with exit code 103
时间: 2023-06-25 10:06:58 浏览: 156
这个错误提示意味着你的 Python 解释器路径设置出现了问题。在你的代码中,Python 解释器路径应该是 "C:\Users\WXF\Application Data\Scripts\python.exe",但错误信息中显示的路径是 "D:\BaiduNetdiskDownload\pyhon3.9.0\python.exe",这两个路径不一致。
你可以尝试修改你的代码,将 Python 解释器路径修改为正确的路径 "C:\Users\WXF\Application Data\Scripts\python.exe"。
另外,如果你安装了多个版本的 Python,需要确认你在使用的是正确的 Python 解释器。你可以通过在命令行中执行 "where python" 命令来确认当前使用的 Python 解释器路径。
相关问题
如何将 Python 解释器路径修改为正确的路径 "C:\Users\WXF\Application Data\Scripts\python.exe"。,命令行在哪里
在 Windows 操作系统中,可以通过以下步骤将 Python 解释器路径修改为正确的路径 "C:\Users\WXF\Application Data\Scripts\python.exe":
1. 打开命令提示符(cmd)窗口;
2. 输入命令 `setx path "%path%;C:\Users\WXF\Application Data\Scripts"`。这个命令将会把路径添加到环境变量中;
3. 退出cmd窗口。
现在,你可以重新打开一个cmd窗口,输入`python`,应该会使用正确的Python解释器。
#创建一个dataset类。 import os import pandas as pd from torchvision.io import read_image from torch.utils.data import Dataset from torch.utils.data import DataLoader import chardet with open(r'C:\Users\WXF\data\cifar10\cifar-10-batches-py\batches.meta', 'rb') as fp: result = chardet.detect(fp.read()) print(result) class CustomImageDataset(Dataset): def __init__(self, annotations_file, img_dir, transform=None, target_transform=None): #self.img_labels = pd.read_csv(annotations_file, sep=' ', header=None, encoding=result['encoding']) self.img_labels = pd.read_csv(annotations_file, sep=';', header=None, encoding=result['encoding']) self.img_labels[0] = self.img_labels[0].astype(str).str.cat(sep=' ') # 合并第一列为完整文件名 self.img_dir = img_dir self.transform = transform self.target_transform = target_transform def __len__(self): return len(self.img_labels) def __getitem__(self, idx): img_path = os.path.join(self.img_dir, self.img_labels.iloc[idx, 0]) image = read_image(img_path) label = self.img_labels.iloc[idx, 1] if self.transform: image = self.transform(image) if self.target_transform: label = self.target_transform(label) return image, label train_dataset = CustomImageDataset(annotations_file=r'C:\Users\WXF\data\cifar10\cifar-10-batches-py\batches.meta', img_dir = r'C:\Users\WXF\data\cifar10\cifar-10-batches-py\data_batch_1',transform=None, target_transform=None) test_dataset = CustomImageDataset(annotations_file=r'C:\Users\WXF\data\cifar10\cifar-10-batches-py\batches.meta', img_dir = r'C:\Users\WXF\data\cifar10\cifar-10-batches-py\test_batch',transform=None, target_transform=None) train_features, train_labels = next(iter(train_dataloader)) print(f"Feature batch shape: {train_features.size()}") print(f"Labels batch shape: {train_labels.size()}") img = train_features[0].squeeze() label = train_labels[0] plt.imshow(img, cmap="gray") plt.show() print(f"Label: {label}")
这段代码创建了一个自定义的图像数据集类 CustomImageDataset,用于读取 CIFAR-10 数据集中的图像数据和标签。该类继承了 PyTorch 中的 Dataset 类,并实现了 __init__、__len__ 和 __getitem__ 方法。其中,__init__ 方法用于初始化数据集,__len__ 方法返回数据集中样本的数量,__getitem__ 方法返回给定索引的图像数据和标签。在代码中,使用 pandas 库读取 CIFAR-10 数据集中的标签文件,然后根据文件名和路径读取图像数据,并将其返回。
此外,代码还创建了两个数据集对象 train_dataset 和 test_dataset,分别用于训练和测试。最后,使用 PyTorch 中的 DataLoader 类加载数据集对象,生成用于训练模型的数据批次。代码还展示了如何读取数据批次中的图像数据和标签,并使用 matplotlib 库显示图像和标签。
阅读全文
相关推荐
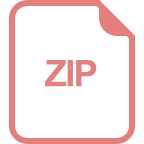
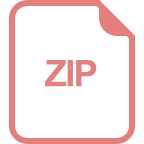
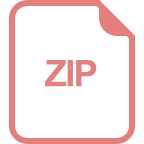
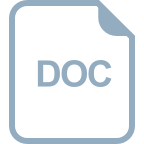

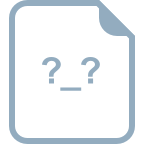
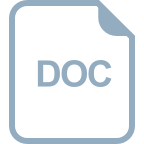






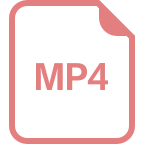
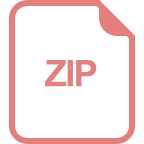