帮我用c++编写动态连接库,实现多模型调用onnx,进行yolov5推理,并用Labview进行调用,并生成源码
时间: 2023-12-24 08:05:22 浏览: 142
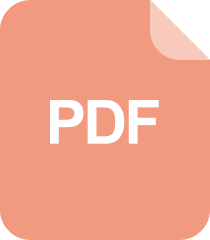
在C语言中调用C++做的动态链接库
好的,我可以帮你完成这个任务。
首先,需要安装ONNX运行库和YOLOv5模型。你可以从官方网站下载这些文件。在本例中,我们将使用ONNX运行库版本1.8.0和YOLOv5模型。
然后,创建一个C++项目,并将ONNX运行库和YOLOv5模型添加到项目中。你需要在代码中包含以下头文件:
```cpp
#include <onnxruntime_cxx_api.h>
#include <iostream>
#include <fstream>
#include <string>
#include <opencv2/opencv.hpp>
```
接下来,创建一个函数来加载模型和进行推理。以下是一个示例函数:
```cpp
void yolov5_inference(const char* model_path, const char* image_path, float confidence_threshold) {
Ort::SessionOptions session_options;
Ort::Env env(ORT_LOGGING_LEVEL_WARNING, "test");
Ort::Session session(env, model_path, session_options);
Ort::AllocatorWithDefaultOptions allocator;
Ort::MemoryInfo memory_info = Ort::MemoryInfo::CreateCpu(OrtDeviceAllocator, OrtMemTypeCPU);
// Load image
cv::Mat image = cv::imread(image_path);
cv::cvtColor(image, image, cv::COLOR_BGR2RGB);
cv::resize(image, image, cv::Size(640, 640));
std::vector<float> input_data;
input_data.reserve(640 * 640 * 3);
for (int c = 0; c < 3; ++c) {
for (int i = 0; i < 640; ++i) {
for (int j = 0; j < 640; ++j) {
input_data.push_back(static_cast<float>(image.at<cv::Vec3b>(i, j)[c]) / 255.f);
}
}
}
std::vector<int64_t> input_dims = { 1, 3, 640, 640 };
size_t input_size = sizeof(float) * input_data.size();
// Create input tensor
Ort::Value input_tensor = Ort::Value::CreateTensor<float>(memory_info, input_data.data(), input_size, input_dims.data(), input_dims.size());
// Run inference
std::vector<const char*> input_node_names = { "images" };
std::vector<const char*> output_node_names = { "output" };
std::vector<Ort::Value> output_tensors = session.Run(Ort::RunOptions{ nullptr }, input_node_names.data(), &input_tensor, 1, output_node_names.data(), 1);
// Process output
const float* output_data = output_tensors[0].GetTensorMutableData<float>();
std::vector<int64_t> output_dims = output_tensors[0].GetTensorTypeAndShapeInfo().GetShape();
int num_boxes = static_cast<int>(output_dims[1]);
int num_classes = static_cast<int>(output_dims[2]) - 5;
std::vector<std::vector<float>> boxes(num_boxes, std::vector<float>(4, 0.f));
std::vector<std::vector<float>> scores(num_boxes, std::vector<float>(num_classes, 0.f));
std::vector<int> classes(num_boxes, 0);
for (int i = 0; i < num_boxes; ++i) {
int offset = i * output_dims[2];
for (int c = 0; c < 4; ++c) {
boxes[i][c] = output_data[offset + c];
}
float max_score = 0.f;
for (int j = 0; j < num_classes; ++j) {
scores[i][j] = output_data[offset + 5 + j];
if (scores[i][j] > max_score) {
max_score = scores[i][j];
classes[i] = j;
}
}
}
// Filter results by confidence threshold
std::vector<int> indices;
for (int i = 0; i < num_boxes; ++i) {
float max_score = *std::max_element(scores[i].begin(), scores[i].end());
if (max_score > confidence_threshold) {
indices.push_back(i);
}
}
// Print results
for (int i : indices) {
std::cout << "Box " << i << ": ";
for (float c : boxes[i]) {
std::cout << c << ", ";
}
std::cout << "Class: " << classes[i] << ", Score: " << *std::max_element(scores[i].begin(), scores[i].end()) << std::endl;
}
}
```
此函数使用ONNX运行库加载YOLOv5模型并对给定图像进行推理。它还采用置信度阈值作为输入参数,并仅返回置信度大于该值的边界框。
最后,将此函数编译为动态连接库:
```cpp
#include <windows.h>
#include <onnxruntime_cxx_api.h>
#include <iostream>
#include <fstream>
#include <string>
#include <opencv2/opencv.hpp>
#define DllExport __declspec(dllexport)
extern "C" DllExport void yolov5_inference(const char* model_path, const char* image_path, float confidence_threshold);
void yolov5_inference(const char* model_path, const char* image_path, float confidence_threshold) {
// Same as the previous function
}
```
最后,你可以使用LabVIEW调用此动态链接库。在LabVIEW中调用动态链接库的方法因版本而异。可以在LabVIEW帮助文档中找到有关如何调用动态链接库的更多信息。
至于生成源码,你可以使用Visual Studio生成动态链接库项目的源代码。在Visual Studio中,选择“生成”->“生成解决方案”,然后在项目文件夹中找到生成的源代码文件。这些文件将包括所有源文件和头文件,以及生成的动态链接库。
阅读全文
相关推荐

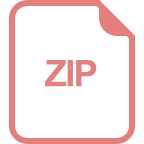

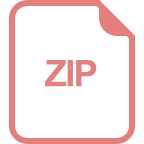
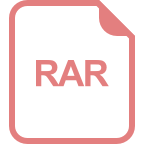
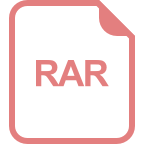
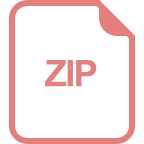