onnxruntime在gpu上部署加速
时间: 2023-09-06 20:06:01 浏览: 96
ONNX Runtime是由微软开发的一个用于优化和加速机器学习模型推理的引擎。它支持在CPU和GPU上进行部署,并提供了一些优化策略和技术来加速模型的推理过程。
在GPU上部署模型可以显著提高推理性能。首先,GPU具有高并行计算的特性,能够同时执行大量的计算任务。通过利用GPU的并行计算能力,ONNX Runtime可以将大部分的计算负载分配给GPU进行处理,从而加快模型的推理速度。
其次,ONNX Runtime通过使用GPU专用的并行计算库(如CUDA)来优化模型的推理过程。这些库提供了一些高效的算法和数据结构,可以在GPU上更快地执行计算任务。ONNX Runtime与这些库进行集成,可以充分利用GPU的硬件加速特性,进一步提高推理性能。
此外,ONNX Runtime还支持将模型的计算图和参数加载到GPU内存中,从而减少CPU和GPU之间的数据传输时间。将数据加载到GPU内存中可以减少数据在主机内存和设备之间的复制开销,进一步提高模型推理的效率。
总结起来,通过在GPU上部署和加速模型,ONNX Runtime能够利用GPU的并行计算能力和硬件加速特性,显著提高模型推理的速度和效率。这使得在处理大规模数据和复杂模型时,能够更快地完成推理任务,从而提升整体的机器学习应用性能。
相关问题
onnxruntime-gpu-c++部署
onnxruntime-gpu-c++是一个用于在GPU上运行模型的C++库,它支持使用ONNX格式的模型进行推理。下面是一个简单的部署步骤:
1. 安装CUDA和cuDNN
onnxruntime-gpu-c++需要CUDA和cuDNN来加速模型推理。您需要安装与onnxruntime-gpu-c++版本兼容的CUDA和cuDNN。您可以从NVIDIA官方网站下载并安装这些软件。
2. 安装onnxruntime-gpu-c++
您可以从onnxruntime-gpu-c++的GitHub仓库中下载源代码,并使用CMake生成库文件。在生成过程中,您需要指定CUDA和cuDNN的路径。
3. 加载模型
使用onnxruntime-gpu-c++加载ONNX格式的模型。您可以使用onnxruntime-cxx库中的API来加载模型并进行推理。以下是一个简单的示例代码:
```cpp
#include <iostream>
#include <vector>
#include <chrono>
#include <onnxruntime_cxx_api.h>
int main() {
Ort::SessionOptions session_options;
Ort::Env env(ORT_LOGGING_LEVEL_WARNING, "test");
Ort::Session session(env, "model.onnx", session_options);
Ort::AllocatorWithDefaultOptions allocator;
Ort::Value input_tensor = Ort::Value::CreateTensor<float>(allocator, {1, 3, 224, 224});
float* input_tensor_data = input_tensor.GetTensorMutableData<float>();
// fill input tensor with data ...
std::vector<const char*> input_names = {"input"};
std::vector<const char*> output_names = {"output"};
std::vector<int64_t> input_shape = {1, 3, 224, 224};
std::vector<float> output_data(1000);
Ort::RunOptions run_options;
Ort::TensorSlicer<float> input_tensor_slicer(input_tensor, input_shape);
auto start_time = std::chrono::high_resolution_clock::now();
for (auto& slice : input_tensor_slicer) {
Ort::Value input_tensor_slice = Ort::Value::CreateTensor<float>(allocator, slice.shape().data(), slice.shape().size(), slice.data(), slice.size());
Ort::Value output_tensor = session.Run(run_options, input_names.data(), &input_tensor_slice, 1, output_names.data(), 1);
std::memcpy(output_data.data() + slice.offset(), output_tensor.GetTensorData<float>(), slice.size() * sizeof(float));
}
auto end_time = std::chrono::high_resolution_clock::now();
std::cout << "Inference time: " << std::chrono::duration_cast<std::chrono::milliseconds>(end_time - start_time).count() << "ms" << std::endl;
// process output data ...
}
```
在这个示例中,我们使用onnxruntime-gpu-c++加载了名为“model.onnx”的模型,并将输入数据填充到名为“input”的张量中。然后,我们运行了推理,并将输出数据存储在名为“output_data”的向量中。最后,我们对输出数据进行了处理。
4. 运行推理
在加载模型和填充输入数据后,您可以使用session.Run()方法运行推理。您需要指定输入和输出张量的名称,并将它们传递给session.Run()方法。
5. 处理输出
session.Run()方法将返回一个或多个输出张量。您可以使用GetTensorData()方法获取输出张量的数据,并对其进行处理。
这些是一个简单的onnxruntime-gpu-c++部署步骤。您可以根据具体情况进行适当的修改。
onnxruntime_gpu-1.13.1-cp38-cp38-linux_aarch64.whl
onnxruntime_gpu-1.13.1-cp38-cp38-linux_aarch64.whl是针对Linux平台上使用aarch64架构的GPU加速版本的ONNX Runtime软件包。ONNX Runtime是一个开源的深度学习推断引擎,它支持在不同硬件平台上运行训练好的模型并进行推断。
这个软件包是针对在嵌入式系统或者边缘设备上进行深度学习推断的场景而设计的。通过GPU加速,可以大幅提升模型推断的速度,从而在资源有限的环境中提供更快速和高效的推断服务。
对于开发者来说,利用这个软件包可以轻松地在Linux平台上进行深度学习模型的部署和推断加速。另外,由于aarch64架构通常用于嵌入式设备或者边缘计算平台,因此这个软件包非常适合用于开发智能手机、物联网设备或者嵌入式系统中的深度学习应用。
总之,onnxruntime_gpu-1.13.1-cp38-cp38-linux_aarch64.whl是一个专门为嵌入式系统和边缘设备优化的GPU加速版本的ONNX Runtime软件包,它能够帮助开发者在资源有限的环境中实现高效的深度学习推断。
相关推荐
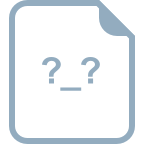












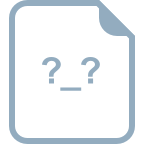