用Python的Matplotlib库来绘制PNG图片,并使用base64库将其转换为Base64格式,用于HTML图片中展示
时间: 2024-03-26 10:41:43 浏览: 15
可以使用Python的Matplotlib库来绘制PNG图片,然后使用base64库将其转换为Base64格式,最后将其嵌入HTML图片中展示。以下是一个示例代码:
```python
import base64
import matplotlib.pyplot as plt
# 绘制Matplotlib图表
fig, ax = plt.subplots()
ax.plot([1, 2, 3, 4], [1, 4, 2, 3])
# 将图表保存为PNG格式并转换为Base64格式
buffer = BytesIO()
plt.savefig(buffer, format='png')
buffer.seek(0)
image_png = buffer.getvalue()
image_base64 = base64.b64encode(image_png).decode()
# 将Base64格式的图片嵌入HTML图片中
html = f"<img src='data:image/png;base64,{image_base64}'/>"
# 输出HTML
print(html)
```
在这个示例代码中,我们首先创建了一个Matplotlib图表,然后使用`plt.savefig()`方法将其保存为PNG格式,并将其保存到BytesIO对象中。接下来,使用`base64.b64encode()`方法将PNG图片转换为Base64格式,并将其嵌入HTML图片中。最后,输出HTML即可。
需要注意的是,在将Base64格式的图片嵌入HTML图片中时,需要在Base64字符串前添加`data:image/png;base64,`前缀,以指定图片的格式和编码方式。
相关推荐
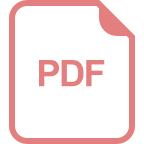
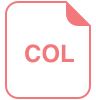
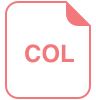
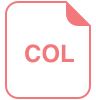
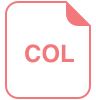
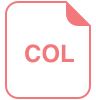










