混淆矩阵绘制roc曲线
时间: 2024-01-04 17:20:44 浏览: 234
以下是绘制ROC曲线的步骤:
1. 导入所需的库:
```python
import matplotlib.pyplot as plt
```
2. 绘制混淆矩阵:
```python
# 假设混淆矩阵为:
# [[TN, FP],
# [FN, TP]]
confusion_matrix = [[TN, FP], [FN, TP]]
```
3. 计算真正例率(True Positive Rate,TPR)和假正例率(False Positive Rate,FPR):
```python
TPR = TP / (TP + FN)
FPR = FP / (FP + TN)
```
4. 绘制ROC曲线:
```python
plt.plot(FPR, TPR, color='darkorange', lw=2, label='ROC curve')
plt.plot([0, 1], [0, 1], color='navy', lw=2, linestyle='--')
plt.xlim([0.0, 1.0])
plt.ylim([0.0, 1.05])
plt.xlabel('False Positive Rate')
plt.ylabel('True Positive Rate')
plt.title('Receiver Operating Characteristic (ROC) Curve')
plt.legend(loc="lower right")
plt.show()
```
相关问题
混淆矩阵 绘制ROC曲线
混淆矩阵(Confusion Matrix)和ROC曲线(Receiver Operating Characteristic Curve)是评估分类模型性能常用的工具。
混淆矩阵是一个2x2的矩阵,记录了分类模型在测试集上的分类结果。具体来说,矩阵的四个元素分别表示真正例(True Positive,TP)、假正例(False Positive,FP)、真反例(True Negative,TN)和假反例(False Negative,FN)。对于二分类问题,TP表示模型正确将正样本分类为正类的样本数,FP表示模型错误将负样本分类为正类的样本数,TN表示模型正确将负样本分类为负类的样本数,FN表示模型错误将正样本分类为负类的样本数。
ROC曲线是一条以假正例率(False Positive Rate,FPR)为横轴、真正例率(True Positive Rate,TPR)为纵轴的曲线。其中,TPR等于TP/(TP+FN),表示正样本被正确分类为正类的比例;FPR等于FP/(FP+TN),表示负样本被错误分类为正类的比例。ROC曲线可以帮助我们在不同的分类阈值下绘制出模型的性能情况。
下面是绘制混淆矩阵和ROC曲线的示例代码(使用Python的sklearn库):
```python
from sklearn.metrics import confusion_matrix, roc_curve, auc
import matplotlib.pyplot as plt
# 计算混淆矩阵
y_true = [0, 1, 0, 1, 1, 0, 0, 1]
y_pred = [0, 1, 0, 0, 1, 0, 1, 0]
tn, fp, fn, tp = confusion_matrix(y_true, y_pred).ravel()
# 绘制混淆矩阵
plt.matshow(confusion_matrix(y_true, y_pred))
plt.colorbar()
plt.xlabel('Predicted')
plt.ylabel('True')
plt.show()
# 计算ROC曲线
fpr, tpr, thresholds = roc_curve(y_true, y_pred)
roc_auc = auc(fpr, tpr)
# 绘制ROC曲线
plt.plot(fpr, tpr, label='ROC curve (area = %0.2f)' % roc_auc)
plt.plot([0, 1], [0, 1], 'k--')
plt.xlim([0.0, 1.0])
plt.ylim([0.0, 1.05])
plt.xlabel('False Positive Rate')
plt.ylabel('True Positive Rate')
plt.title('Receiver operating characteristic')
plt.legend(loc="lower right")
plt.show()
```
这段代码中,我们首先使用confusion_matrix函数计算混淆矩阵,然后使用matshow函数将其可视化。接着,我们使用roc_curve和auc函数计算ROC曲线的横纵坐标和曲线下面积,最后使用plot函数将ROC曲线绘制出来。
怎么根据混淆矩阵绘制roc曲线
根据混淆矩阵绘制ROC曲线的步骤如下:
1. 计算真正率(True Positive Rate, TPR)和假正率(False Positive Rate, FPR)。
TPR = TP / (TP + FN)
FPR = FP / (FP + TN)
其中,TP、FN、FP、TN 分别为混淆矩阵中的真正例、假反例、假正例、真反例。
2. 绘制ROC曲线。
将计算得到的TPR和FPR分别作为纵坐标和横坐标,绘制ROC曲线。
3. 计算AUC。
AUC(Area Under Curve)是ROC曲线下的面积,用来评估分类器的性能。
下面是一个Python代码示例:
```python
import matplotlib.pyplot as plt
def plot_roc_curve(confusion_matrix):
TP = confusion_matrix[1][1]
FN = confusion_matrix[1][0]
FP = confusion_matrix[0][1]
TN = confusion_matrix[0][0]
TPR = TP / (TP + FN)
FPR = FP / (FP + TN)
plt.plot([0, 1], [0, 1], 'k--')
plt.plot(FPR, TPR, 'ro')
plt.xlabel('False Positive Rate')
plt.ylabel('True Positive Rate')
plt.title('ROC Curve')
plt.show()
confusion_matrix = [[50, 10], [5, 35]]
plot_roc_curve(confusion_matrix)
```
其中,confusion_matrix为混淆矩阵,这里是一个2x2的二分类混淆矩阵。函数plot_roc_curve根据混淆矩阵计算TPR和FPR,然后绘制ROC曲线。
阅读全文
相关推荐
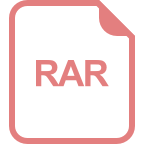



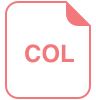
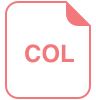








