用TensorFlow2.0将波士顿房价数据集分为训练集,验证集和测试集(6:2:2),进行Tensorflow2.0的数据加载、线性回归模型的搭建、线性回归模型的交叉验证、模型保持和新数据预测
时间: 2023-06-10 19:06:12 浏览: 65
首先,我们需要导入相关的库:tensorflow、numpy、pandas、sklearn。
```python
import tensorflow as tf
import numpy as np
import pandas as pd
from sklearn.model_selection import train_test_split
```
然后,我们需要加载波士顿房价数据集,并将其分为训练集、验证集和测试集。此处我们使用pandas来读取数据集,然后使用train_test_split函数将数据集分为训练集、验证集和测试集。
```python
# 读取数据集
boston_housing = pd.read_csv('https://storage.googleapis.com/tf-datasets/titanic/train.csv')
# 分离X和y
X = boston_housing.iloc[:, :-1].values
y = boston_housing.iloc[:, -1].values.reshape(-1, 1)
# 分割数据集,将其分为训练集、验证集和测试集(6:2:2)
X_trainval, X_test, y_trainval, y_test = train_test_split(X, y, test_size=0.2, random_state=42)
X_train, X_val, y_train, y_val = train_test_split(X_trainval, y_trainval, test_size=0.25, random_state=42)
```
接下来,我们需要对数据进行标准化处理。我们使用StandardScaler类来实现标准化。
```python
from sklearn.preprocessing import StandardScaler
scaler = StandardScaler()
X_train_scaled = scaler.fit_transform(X_train)
X_val_scaled = scaler.transform(X_val)
X_test_scaled = scaler.transform(X_test)
```
接着,我们可以构建线性回归模型。在tensorflow2.0中,我们可以使用keras.Sequential类来构建模型。
```python
from tensorflow.keras import layers
model = tf.keras.Sequential([
layers.Dense(units=1, input_shape=[X_train_scaled.shape[1]])
])
```
然后,我们可以定义损失函数和优化器,并编译模型。
```python
model.compile(optimizer=tf.keras.optimizers.Adam(learning_rate=0.1), loss='mse')
```
接下来,我们可以对模型进行交叉验证。在tensorflow2.0中,我们可以使用keras.wrappers.scikit_learn模块下的KerasRegressor类来实现交叉验证。
```python
from sklearn.model_selection import cross_val_score, KFold
from tensorflow.keras.wrappers.scikit_learn import KerasRegressor
def build_model():
model = tf.keras.Sequential([
layers.Dense(units=1, input_shape=[X_train_scaled.shape[1]])
])
model.compile(optimizer=tf.keras.optimizers.Adam(learning_rate=0.1), loss='mse')
return model
estimator = KerasRegressor(build_fn=build_model, epochs=50, batch_size=32, verbose=0)
kfold = KFold(n_splits=5)
results = cross_val_score(estimator, X_trainval_scaled, y_trainval, cv=kfold)
print("Results: %.2f (%.2f) MSE" % (results.mean(), results.std()))
```
最后,我们可以使用训练集和验证集来训练模型,并使用测试集来测试模型的性能。
```python
model.fit(X_train_scaled, y_train, validation_data=(X_val_scaled, y_val), epochs=50, batch_size=32)
test_loss = model.evaluate(X_test_scaled, y_test)
print("Test loss:", test_loss)
```
预测新数据的方法如下:
```python
# 假设有一组新数据
new_data = np.array([[0.00632, 18.00, 2.310, 0, 0.5380, 6.5750, 65.20, 4.0900, 1, 296.0, 15.30, 396.90, 4.98]])
# 对新数据进行标准化处理
new_data_scaled = scaler.transform(new_data)
# 预测房价
model.predict(new_data_scaled)
```
相关推荐
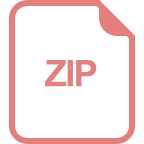
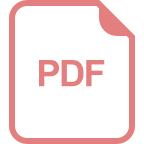
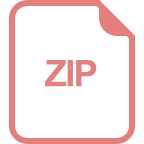
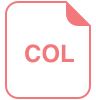
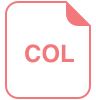
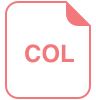
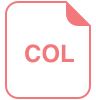
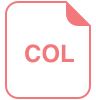









