tensorflow2.0分训练集,验证集和测试集交叉验证实现波士顿预测房价Python
时间: 2023-11-19 13:03:58 浏览: 105
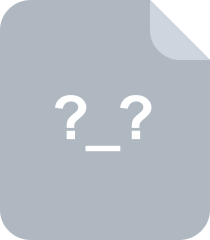
波士顿房价预测数据集,可以用来学习

好的,下面是实现波士顿预测房价的代码,包括训练集、验证集和测试集的交叉验证:
```python
import tensorflow as tf
from sklearn.datasets import load_boston
from sklearn.model_selection import train_test_split
from sklearn.preprocessing import StandardScaler
# 加载波士顿房价数据集
boston = load_boston()
# 数据预处理
ss = StandardScaler()
X = ss.fit_transform(boston.data)
y = boston.target
# 分割训练集、验证集和测试集
X_train, X_test, y_train, y_test = train_test_split(X, y, test_size=0.2, random_state=42)
X_train, X_val, y_train, y_val = train_test_split(X_train, y_train, test_size=0.25, random_state=42)
# 定义模型
model = tf.keras.Sequential([
tf.keras.layers.Dense(units=64, activation='relu', input_shape=(X_train.shape[1],)),
tf.keras.layers.Dense(units=32, activation='relu'),
tf.keras.layers.Dense(units=1)
])
# 编译模型
model.compile(optimizer=tf.keras.optimizers.Adam(),
loss=tf.keras.losses.MeanSquaredError(),
metrics=['mae'])
# 训练模型
history = model.fit(X_train, y_train, epochs=100, batch_size=32, validation_data=(X_val, y_val))
# 评估模型
test_loss, test_mae = model.evaluate(X_test, y_test)
print('Test Loss:', test_loss)
print('Test MAE:', test_mae)
```
代码中,我们首先加载波士顿房价数据集,并对数据进行标准化处理。然后使用`train_test_split`函数将数据集分割成训练集、验证集和测试集。其中,测试集占总样本的20%,训练集和验证集按照3:1的比例划分。接着,我们定义了一个包含3个全连接层的神经网络模型,并使用Adam优化器和均方误差损失函数进行编译。最后,我们使用`fit`函数训练模型,并在测试集上评估模型的性能。
阅读全文
相关推荐







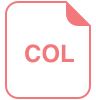
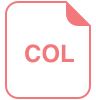
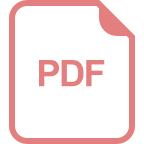
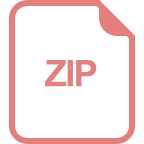
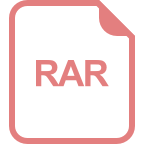
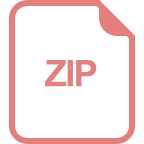