r语言logistic
时间: 2024-06-16 13:07:30 浏览: 112
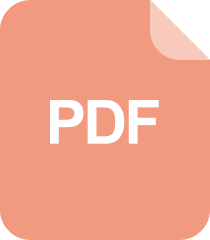
r language
R语言中的logistic回归是一种常用的统计分析方法,用于建立一个二分类模型。它通过将自变量与因变量之间的关系建模为一个S形曲线(logistic函数),来预测因变量的概率。
在R语言中,可以使用glm函数(generalized linear model)来进行logistic回归分析。下面是一个简单的示例代码:
```R
# 导入数据
data <- read.csv("data.csv")
# 构建logistic回归模型
model <- glm(y ~ x1 + x2, data = data, family = binomial)
# 查看模型结果
summary(model)
# 预测新数据
new_data <- data.frame(x1 = c(1, 2, 3), x2 = c(4, 5, 6))
predictions <- predict(model, newdata = new_data, type = "response")
```
在上述代码中,`data.csv`是包含自变量和因变量的数据文件。`y`是因变量,`x1`和`x2`是自变量。首先使用`read.csv`函数导入数据,然后使用`glm`函数构建logistic回归模型。`family = binomial`表示使用二项分布作为模型的假设。
通过`summary`函数可以查看模型的统计结果,包括系数估计、标准误差、z值和p值等。最后,可以使用`predict`函数对新数据进行预测,其中`type = "response"`表示输出的是概率值。
阅读全文
相关推荐
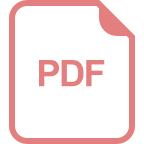
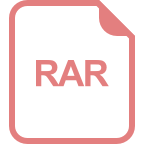















