dataframe随机拆分成两个dataframe
时间: 2023-08-08 14:02:17 浏览: 165
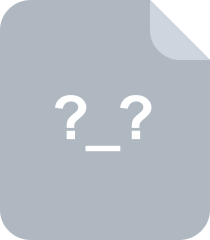
一份数据随机分成n等份

要将一个DataFrame随机拆分成两个DataFrame,可以使用pandas库中的sample()函数和drop()函数来实现。
首先,假设我们有一个名为df的DataFrame,它包含了我们想要拆分的数据。
接下来,我们可以使用sample()函数随机选择一部分数据样本。sample()函数可以接受一个参数n,表示选择的样本数量,以及一个可选参数random_state,用于设置随机种子,以保证每次运行结果的一致性。例如,我们可以使用以下代码选择df的50%的样本:
df1 = df.sample(frac=0.5, random_state=1)
这里,frac=0.5表示选择50%的样本,random_state=1表示使用随机种子1进行随机选择。
接下来,我们可以使用drop()函数从原始DataFrame中删除已选择的样本。例如,我们可以使用以下代码删除df中已选择的样本:
df2 = df.drop(df1.index)
这里,我们使用df1.index选择了df1中已选择的样本的索引,并使用drop()函数删除它们,得到了df2。
最后,我们可以通过打印df1和df2来查看结果:
print(df1)
print(df2)
这样,我们就成功将原始DataFrame随机拆分成了两个DataFrame。
阅读全文
相关推荐
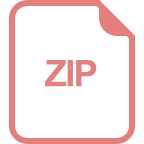
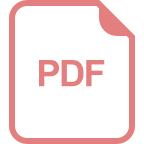
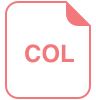
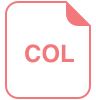
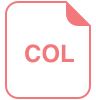
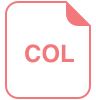
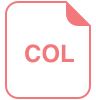
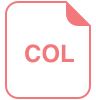
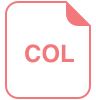
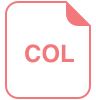
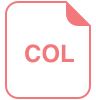
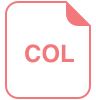
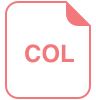
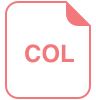
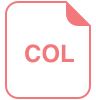


