# 生成混淆矩阵 cm = confusion_matrix(y_test, y_pred) # y_test为真实值,y_pred为预测值 print(cm)在此基础上绘制混淆矩阵
时间: 2024-03-07 15:49:33 浏览: 152
好的,你可以使用以下代码基于生成的混淆矩阵来绘制热力图混淆矩阵:
```python
import matplotlib.pyplot as plt
import seaborn as sns
from sklearn.metrics import confusion_matrix
# 生成混淆矩阵
cm = confusion_matrix(y_test, y_pred)
# 绘制热力图混淆矩阵
sns.heatmap(cm, annot=True, cmap='Blues', fmt='g')
# 设定图形属性
plt.title('Confusion Matrix')
plt.xlabel('Predicted Labels')
plt.ylabel('True Labels')
# 显示图形
plt.show()
```
此处,`y_test`是测试集的真实值,`y_pred`是模型对测试集的预测值。`confusion_matrix()`函数生成混淆矩阵,然后使用`sns.heatmap()`函数绘制热力图混淆矩阵,`annot=True`表示将数字显示在矩阵中,`cmap='Blues'`表示使用蓝色调色板,`fmt='g'`表示数字格式为普通数字。最后,使用`plt.title()`、`plt.xlabel()`和`plt.ylabel()`函数设定图形属性,使用`plt.show()`函数显示图形。
相关问题
# 生成混淆矩阵 cm = confusion_matrix(y_test, y_pred) # y_test为真实值,y_pred为预测值 print(cm) # 绘制热力图混淆矩阵 sns.heatmap(cm, annot=True, cmap='Blues', fmt='g') # 设定图形属性 plt.title('Confusion Matrix') plt.xlabel('Predicted Labels') plt.ylabel('True Labels')请在此基础上进行可视化
好的,您可以使用以下代码进行可视化,其中包括了对热力图的调整和对标签的旋转等操作:
```python
import matplotlib.pyplot as plt
import seaborn as sns
# 生成混淆矩阵
cm = confusion_matrix(y_test, y_pred)
# 绘制热力图混淆矩阵
sns.heatmap(cm, annot=True, cmap='Blues', fmt='g')
# 设定图形属性
plt.title('Confusion Matrix')
plt.xlabel('Predicted Labels')
plt.ylabel('True Labels')
# 调整热力图的左边和下边距离
bottom, top = plt.ylim()
plt.ylim(bottom + 0.5, top - 0.5)
plt.subplots_adjust(left=0.2, bottom=0.2)
# 旋转标签
plt.xticks(rotation=45)
plt.yticks(rotation=0)
plt.show()
```
这样,您就可以得到一个更加美观的混淆矩阵可视化图了。
解释cm = confusion_matrix(y_test, y_pred)
`confusion_matrix` 是一个用于计算分类模型预测结果的混淆矩阵的函数,它需要两个参数 `y_test` 和 `y_pred` 作为输入。其中,`y_test` 是测试数据集中的真实分类标签,`y_pred` 是分类模型对测试数据集的预测标签。该函数的输出结果是一个混淆矩阵 `cm`,它是一个二维数组,行表示真实分类标签,列表示预测分类标签。混淆矩阵中的每个元素表示预测为某个分类标签的样本在真实分类标签为该分类标签的情况下的数量。例如,`cm[i][j]` 表示真实分类标签为第 `i` 类,预测分类标签为第 `j` 类的样本数量。通过混淆矩阵可以更直观地了解分类模型的分类效果,进而可以对模型进行调整和优化。
阅读全文
相关推荐
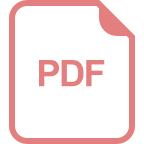
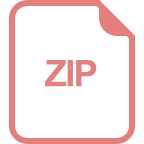
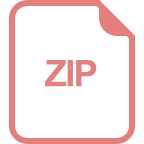













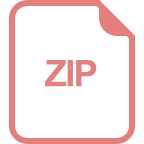