一维数据集 五分类混淆矩阵代码
时间: 2023-08-15 15:04:24 浏览: 105
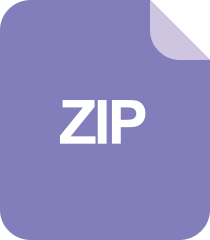
混淆矩阵
下面是一个计算五分类混淆矩阵的示例代码,假设你有一个一维数据集 `y_true` 表示真实标签,一个一维数据集 `y_pred` 表示预测标签:
```python
import numpy as np
from sklearn.metrics import confusion_matrix
# 定义标签类别
labels = [0, 1, 2, 3, 4]
# 计算混淆矩阵
cm = confusion_matrix(y_true, y_pred, labels=labels)
# 打印混淆矩阵
print(cm)
```
其中,`labels` 列表定义了标签类别,`y_true` 和 `y_pred` 分别表示真实标签和预测标签。调用 `confusion_matrix` 函数计算混淆矩阵,并将 `labels` 传入来指定标签类别。最后打印混淆矩阵即可。
阅读全文
相关推荐
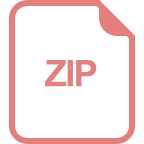

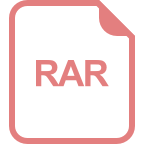
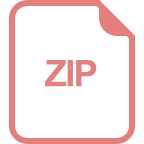
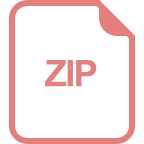
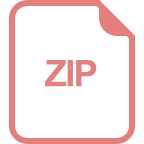
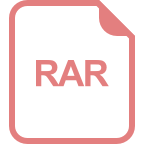
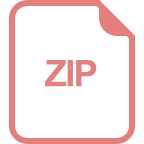
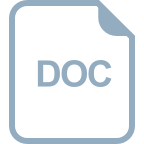
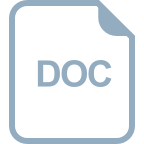






