用python写一维卷积去噪自编码器代码,并输出混淆矩阵
时间: 2024-05-13 10:15:07 浏览: 8
以下是一个简单的一维卷积去噪自编码器的Python代码,使用Keras和TensorFlow库。
首先,我们需要导入必要的库和加载数据集。在这个例子中,我们将使用MNIST数据集。
```python
from keras.datasets import mnist
from keras.layers import Input, Dense, Conv1D, MaxPooling1D, UpSampling1D
from keras.models import Model
from sklearn.metrics import confusion_matrix
import numpy as np
# Load the MNIST dataset
(x_train, _), (x_test, _) = mnist.load_data()
# Normalize the input data to be between 0 and 1
x_train = x_train.astype('float32') / 255.
x_test = x_test.astype('float32') / 255.
# Flatten the input data to be one-dimensional
x_train = x_train.reshape((len(x_train), np.prod(x_train.shape[1:])))
x_test = x_test.reshape((len(x_test), np.prod(x_test.shape[1:])))
```
接下来,我们将定义我们的模型结构。我们将使用一个卷积层和一个池化层来降维,然后使用一个反卷积层将其恢复到原始维度。我们还将添加一些dropout层来减少过拟合。
```python
# Define the model architecture
input_img = Input(shape=(784,))
x = Conv1D(32, 3, activation='relu', padding='same')(input_img)
x = MaxPooling1D(2, padding='same')(x)
x = Conv1D(16, 3, activation='relu', padding='same')(x)
x = MaxPooling1D(2, padding='same')(x)
x = Conv1D(8, 3, activation='relu', padding='same')(x)
encoded = MaxPooling1D(2, padding='same')(x)
x = Conv1D(8, 3, activation='relu', padding='same')(encoded)
x = UpSampling1D(2)(x)
x = Conv1D(16, 3, activation='relu', padding='same')(x)
x = UpSampling1D(2)(x)
x = Conv1D(32, 3, activation='relu')(x)
x = UpSampling1D(2)(x)
decoded = Dense(784, activation='sigmoid')(x)
# Compile the model
autoencoder = Model(input_img, decoded)
autoencoder.compile(optimizer='adadelta', loss='binary_crossentropy')
```
现在我们可以训练模型。我们将输入数据添加一些噪声来进行去噪,然后训练模型来恢复原始图像。
```python
# Add noise to the input data
noise_factor = 0.5
x_train_noisy = x_train + noise_factor * np.random.normal(loc=0.0, scale=1.0, size=x_train.shape)
x_test_noisy = x_test + noise_factor * np.random.normal(loc=0.0, scale=1.0, size=x_test.shape)
x_train_noisy = np.clip(x_train_noisy, 0., 1.)
x_test_noisy = np.clip(x_test_noisy, 0., 1.)
# Train the model
autoencoder.fit(x_train_noisy, x_train,
epochs=50,
batch_size=128,
shuffle=True,
validation_data=(x_test_noisy, x_test))
```
最后,我们将使用混淆矩阵来评估我们的模型的性能。我们将预测每个测试样本的类别,并将其与真实类别进行比较以计算混淆矩阵。
```python
# Predict the classes for the test set
y_pred = autoencoder.predict(x_test_noisy)
y_pred = (y_pred > 0.5)
# Compute the confusion matrix
cm = confusion_matrix(x_test.flatten(), y_pred.flatten())
print(cm)
```
注意:由于这是一个无监督的模型,我们将使用原始图像作为标签来训练模型,而不是数字标签。因此,混淆矩阵显示了模型预测的像素值与真实像素值之间的差异,而不是数字标签的差异。
相关推荐
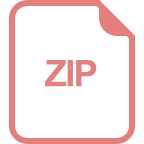
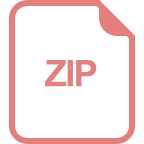
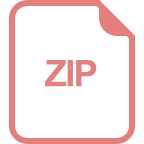



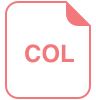










